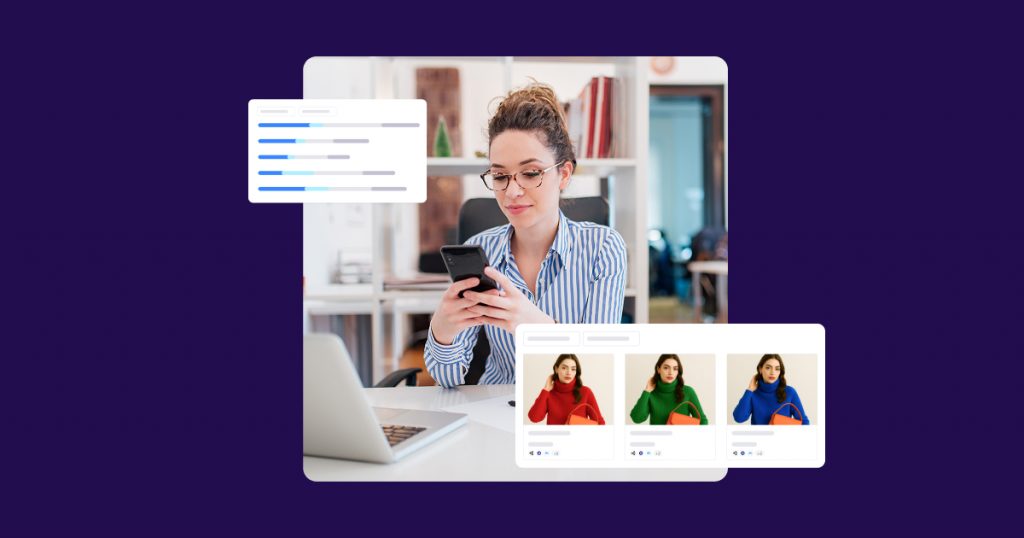
Вступление
По мере развития цифровой экосистемы, технология SaaS стала критически важной составляющей эффективных маркетинговых операций.
Маркетинговые и рекламные технологии подразделяются на множество сложных и пересекающихся категорий, где буквально тысячи партнеров соревнуются за ваши маркетинговые бюджеты.
Неважно, с какой целью вы строите свой маркетинговый технический стек, основной вопрос заключается в том, с чего начать. И хотя добавление или изменение маркетинговых технологий требует значительных вложений в анализ, тренинг, развитие и другие ресурсы, ключом к успеху является адаптивность.
Согласно опросу Ascend2 за март 2019 года, 67% маркетологов добавляют новые типы MarTech в стек каждый месяц или раз в квартал.
Существуют разные причины создания Martech стека: от настраивания рекламных кампаний и их автоматизации, до измерения и обогащения данных.
- Маркетинговые технологии могут значительно повысить эффективность, прозрачность, гибкость и ценность всех маркетинговых операций.
- Хотя некоторые технологии можно создать своими силами, это процесс довольно энергозатратный и недостаточно эффективный. Среди продуктов, которые невозможно смоделировать самостоятельно, можно назвать мобильную атрибуцию.
- Конкуренция в сфере маркетинговых технологий чрезвычайно высокаь. Существование лидеров рынка, которым принадлежит 80% доли рынка в данной категории, не мешает постоянному притоку новых игроков, что стимулирует стремительные инновации и ценовую конкуренцию.
Хотя стандартной формулы успеха не существует, цель этого руководства – дать основу для построения надежного стека маркетинговых технологий с акцентом на мобайл.
В этом руководстве мы рассмотрим следующие темы:
- Основы маркетингового стека: определение стратегических целей и инструментов стека, которые следует учитывать на протяжении всего жизненного цикла продукта
- Глубокое погружение в категории: аналитика продуктов, автоматизация маркетинга, мобильная атрибуция и платформы клиентских данных (CDP)
- Дополнительные соображения: критерии оценки и отраслевые тенденции (например, структура стекового проектирования, анализ затрат/прибыли, сравнение между самостоятельным построением технологий и их покупкой, выбор лучших инструментов и т. д.)
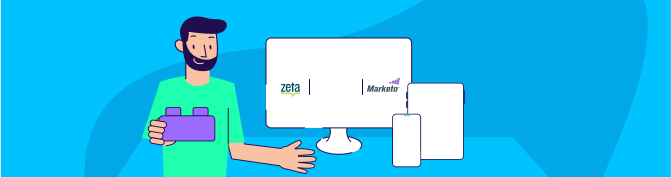
Глава 1
Основы стека технологий мобильного маркетинга
Зачем вам нужен стек маркетинговых технологий?
Большинство современных маркетологов активно используют технологический стек, но если вы еще не знаете, что это такое, давайте начнем с самого начала.
Стек маркетинговых технологий – это набор технологий, которые маркетологи используют для проведения и оптимизации своей маркетинговой деятельности.
Эти инструменты «MarTech» поддерживают, упрощают и унифицируют сбор, организацию и использование данных об активности пользователей по платным и собственным каналам.
Вот пример хорошо продуманного технического стека, любезно предоставленного Amplitude:
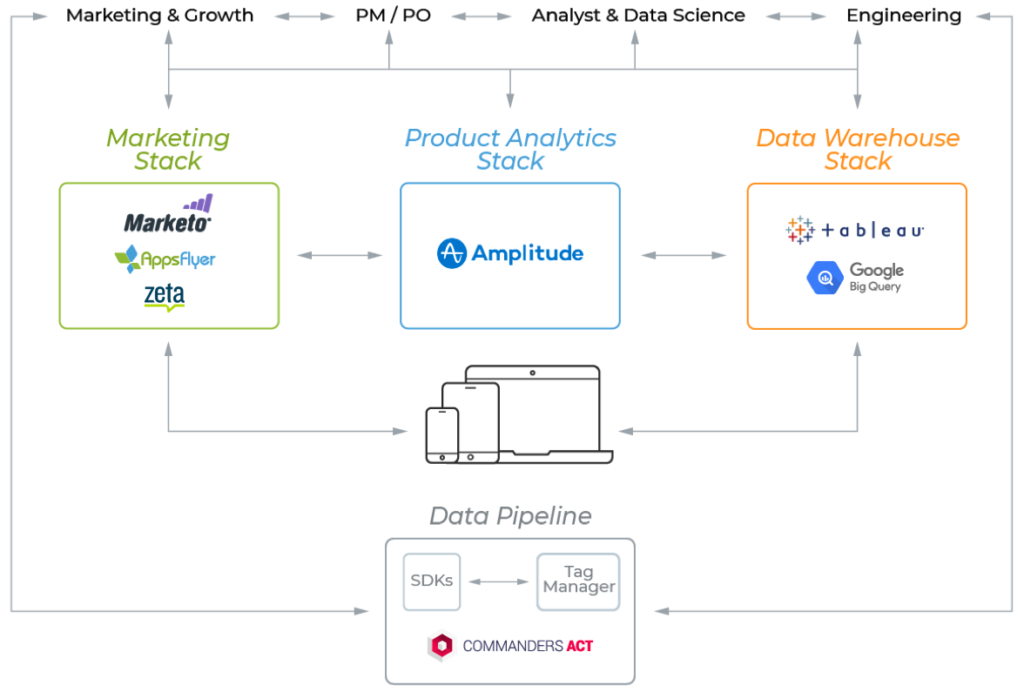
Существуют разные мнения относительно того, насколько необходимо наличие той или иной технологии в своем стеке. Существуют разные варианты: от разработки собственных решений до покупки продвинутых технологий. Однако вне зависимости от выбранного варианта, основные преимущества обычно сводятся к эффективности и масштабируемости.
Вот несколько примеров этих преимуществ:
- Объединение разрозненных систем (например, хранение данных, интеграция партнеров, обогащение данных для кросс-канальной аналитики, создание аудиторий, постбэков и т. д.)
- Активация и управление кампанией (например, сегментация аудитории и обмена сообщениями, развертывание ссылок/креативов, A/ B-тестирование, электронная почта, push-уведомления и т. д.)
