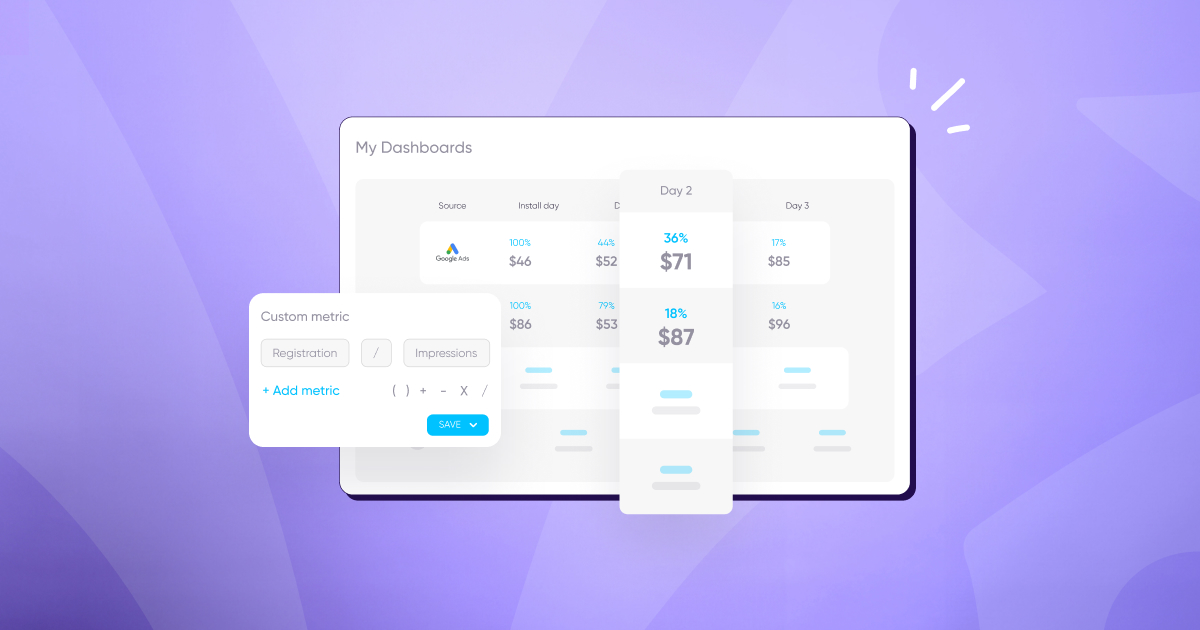
예측 분석으로 iOS 앱 성과 측정 마스터하기
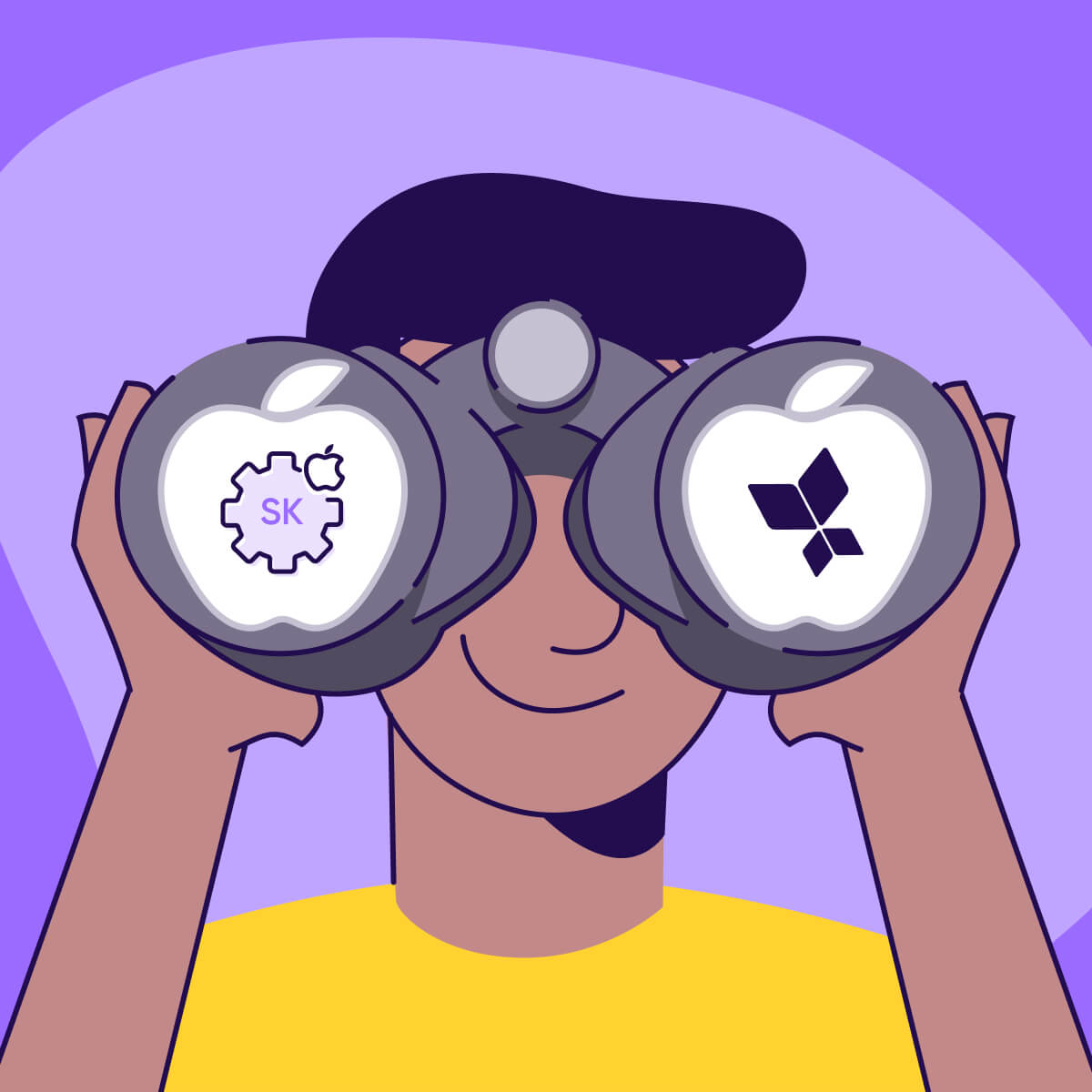
앱스플라이어는 2019년 말부터 오랜 시간 예측 분석 솔루션을 개발해왔습니다.
예측 분석 솔루션의 초기 목적은 UA 관리자에게 예측 데이터를 조기에 제공하여 최적화할 시간을 절약하도록 하는 것이었습니다.
그런데 2020년 6월, 애플이 iOS의 어트리뷰션 프레임워크, SKAdNetwork의 본격적인 출시를 발표하며 모바일 생태계에 새로운 개인정보보호 중심 시대가 열렸습니다. 이에 따라 전혀 보지 못했던 새로운 과제들이 생겼습니다.
그리고 Predict가 이러한 과제에 대한 해결책으로 떠올랐습니다. 제한 시간 내 정확히 측정하되, 개인정보를 보호하는 방식으로 빠르게 데이터를 분석하는 것, 그것이 디지털 마케팅에서 주 업무가 되었고 앱스플라이어가 중점을 둔 부분입니다.
앱스플라이어 Predict 최신 버전은 앱 설치 후 30일차 ROAS, ARPU, 잔존율 및 인앱 결제 유저율 예측치를 개인정보 노출 없이 집약된 형태로 제공합니다. 이렇게 UA에 유용한 데이터는 모두 SKAN 프로토콜과 원할히 통신하는 동안 생성됩니다.
그렇다면 다음 단계는 무엇일까요?
앱스플라이어는 트래픽 유형에 따라 정확한 성과 예측 데이터를 제공하기 시작했습니다.
iOS 트래픽 측정에서 가장 어려운 부분인 SKAN 측정을 이미 진행하고 있으며, 측정 방식은 매일 점점 더 최적화되고 있습니다.
이 지점에서 나아갈 다음 목표는 모든 iOS 트래픽 유형에 대한 예측 분석 데이터를 제공하는 것입니다.
모든 iOS 트래픽에 대한 예측 분석 데이터를 제공한다는 것이 실제로 무슨 뜻일까요?
모든 iOS 트래픽에 대한 예측 분석 데이터를 제공한다는 것이 실제로 무슨 뜻일까요?
iOS 14+ 시대에 iOS 트래픽은 여러 채널로 나눌 수 있습니다.
- 온드 미디어(owned media)
- 오가닉 트래픽
- Apple Search Ads
- 광고주 앱과 광고 게재 앱(퍼블리셔 앱)에서 모두 추적에 동의한 유저
- 앱스플라이어에서 측정된 앱 설치
- SKAN에서 측정된 앱 설치
위에 열거한 트래픽들은 다양한 측정 방법으로 측정되며, 모두 각각 관련 프라이버시 규정과 측정 제약 사항을 준수해야 합니다.
이러한 iOS 앱 트래픽 유형은 크게 두 가지 주요 어트리뷰션 방식으로 측정할 수 있습니다.
SKAN으로만 측정되는 iOS 트래픽
SKAN 포스트백만 사용하여 모바일 앱 캠페인 성과를 측정한 결과
MMP로만 측정되는 iOS 트래픽
ATT 추적에 동의한 유저(유저 레벨 데이터를 다른 회사와 공유하도록 허용한 사용자)에 대하여 ID 매칭 기법으로 어트리뷰션을 하거나 모든 유저에 대해 확률적 모델링 어트리션을 수행한 결과
각 어트리뷰션 방식에 대한 예측 데이터를 추출하려면 예측 솔루션을 각 방식의 요건과 제약사항에 맞춰야 했습니다. 엄청 복잡한 일이죠.
그러나 저희가 예측 솔루션을 개발할 때, 두 어트리뷰션 방식을 반영한 전체 그림을 제공하는 것이 필수였습니다. 광고주든 매체든 완전하고 종합적인 데이터 없이는 광고를 제대로 관리하고 최적화할 수 없기 때문입니다.
예측 솔루션 작동 방식
컨버전 스튜디오 Predict 모드
SKAN 연동 구조를 구축하고 실사용 테스트까지 성공적으로 완료하면, Predict를 앱스플라이어 컨버전 스튜디오 솔루션에 도입할 때 입니다.
앱스플라이어의 컨버전 스튜디오는 SKAN에서 전환 값 측정에 쓸 수 있는 6비트를 최대한 활용할 수 있도록 지원하는 유연한 솔루션 입니다. 광고주는 측정하고자 하는 유저의 인앱 활동과 앱 설치 이후 측정 기간을 직접 선택하여 측정 전환 값을 정밀하게 설정할 수 있습니다. 이렇게 컨버전 스튜디오에서 설정된 전환 값은 0~63으로 인코딩됩니다.
Predict는 SKAN 컨버전 스튜디오에서 수익, 전환, 인게이지먼트, 커스텀 모드와 같이 하나의 측정 모드로 추가됩니다.