- Расширенные возможности анализа и повышения ROI (например, визуализация данных, когортный анализ, мобильная атрибуция и т. д.)
- Оптимизация пути пользователя (например, A/B-тестирование, диплинки , баннеры и т. д.)
Действительно, эти преимущества четко отражены в исследовании Ascend2 за март 2019 года , в котором эффективность стала основной причиной для использования маркетинговых технологий:
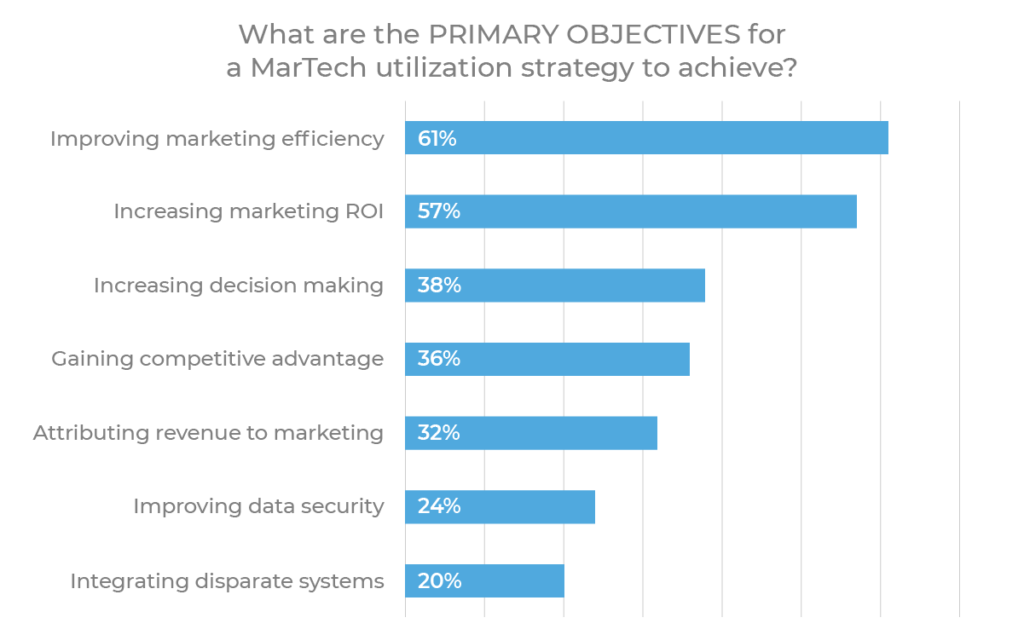
Тот же опрос также показал, что 63% маркетологов планируют умеренно увеличить свой общий бюджет на мартех, а 24% планируют увеличить его значительно.
Во многих случаях выгода от покупки более продвинутых решений перевешивает затраты.
Основы: как начать создавать стек технологий мобильного маркетинга
Первым шагом к построению вашего стека технологий является понимание цели.
Ответьте себе на следующие вопросы:
- Каковы ваши цели роста (удовлетворить нужды, связанные с увеличением объема данных или решить проблему масштабируемости)?
- Как выглядит путь вашего пользователя?
- Каковы основные KPI на каждом этапе пути?
- Какие дополнительные события было бы полезно измерить для того, чтобы оптимизировать весь путь пользователя?
- Какие каналы и функции вам понадобятся для привлечения и удержания пользователей?
- С какими медиа-партнерами вы работаете (или планируете работать)?
- Какая инфраструктура вам понадобится для хранения данных и управления ими?
- Кому принадлежат ваши данные, и какие протоколы безопасности вы должны учитывать, чтобы обеспечить безопасность ваших данных?
- Как вы видите свой маркетинг в будущем?
- Обязательно ли вам иметь полное представление о маркетинге/деятельности продукта по каналам и платформам?
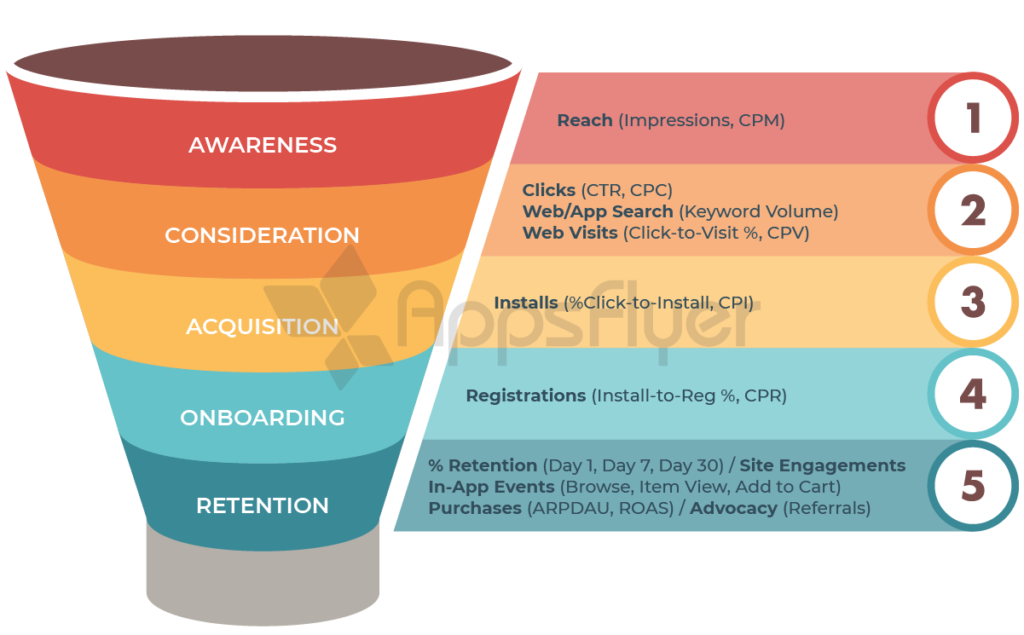
Ответы на эти вопросы позволят вам не только разделить инструменты на категории «обязательные» и «полезные», но и помогут в оценке партнера по таким критериям, как функционал, стоимость, интеграция, поддержка и масштабируемость.
Выбор инструментов вашего стека на начальном этапе будет зависеть от бюджета, однако в долгосрочной перспективе лучше создавать стек исходя из масштабируемости.
При составлении маркетингового стека нужно подумать не только о своих целях, приоритетах и бюджетах, но и о возможных трудностях
Платформа клиентских данных Segment недавно опубликовала исследование данных, которое иллюстрирует различные варианты реализации этой стратегии. Например, некоторые компании начинают с небольшого набора технологий и постепенно заменяют их на новые и более продвинутые. По мере того как появляются более продвинутые инструменты, такие компании переводят свои бизнесы на новые платформы.
На следующем рисунке показан пример этой стратегии.
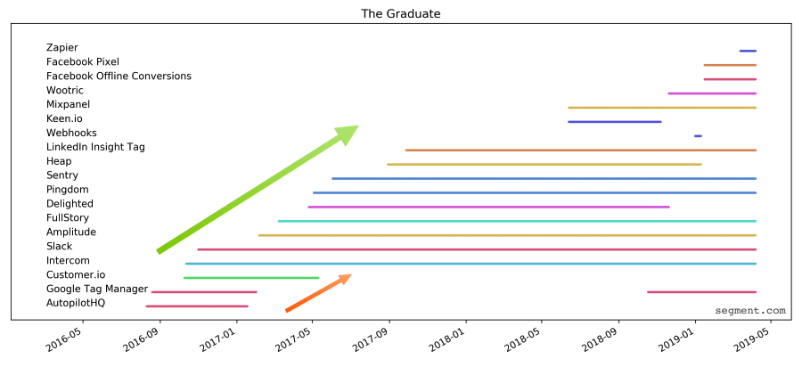
Вот еще один пример компании, которая в течение 1,5 лет неуклонно расширяла свои инструменты в области аналитики, обмена сообщениями, рекламы и атрибуции:
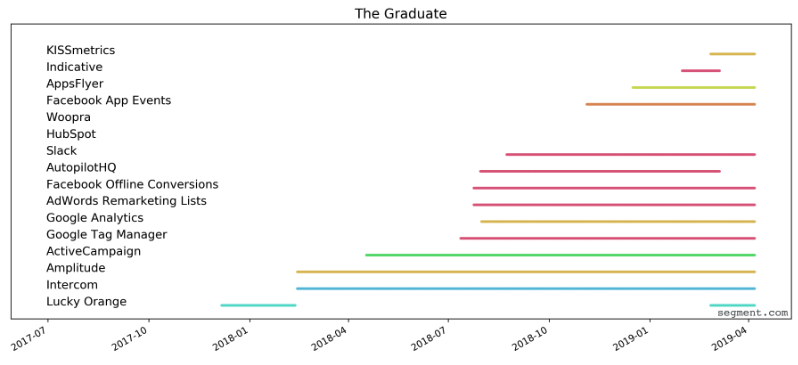
Этот подход может быть полезен для компаний на начальном этапе становления, когда идет постепенное накапливание средств и знаний для добавления новых инструментов. Однако этот подход требует продуманного планирования, чтобы избежать чересчур мудреного набора инструментов, случайно объединенных в стек. Единый и четкий стек требует более серьезного и продуманного подхода.
Segment представляет другой пример подхода под названием «Массовая покупка», при котором компании покупают сразу много инструментов, но не начинают использовать их все одновременно, а запускают группами, состоящими из 3 -5 инструментов.
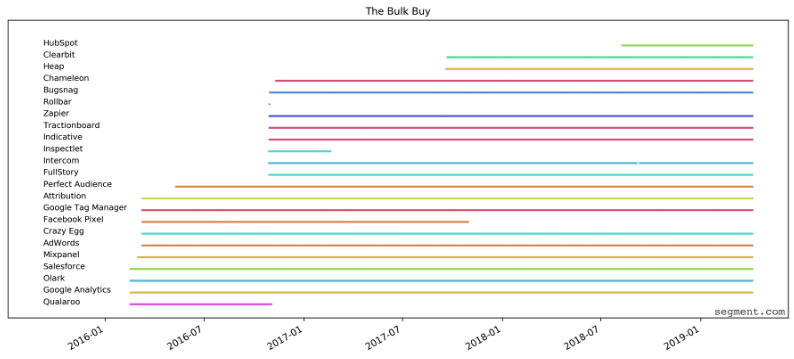
Какая стратегия больше всего подходит для вашей компании?
Оценивая ваших партнеров по маркетинговым инструментам, исходите из соотношения “цена-качество”.
Вещи, которые также следует учитывать:
- С чего начать? Базовое/бесплатное или платное / расширенное решение?
- Покупка или создание собственного решения?
- Старые добрые инструменты по типу «все в одном флаконе» или супер-современные технологии?
Мы углубимся в каждую из этих тем в следующих главах, но сначала давайте начнем с определения основных компонентов стека технологий мобильного маркетинга.
Основы: Иерархия потребностей мобильного стека технологий
Само собой разумеется, что будучи компанией, занимающейся атрибуцией, мы считаем, что атрибуция является ключевым элементом эффективного маркетинга . Хотя атрибуция может быть не первым, о чем вы думаете, когда создаете и запускаете свое первое приложение с ограниченным бюджетом, в реальности инструменты атрибуции и диплинков критически важны для любой маркетинговой команды, стремящейся к росту.
Рост – это основная задача как для развивающихся стартапов, так и для компаний из списка Fortune 500, которые расширяют свое цифровое присутствие. В конечном итоге рост заключается в способности отслеживать источники вашего трафика на десктопе и в мобайле.
Тем не менее есть много факторов, которые влияют на решение о том, является ли мобильная атрибуция обязательной в вашем маркетинговом стеке или желательной.
Эти факторы могут включать размер компании, технические навыки маркетинговой команды, бюджет, эксплутационная совместимость устаревших технологий и личные предпочтения.
На следующем рисунке показана типичная иерархия потребностей мобильного маркетолога на протяжении жизненного цикла продукта и роль каждого решения в пользовательской воронке.
Порядок потребностей в жизненном цикле продукта не обязательно отражает логический порядок последовательной интеграции.
Например, инструменты бизнес-аналитики, такие как CDP, инструменты визуализации данных и облачного хранения, можно рассматривать как фундамент целостного технологического стека.
Возможно, в самом начале пути у вас не будет необходимости во всех этих инструментах, однако, если вы рассчитываете на масштабирование, стоит подумать об интеграции этих решений на каком-то этапе.
Взгляните на оба вида графика:
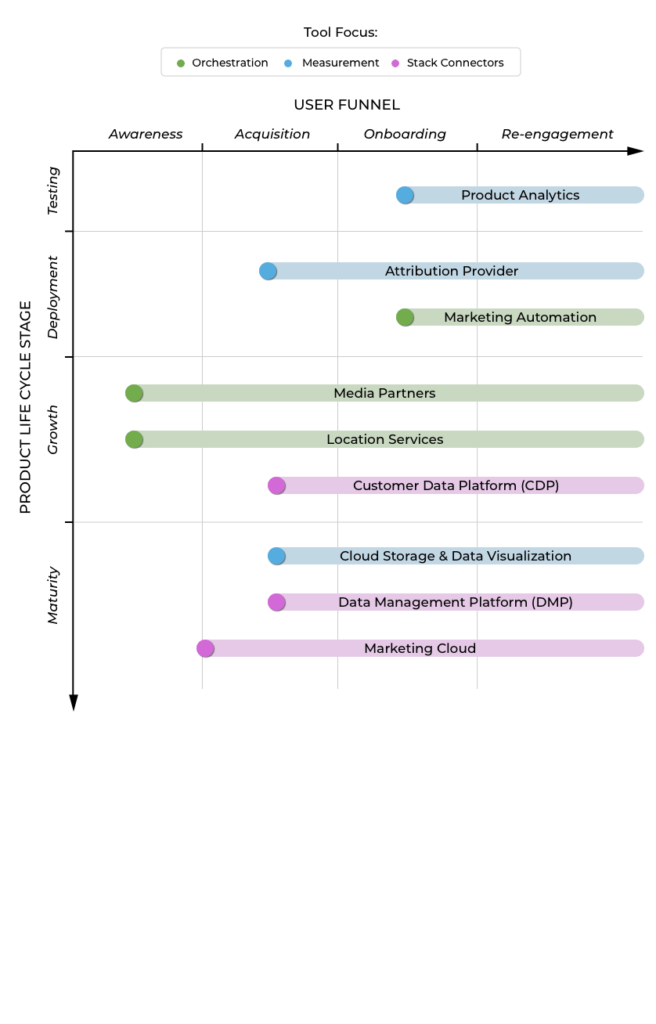
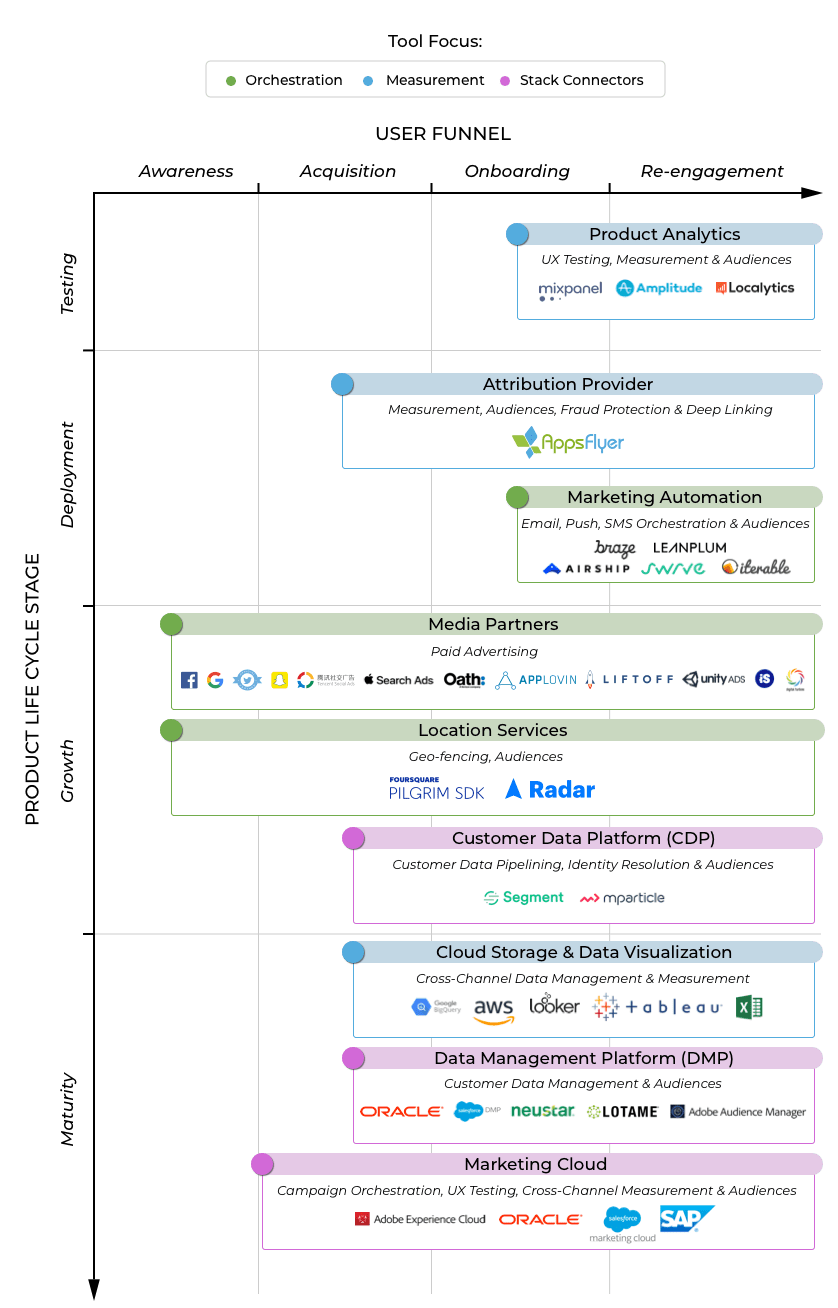
Давайте поговорим об отдельных компонентах иерархии:
- Продуктовая аналитика:
Ориентируясь на опыт работы с мобильными приложениями, компании, занимающиеся продуктовой аналитикой, специализируются на тестировании UX и созданием персоны пользователей. Благодаря поведенческой и прогностической аналитике они обеспечивают простую структуру для оптимизации продукта, а также расширенные отчеты о показателях удержания, воронках и когортном анализе.
Очевидно, что для новых приложений крайне важно иметь план измерений для тестирования UX перед запуском. Тем не менее мы обычно видим, что стартапы начинают с базовых бесплатных инструментов, таких как Firebase и/или с аналитики приложений, предоставляемой поставщиками мобильной атрибуции и автоматизации маркетинга.
Точно так же интернет-компании, которые открывают свои первые приложения, на начальном этапе часто используют инструменты веб-аналитики. - Поставщик атрибуции:
Основная функция партнеров, таких как AppsFlyer – это мобильная атрибуция, те есть процесс измерения, позволяющий соотнести каждую установку и любое внутреннее событие с маркетинговой кампанией и медиа-источником. Хотя некоторые считают атрибуцию «необязательной технологией» до тех пор, пока не начнется привлечение платных медиа, важно помнить, что большинство поставщиков автоматизации маркетинга не предоставляют инструментов для диплинкинга.
Например, OneLink от AppsFlyer позволяет маркетологам создавать единую ссылку, которая может автоматически определять платформу каждого пользователя и состояние приложения (установлено или нет), чтобы отправлять их на оптимальную страницу вебсайта или приложения. Диплинки имеют решающее значение не только для платных, но и для собственных медиа, таких как электронная почта, веб-страницы и SMS.