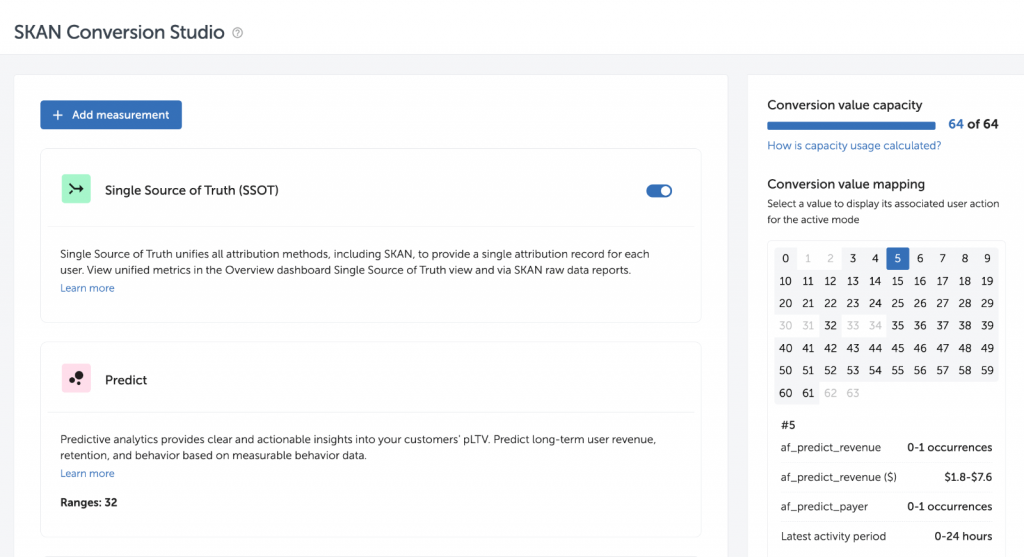
컨버전 스튜디오 내 Predict는 사용 가능한 6비트 중 5비트를 활용해 앱 설치 후 30일차 KPI에 대한 예측치를 추출합니다.
다른 컨버전 스튜디오 측정 모드는 앱 설치 후 24~72시간 내 측정된 실제 이벤트 정보를 제공하지만, Predict 모드는 첨단 머신 러닝 분석에 기반하여 그 시간 이후 발생할 가능성이 있는 이벤트에 대한 예측치를 제공합니다.
구독, 인앱 구매, 고급 레벨 달성 등 마케팅 퍼널 깊숙한 단계의 이벤트에 기반하여 최적화를 하는 광고주들에게는 가치있는 솔루션 입니다. 다운 퍼널 이벤트는 현 SKAN 구조에서 측정하기 어렵기 때문입니다.
컨버전 스튜디오에서 Predict를 사용할 때의 한 가지 장점은 예측 데이터와 함께 남은 6번째 비트로 SKAN의 유저 활동 측정 기간 내 발생하는 이벤트를 추가로 측정할 수 있다는 것입니다.
컨버전 스튜디오에 Predict가 추가됨으로써 앱스플라이어는 SKAN에 대한 준비를 마쳤습니다.
Predict 베타에 참여하는 광고주는 전환 값 스키마 매핑 상태를 모두 명확히 볼 수 있고 6번째 비트는 필요에 맞게 할당할 수 있습니다. 모든 작업이 한 대시보드에서 가능합니다.
앱스플라이어 어트리뷰션
앞서 말씀드렸듯이, 앱스플라이어 어트리뷰션은 추적에 동의한 유저들에 대해 ID 매칭을 하거나 모든 유저에 대해 확률적 모델링을 통해 수행됩니다.
앱스플라이어가 측정한 앱 설치에 대해 앱스플라이어의 예측 모델링을 적용하면 정확도가 더욱 높아집니다.
예측 데이터 인사이트는 엄격하게 작동되는 SKAN 모델과 개인정보보호를 위한 규제가 적용됨에 따라 여전히 24시간 내에 제공됩니다. 그러나 pARPU, pROAS, p잔존율과 같은 예측 KPI 값은 모두 예측치 자체로 사용할 수 있으며 SKAN에서 사용되는 값의 범위를 대체합니다.
6번째 비트 제한이 사라지면, 이러한 값들을 미리 정해진 범위에 맞출 필요가 없으며 각 예측 데이터 인사이트의 정확도가 향상될 수 있습니다.