В дополнение к мобильной атрибуции и глубоким ссылкам поставщики атрибуции также обеспечивают сегментацию аудитории, защиту от фрода, расширенную аналитику и, в зависимости от поставщика, атрибуцию People-Based для получения целостной картины о всех точках взаимодействия пользователей с рекламой на разных каналах. - Автоматизация маркетинга:
Партнеры по автоматизации маркетинга, такие как Braze и Leanplum, способствуют повторному вовлечению существующих пользователей через каналы CRM и основной продукт. Вовлечение пользователей можно сочетать с сегментацией аудитории и A/B-тестированием с помощью push-сообщений, имейл маркетинга, обмена сообщениями в приложении или SMS. - Медиа-партнеры:
Медиа-партнеры, такие как Google, Facebook и Amazon, предоставляют рекламные ресурсы в мобильных приложениях, интернете и на TV, чтобы повысить осведомленность, привлечь новых пользователей и повторно привлечь существующих.
Медиа-партнеры используются для показа рекламы через различные каналы и форматы, включая социальные сети, дисплейную рекламу, видео и другие. Вы можете узнать больше о подкатегориях медиа-партнеров в нашем руководстве «Как начать работать с мобильной атрибуцией». - Службы геолокации:
В своем маркетинговом сценарии использования Burger King продемонстрировали эффект объединения данных геоположения с автоматизацией маркетинга и привлечением пользователей.
Службы геолокации дают возможность отслеживать пользователей на разных геозонах. Это означает возможность устанавливать геозоны динамически и обеспечивать поступление маркетинговых данных в ваш CDP, инструменты атрибуции и автоматизации маркетинга. - Платформа клиентских данных (CDP):
Новый игрок в мире маркетинговых технологий. Такие CDP, как mParticle и Segment, собирают, унифицируют, сегментируют и активируют пользовательские данные из различных SDK.
CDP автоматизируют и обогащают распределение сегментов данных о клиентах между всеми другими системами в техническом стеке рекламодателя в режиме реального времени. Это не только упрощает установку новых SDK, но также поддерживает согласованность агрегированных отчетов по сырым данным, загружаемых из хранилищ данных и продуктов визуализации. - Облачное хранилище:
Поставщики облачных хранилищ поддерживают, управляют и удаленно создают резервные копии необработанных данных во всех ваших системах технического стека. Такие поставщики, как AWS, Microsoft Azure, Google Cloud Storage и Snowflake, предоставляют безопасный и масштабируемый способ хранения и доступа к огромным объемам необработанных данных в вашей организации. - Визуализация данных:
Простейшая визуализация данных обычно выполняется с помощью электронных таблиц (т.е. CSV, Excel, Google Sheets), но этот формат может стать слишком трудоемким или немасштабируемым по мере роста вашего бизнеса. По этой причине более продвинутые команды часто работают с такими продуктами, как Looker и Tableau, для создания онлайн дэшбордов, статистических моделей и автоматических отчетов. - Платформа управления данными (DMP):
DMP используются для сбора данных об аудитории на разных платформах, устройствах и каналах. В отличие от MMP, DMP собирают не только собственные, но и сторонние данные для отображения демографических, психографических и фирмографических сегментов.
Большинство медиа-партнеров могут получить для вас доступ к сторонним данным DMP (взимая дополнительную плату за таргетинг), но некоторые рекламодатели предпочитают работать со своими собственными DMP для простоты управления собственной/сторонней аудиторией. - Маркетинговое облако:
Маркетинговые облачные компании предлагают услуги по всему спектру инструментов MarTech, AdTech и BI (несмотря на их попадание в категорию автоматизации маркетинга выше). Маркетинговые облака, такие как Adobe, Oracle и Salesforce, обеспечивают управление данными клиентов, а также инструменты аудитории, регулирование кампаний и аналитику по всем каналам, включая мобайл, интернет, ТВ и офлайн CRM.
Некоторые поставщики облачных услуг также предлагают дополнительные продукты, такие как CDP, платформы управления данными (DMP) и автоматизированные системы покупки рекламы (DSP).
Теперь, когда мы прошлись по основным определениям, давайте глубже погрузимся в конкретные функции и варианты использования четырех основных инструментов мобильного маркетинга: атрибуции, автоматизации маркетинга, аналитики продуктов и CDP.
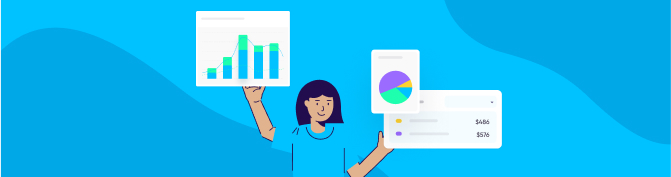
Глава 2
Глубокое погружение в мобильную атрибуцию
Помимо платных кампаний, собственных каналов и оптимизации магазина приложений, привлечение пользователей (UA) является фундаментальной частью вашего мобильного бизнеса, учитывая то, что:
- Оно способствует развитию гиперконкурентной экосистемы “free-to-install” приложений.
- Органическое обнаружение приложений во многих случаях проблематично.
- Привлечение пользователей – ящик Пандоры, где эффективная практика влечет за собой более высокий рейтинг в магазине приложение, что, в свою очередь, приводит к еще большему притоку пользователей.
OnОдним из самых мощных инструментов преобразования сложных данных в ценные инсайты для UA является поставщиком атрибуции, также известный как партнер по мобильным измерениям (MMP) в мире приложений.
Хотя возможности разных провайдеров несколько различаются, MMP предоставляет множество инструментов, включая диплинки, защиту от мошенничества, сегментацию аудитории и, конечно же, атрибуцию.
Мобильная атрибуция – это процесс измерения, позволяющий соотнести каждую установку и любое внутреннее событие с маркетинговой кампанией и медиа-источником.
WБез атрибуции маркетологам не хватает наглядного представления эффективности, что приводит к значительному перерасходу средств. Для того чтобы заплатить медиа-партнерам за привлеченных пользователей, маркетологам необходимо работать с партнером, имеющим целостное видение картины.
Встраивание мобильной атрибуции в ваш стек MarTech
Сейчас уже ни у кого не возникает сомнений относительно роли поставщика атрибуции в успехе мобильного приложения. Тем не менее существует заблуждение, что для рекламы на собственных медиа поставщик атрибуции не нужен.
Вот две основных причины интеграции мобильной атрибуции на ранних этапах жизненного цикла продукта еще до того, как вы прибегнете к платным медиа:
- Атрибуция основана на понимании взаимодействия как с платными, так и с собственными медиа на протяжении всего пути пользователя, от показа рекламы до push-уведомлений и имейлов.
Атрибуция имеет решающее значение для оптимизации внутренних событий приложения, таких как подписки, покупки и завершение уровней, в дополнение к таким метрикам, как удержание, доход и пожизненная ценность (LTV). - Используя информацию, собранную поставщиком атрибуции, диплинки позволяют маркетологам создавать единую ссылку, которая может автоматически определять устройство пользователя, канал, платформу и состояние приложения (установлено или нет), чтобы отправить их на оптимальную страницу в Интернете или приложении.
Будучи безусловно полезными для платных кампаний, диплинки абсолютно незаменимы для оптимизации опыта пользователей с собственными медиа.
Если вы уже установили приложение, тут еще более проблематично, потому что вам нужно закрыть веб-страницу, открыть приложение и вручную найти интересующий вас экран приложения.
Именно поэтому Universal Links так важны.
Если маркетологи не прибегают к услугам поставщика диплинков, у них нет возможности оптимизировать опыт пользователя с учетом устройства и платформы.
И хотя некоторые поставщики услуг электронной почты (ESP) и платформы автоматизации маркетинга разработали собственные решения для Universal Links, немногие из них способны справиться с любым сценарием.
Onelink от AppsFlyer безупречно работает на платных каналах, в SMS кампаниях, при приглашениях пользователей, на баннерах “web-to-app”, реферальных программах, QR кодах и т.д.
Обратите внимание, что без данных атрибуции о том, где, когда и как пользователи взаимодействуют с рекламой и приложениями, диплинки не могут быть полностью оптимизированы; эти две функции дополняют друг друга.
That is, deep linking is the process of using attribution data to route the user appropriately. Since deep links are powerful tools То есть диплинки – это процесс использования данных атрибуции для правильной маршрутизации пользователя.
Поскольку диплинки являются мощными инструментами на любом этапе развития вашего приложения, крайне важно найти поставщика атрибуции, который объединит диплинки и атрибуцию на едином дэшборде.
Теперь, когда мы рассмотрели две основные причины для интеграции атрибуции на ранних этапах жизненного цикла продукта, давайте углубимся в более сложные функции и варианты использования.
Не знаете, как атрибуция вписывается в ваш текущий стек технологий? Свяжитесь с нами.
Основные варианты использования атрибуции
WСуществует множество случаев применения данных атрибуции, но давайте рассмотрим пять наиболее универсальных и широко распространенных вариантов:
1. Объединение данных по платным и собственным медиа-каналам.
Во многих смыслах поставщика атрибуции можно рассматривать как связующее звено между рекламными партнерами и партнерам по маркетинговым технологиям.
Если маркетологи смогут определить принадлежность in-app событий к платным кампаниям повторного вовлечения или к кампаниям маркетинговой автоматизации (push, email и т.д.), они смогут лучше синхронизировать усилия и увеличить ROI.
Благодаря встроенной партнерской интеграции, MMP предоставляют целостные данные об атрибуции для аналитики продуктов, автоматизации маркетинга, инструментов разработчика и других партнеров по стеку.
MMP также избавляют маркетолога от необходимости интегрировать новые SDK для каждого нового рекламного партнера, оптимизируя процесс тестирования и обеспечивая непрерывный цикл обратной связи за счет использования постбэков.
2. Оптимизация кампаний с помощью расширенной атрибуции и аналитики.
Эффективная оптимизация кампании требует детализированных измерений.
Например, приложение для путешествий может измерять основные события, такие как установки, открытие приложения, регистрации, добавление в корзину и бронирование в качестве ключевых показателей эффективности (KPI).
Однако, сопоставляя дополнительные параметры, такие как страна вылета, пункт назначения и класс, маркетолог может получить больше возможности для оптимизации, а также для сегментации аудитории.
Кроме того, анализируя корреляцию конкретных событий в приложении, маркетологи могут создавать прогностические модели, чтобы лучше оптимизировать рентабельность инвестиций и, в конечном итоге, LTV.
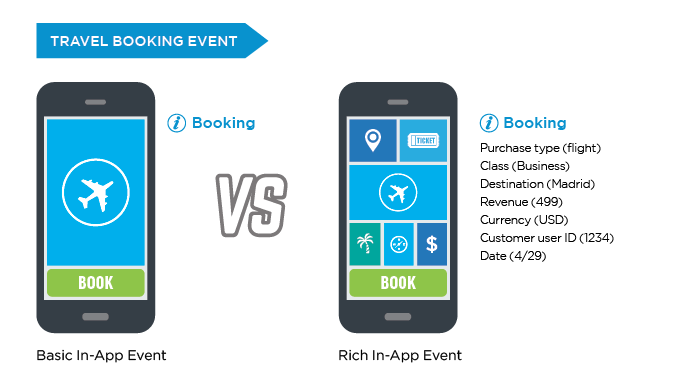
Помимо обеспечения детального измерения внутренних событий приложения, поставщики атрибуции также могут связывать точки между различными каналами и устройствами, чтобы обеспечить целостное представление пути пользователей в рамках единого дэшборда.
Например, AppsFlyer предлагает мультитач атрибуцию, чтобы показать, с каким медиа-источником пользователь взаимодействовал непосредственно перед установкой, и какие сети оказались “вспомогательными” на этом пути.