또, 예측 데이터를 SKAN 전환 값으로 해석하고 SKAN 프로토콜과 통신할 필요도 없어서 프로세스 자체가 더 쉬워집니다.
익명으로 집계된 예측 KPI는 관련 매체와 함께 대시보드에 명확히 표시됩니다.
중복을 없앤 예측 데이터 단일 소스
SKAN과 MMP, 두 어트리뷰션 유형을 모두 사용하면 iOS 트래픽 전체를 측정할 수 있지만 데이터가 중복될 위험도 있습니다.
데이터 중복은 앱스플라이어와 SKAN이 동일한 앱 설치를 측정할 때 발생합니다.
앱스플라이어의 SSOT(Single Source of Truth)솔루션은 이러한 리스크를 정면으로 해결하고 광고주에게 정확한 iOS 어트리뷰션 데이터를 제공합니다.
측정된 모든 앱 설치는 중복 여부 확인을 거치고 동일한 앱 설치 건에 대해 SKAN과 앱스플라이어가 모두 기록한 경우, 앱스플라이어의 기록만 인정됩니다.
앱스플라이어 예측 데이터에서도 중복 데이터 제거 로직이 적용된다는 점을 기억해 두시기 바랍니다. 예측 데이터에는 앱 설치 건 하나하나가 모두 반영되기 때문에, 동일 앱 설치 데이터가 중복해서 들어가면 특정 유저 집단(코호트)에 대한 KPI 예측치가 변경될 수 있습니다.
앱스플라이어의 예측 데이터에서 고려해야 할 또다른 중요한 점은 오가닉 인스톨은 SKAN 측정 범위 밖이라는 점 입니다. 따라서 오가닉 유저 집단의 행동을 정확히 예측하려면 SSOT을 반드시 실행시켜야 합니다.
오가닉 유저는 앱스플라이어와 SKAN 모두 측정하지 않은 유저 집단으로, iOS 성과 측정에서 중요한 부분입니다.
SKAN 전환 값 측정을 위한 6개 비트 중 예측에 사용되는 비트는 5개이므로, 6번째 비트는 앱스플라이어로 측정되는 유저와 SKAN으로 측정되는 유저를 구분하는데 사용할 것을 권장드립니다. (한 플랫폼으로 모든 광고 성과를 측정할 수 있도록 이 방법을 추천드립니다.)
iOS 앱 성과 예측 마스터하기
Predict는 새로운 iOS 환경에 맞춰 개발된 다양한 앱스플라이어 솔루션들을 통합하여 각 어트리뷰션 모델에 따른 예측 데이터를 제공합니다.
앱스플라이어 대시보드에서 토글을 간단히 조작하여 예측 데이터, SKAN 데이터, 데이터 중복을 제거한 SSOT를 쉽게 조회할 수 있습니다.

Predict가 어떤 식으로 발전하든 기본적으로 그 가치는 정확한 예측 데이터를 제공하는데 있습니다. 유일한 변화는 예측 데이터를 제공하는 어트리뷰션 방식입니다.
마케터는 이제 iOS 전역에서 ARPU, ROAS, 잔존율, 결제 유저율 같은 주요 KPI를 예측하여 최적화를 더욱 정확하고 빠르게 할 수 있습니다.