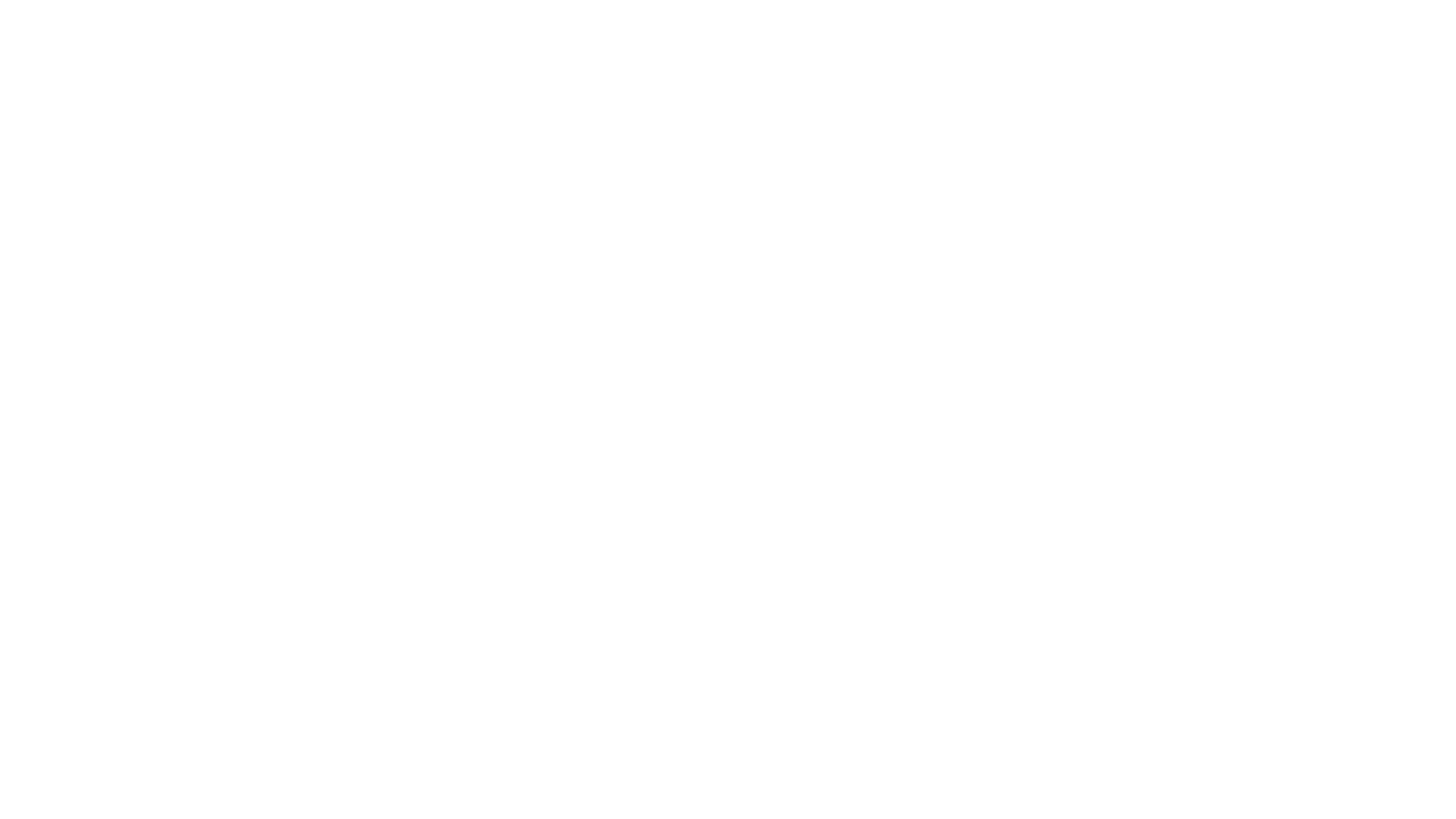
Благодаря таким четким данным у маркетологов больше ресурсов для борьбы с фродом, для правильного распределения рекламных расходов и для предотвращения двойной, а порой и тройной переплаты рекламным сетям
3. Обнаружение и предотвращение мошенничества с рекламой в режиме реального времени.
Согласно отчету AppsFlyer Состояние мобильного фрода [H1 2019], мошенничество с мобильной рекламой представляет собой растущую отраслевую проблему: 22,6% установок неорганических приложений во всем мире являются мошенническими.
Мошенничество – это игра в кошки-мышки, в которой мошенники постоянно находят способы избежать обнаружения. Учитывая динамический характер мошенничества, лучший способ предотвратить изощренные тактики мошенничества – это использовать масштабные данные MMP для обнаружения и блокировки фрода.
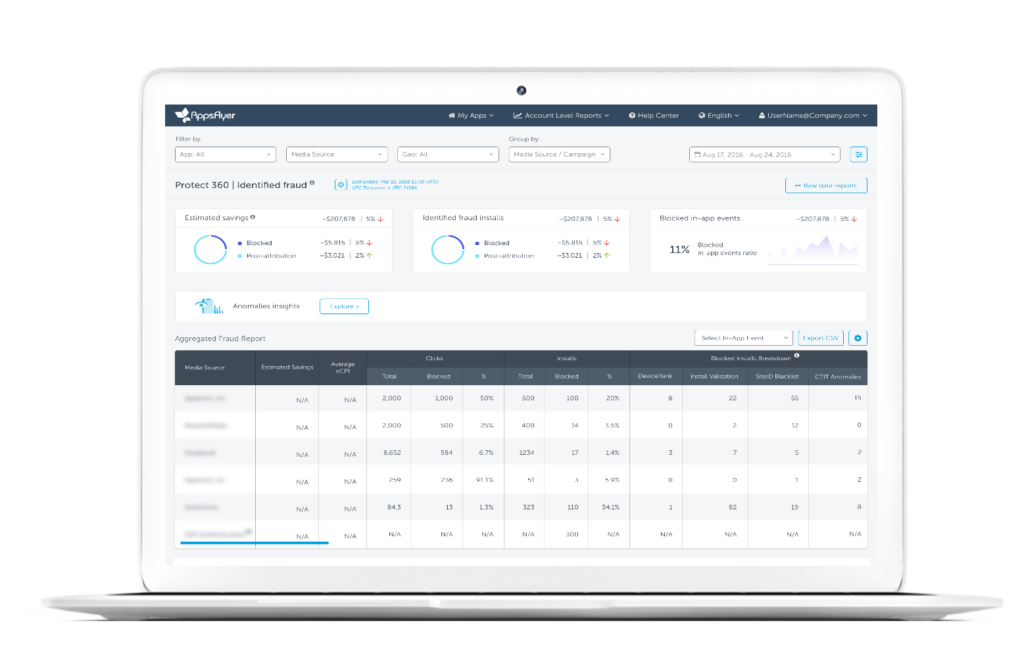
Использование передового решения, такого как Protect360 от AppsFlyer, гарантирует защиту от мошенничества в реальном времени, а также предоставляет набор расширенных отчетов, которые можно использовать для настройки кастомизированных правил и оповещений.
Понимая, что 16% случаев фрода, связанного с установкой приложений, невозможно обнаружить в режиме реального времени, Protect 360 также является первым отраслевым решением, которое выявляет поведенческие аномалии после установки.
4. Определите и увеличьте число наиболее ценных пользователей.
Данные поставщика атрибуции можно использовать не только для оптимизации рекламных расходов, но и для улучшения таргетинга и увеличения релевантности рекламных сообщений.
Анализируя производительность, вы можете добиться понимания того, какие действия и метрики ассоциируются с вашими самыми ценными пользователями, и какие метрики указывают на вероятность того, что пользователь удалит ваше приложение.
На основании этих данных вы можете сегментировать аудитории исходя из таких метрик, как просмотры рекламы, установки, конкретные события и сумма прибыли за определенный период времени.
Правила можно комбинировать и настраивать для создания похожих аудиторий (исходя из модели поведения активных пользователей), ретаргетинга аудиторий для новых пользователей, а также настройки списка исключений активных пользователей из кампаний привлечения.
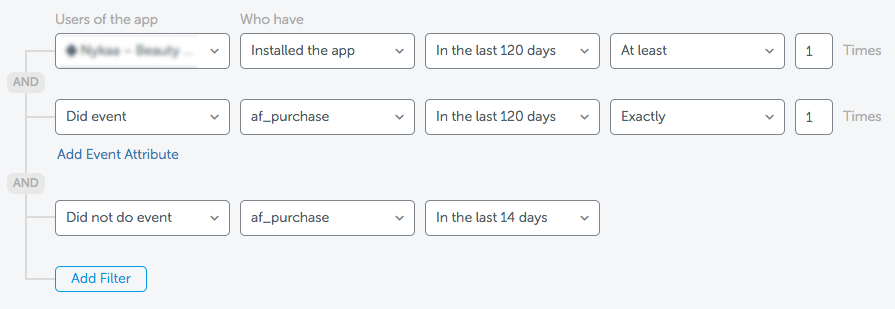
Аудитории могут быть синхронизированы в режиме реального времени со всеми вашими интегрированными рекламными партнерами, включая партнера по аналитике продуктов и партнера по автоматизации маркетинга.
5. Оптимизация пути пользователя с помощью диплинкинга и смарт-баннеров.
Как упоминалось ранее, диплинки – это важный инструмент, позволяющий упростить взаимодействие пользователей с вашим приложением и брендом на разных каналах, платформах и устройствах.
Диплинки могут повысить коэффициент конверсии в 2,5 раза и удержание в 2,1 раза благодаря бесшовному процессу конверсии и контекстуальному опыту, основанному на интересах пользователя и истории взаимодействия с брендами.
Диплинки также могут использоваться для превращения ваших веб-пользователей в ценных пользователей приложений с помощью смарт-баннеров .
Возьмем в качестве примера электронную коммерцию: учитывая, что 70% мобильных покупок происходит в приложении, неудивительно, что коэффициент конверсии в приложениях 3 раза выше, чем в вебе. Имейте в виду, что когда вы привлечете пользователя в свое приложение, вы можете поддерживать с ним постоянную коммуникацию с помощью push и in-app сообщений.
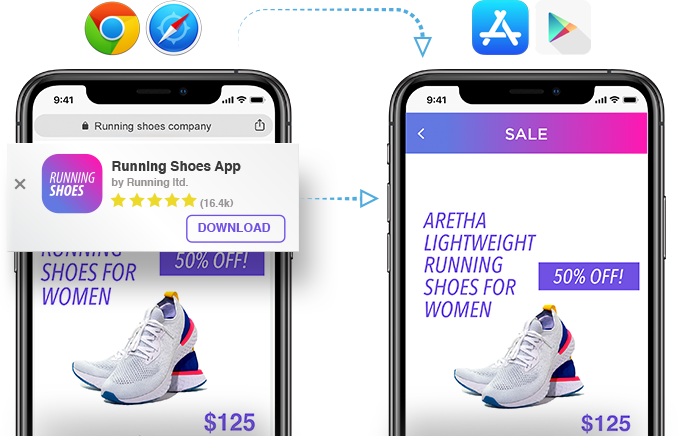
Оставьте работу с данными специалистам
Если на этом этапе у вас все еще остались сомнения относительно того, нужен ли вам поставщик атрибуции, вот еще примеры.
Например, если вы перевели ваш веб бизнес в приложение, у вас может быть соблазн продолжать использовать существующий набор продуктовой аналитики для веба. Вы безусловно получите некоторые инсайты благодаря инструментам автоматизации маркетинга и бесплатным продуктам атрибуции, таким как Firebase.
И хотя некоторый функционал действительно можно построить “своими силами”, в долгосрочной перспективе это не всегда оправданно.
Многие функции поддерживаются только комплексным инструментом, например: разнообразные партнерские интеграции, атрибуция до и после установки, Universal Links и безопасность для данных аудиторий.
Такое разнообразие функций может оказаться неподъемным для небольших компаний. Добавьте к этому сложность работы в разных средах (мобильной и веб-среде), где нужны разные методологии, и вероятность того, что что-то пойдет не так, только возрастет.
Еще одно соображение – это способность эффективно защитить себя от мошенничества.
DИз-за невозможности вручную противодействовать всем формам мошенничества, и времени, необходимого для постоянного обновления технологий, инвестиции в стороннее антифродовое решение обычно окупаются. Поставщики атрибуции лучше оснащены ресурсами, знаниями и масштабами для борьбы с мошенничеством.
В конечном итоге поставщик атрибуции может предоставить вам ценнейшие инсайты и возможности вне зависимости от того, на каком этапе роста находится ваш бизнес. Чтобы узнать больше о том, как атрибуция может способствовать вашему росту, запросите демо или ознакомьтесь с нашим руководством по началу работы с мобильной атрибуцией.
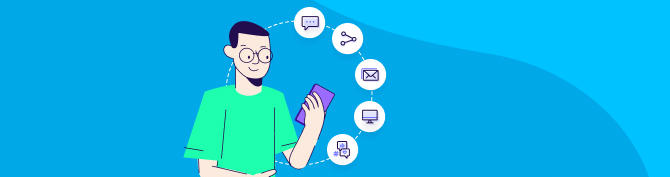
Глава 3
Глубокое погружение в автоматизацию маркетинга
Автоматизированные стратегии взаимодействия с клиентами могут быть проблематичными для брендов.
Сколько раз вы по ошибке получали оповещение или имейл, который был рассчитан на кого-то другого?
А было ли такое, что вы получали сообщение позже, чем рассчитывали? А как насчет повторяющихся сообщений?
Со всеми такое случалось, и такие вещи имеют огромное влияние на бренд.
Некоторые клиенты могут отписаться от ваших сообщений, чтобы не получать такой “спам” в будущем. В любом случае неэффективное общение с клиентами может иметь серьезные последствия для бизнеса.
Сегодня потребители ожидают качественного взаимодействия с брендом на всех каналах и устройствах.
Кроме того, пользователи более критично оценивают контент, присылаемый брендом. Мы видим, что такое поведение пользователей находит отражение в технологиях – например, Apple, который предоставил пользователя больше контроля над своими push-уведомлениями и Центром уведомлений.
Более высокие ожидания пользователей заставляют бренды продуманно относиться к взаимодействию с пользователями с самого начала.
В конце концов, мы все люди.
IЧтобы строить продуктивные отношения с пользователями, бренды должны создавать персонализированный опыт на основе понимания пользовательского поведения.
Благодаря интеграции маркетинговой автоматизации маркетинга в ваш стек технологий, бренды могут более эффективно персонализировать опыт клиента.
Платформы автоматизации маркетинга, также известные как платформы взаимодействия с клиентами, помогают брендам организовывать, тестировать и автоматизировать взаимодействие со своими клиентами через собственные каналы, такие как обмен сообщениями в приложениях, push-уведомления, имейл маркетинг и SMS.
Создание интегрированного стека технологий для взаимодействия с клиентами
Стеки маркетинговых технологий бывают разных форм и размеров. Стартапы могут обойтись собственными системами для push-уведомлений и электронной почты.
Однако по мере роста компаний становится все труднее эффективно масштабировать и автоматизировать кампании без привлечения аналитиков и инженеров.
В последние годы мы наблюдаем изменение отношения брендов к динамичности данных.
Раньше брендам было сложно консолидировать собственные и сторонние данные. Для создания и оптимизации маркетинговых кампаний требовалось вмешательство инженеров и работа с Excel.
Процессы, обрабатываемые вручную, затрудняли обработку кроссканальных данных в реальном времени. По мере того как технологии становятся все более специализированными, бренды создают комплексные стеки маркетинговых технологий.
Сегодня платформы автоматизации маркетинга, такие как Braze, позволяют маркетологам использовать более эффективные стратегии взаимодействия с клиентами.
Бренды могут фильтровать большие наборы данных или обогащать свои данные с помощью сторонних интеграций, таких как AppsFlyer или Mixpanel. Анализируя результаты тестов межканального обмена сообщениями с разбивкой по сегментам аудитории, бренды могут создавать более релевантные сообщения.
Например, фильтрация аудитории может быть привязана к правилам, которые запускают сообщения в реальном времени после того, как клиенты совершают определенные события в вашем мобильном или веб интерфейсе. Кроме того, функция Connected Content от Braze позволяет создавать масштабный персонализированный контент для ваших клиентов с помощью API.