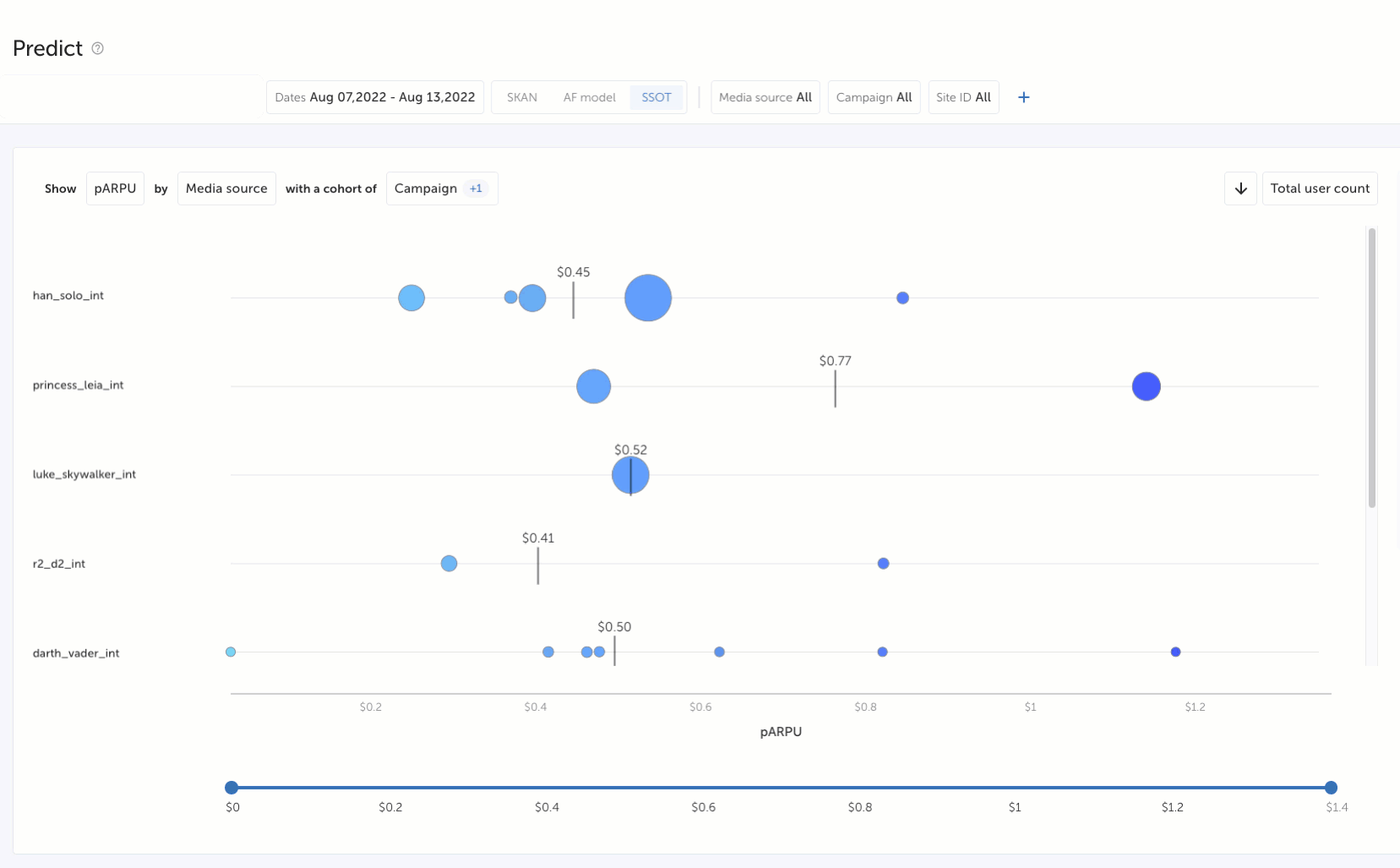
성적이 저조한 캠페인을 정확한 LTV(유저생애가치) 결과를 기다리기 위해 귀한 마케팅 예산을 낭비하면서 보름에서 한 달이나 집행한 후에야 멈출 필요는 없습니다.
효과가 좋은 캠페인은 조기에 활용하여 집행 시작일부터 투자 효율성을 더욱 높일 수 있습니다.
이제 iOS 앱 성과 예측을 할 수 있게 되었으니, 앱스플라이어는 다음 영역으로 진출합니다. 바로 안드로이드 예측 기술입니다.
다음 소식을 기대하세요!