Теперь межканальный обмен сообщениями может быть организован в рамках единого интерфейса, такого как Canvas Braze. Он позволяет автоматизировать синхронизированный обмен сообщениями для электронной почты, push-оповещений, in-app сообщений и в новостной ленте приложения как на десктопе, так и на мобайле. Благодаря партнерской интеграции обмен сообщениями может быть настроен и на других каналах, таких как платная реклама, SMS и прямая почтовая рассылка.
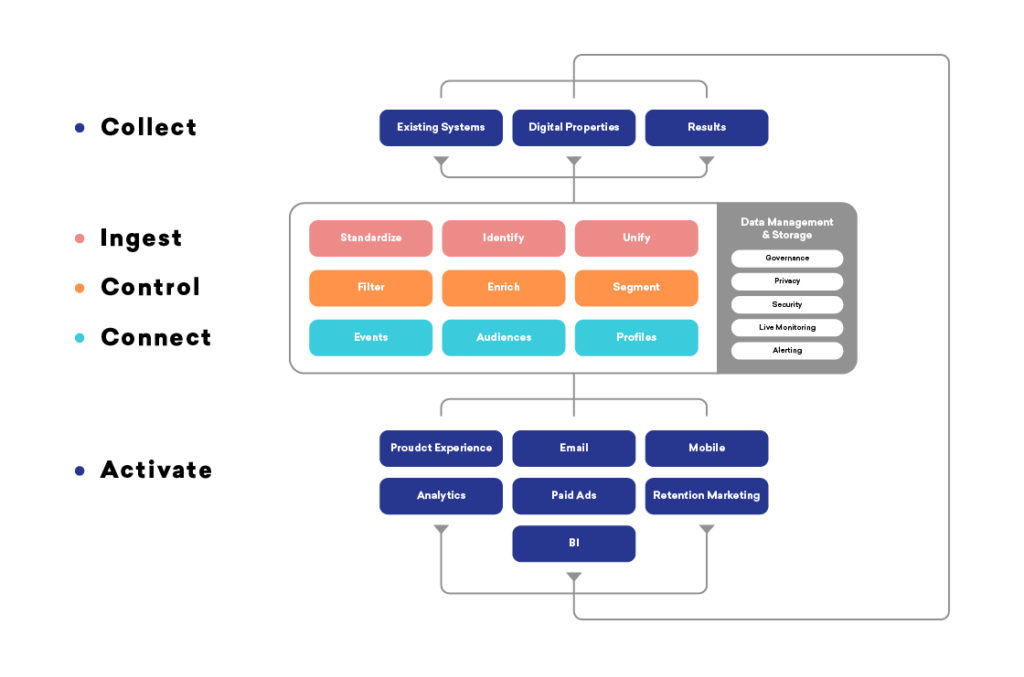
Как показано на изображении выше, эти варианты использования становятся еще более эффективными, когда ваша платформа автоматизации маркетинга позволяет осуществлять целостный сбор и обработку данных.
Например, многие бренды считают полезным использовать CDP, такой, как mParticle или Segment, для оповещений, централизации и стандартизации данных о клиентах в собственных и сторонних системах, что обеспечивает передачу данных в реальном времени между инструментами стека.
Это обогащает данные и позволяет обмениваться ими с платформами аналитики продуктов и атрибуции, что дает брендам возможность быстро синхронизировать аудитории и обмен сообщениями в рамках платных и собственных кампаний.
Поскольку большинство платформ автоматизации маркетинга не имеют в распоряжении универсальных ссылок, в предыдущей главе этого руководства подчеркивалась важность использования диплинков от поставщика атрибуции, такого как AppsFlyer.
Создание качественного взаимодействия с пользователями
Интеграция стека маркетинговых технологий может открыть безграничные возможности для брендов в плане улучшения пользовательского опыта.
Давайте рассмотрим некоторые передовые практики.
Персонализация онбординга для создания “неизгладимого” впечатления
Первые несколько дней взаимодействия с новым пользователем имеют решающее значение. Если клиенты сразу не увидят для себя ценности в вашем продукте, они, скорее всего, не будут его использовать
Бренды должны создавать такие условия, чтобы:
1. Пользователи чувствовали, что их ценят.
Это может сводиться к приветственному имейлу или выражению благодарности за подписку.
Вы можете узнать ваших клиентов получше, спросив их, какие каналы получения сообщений они предпочитают.
Push notification opt-in prompts via in-app messaging have been widely used across web and mobile devices as a means to ask for permission and highlight the value of the content the customer will receive if they enable push.
Если вы отправляете данные атрибуции из AppsFlyer в Braze, вы также можете настроить онбординг и контент в зависимости от источника, из которого был привлечен клиент.
Использование последовательных сообщений с самого начала поможет сделать ваш бренд удобным и убедительным.
Например, если пользователи установили ваше приложение в результате просмотра Google Ad с промокодом, вы можете так настроить обмен сообщениями или имейлы так, чтобы в них была включена та же акция, которая привела пользователей в ваше приложение.
2. Пользователи должны увидеть ценность вашего продукта с самых первых шагов.
Убедитесь, что вы заранее разработали стратегии, которые помогут вашим пользователям лучше познакомиться с приложением или веб-сайтом.
Например, если у вас есть приложение для электронной коммерции, вы можете рассказать пользователям, насколько легко находить продукты, покупать и получать вознаграждения за повторные покупки в будущем.
Путь каждого пользователя уникален.
Чтобы узнать больше о передовых методах, ознакомьтесь с гайдом Braze по созданию персонализированного опыта пользователя при онбординге.
Продолжайте создавать персонализированный опыт пользователя после процесса онбординга
Привлечение новых клиентов через привлекательный процесс онбординга- это только первый шаг.
Построение длительных отношений может быть сложной задачей, требующей времени и постоянного взаимодействия, чтобы добиться лояльности пользователей.
1. Всегда будьте на шаг впереди, предвосхищая запросы и поведение пользователей.
Иногда пользователям необходима мотивация, чтобы сделать следующий шаг к конверсии.
Их нужно немного подтолкнуть!
Отправьте им сообщение, в котором они найдут контент, необходимый для того, чтобы сделать следующий шаг. Может быть, вам нужно напомнить им о товаре, который они отложили в корзину. Если они вышли из игры, дайте им стимул вернуться и завершить уровень.
Неважно, что именно вы предлагаете пользователям, главное – найти способ не потерять с ними связь.
2. Убедитесь, что каждое сообщение является релевантным и своевременным.
Многие бренды слишком часто используют сообщения.
И что в результате? Увеличивается число отписок, отказов от рассылки и даже удалений.
Не попадитесь в эту ловушку.
Хотя в некоторых случаях массовые рассылки необходимы, (имейлы, связанные с GDPR или новыми условиями обслуживания), в большинстве случаев лучше отправлять кастомизированные сообщения, основанные на интеракциях конкретного пользователя с вашим контентом.
Например, если пользователь игрового приложения покидает приложение после завершения уровня 9, отправьте ему сообщение о том, как близок он был к прохождению уровня 10.
Кроме того, можно использовать API, которые помогут осуществить сегментацию и персонализацию. От рекомендаций по продуктам до персонализированных сообщений на основе прогнозов погоды в реальном времени – динамический контент может привести к мощным результатам.
3. Наладьте эффективное общение по нескольким каналам.
У каждого клиента разные предпочтения относительно того, как они хотят получать сообщения.
Поэтому важно, чтобы ваши пользователи смогли сами (через настройки оповещений) выбрать тип и способ коммуникации. Когда у пользователя есть выбор, он вряд ли захочет полностью отключить оповещения.
Независимо от устройства или канала всегда следует использовать диплинки, чтобы обеспечить конверсию, содержащую данные атрибуции. Это позволит вам понять, как ваши клиенты реагируют на каждый канал, и внести соответствующие изменения в персонализацию.
Мысли в заключение
Современные технологические стеки построены вокруг вовлечения пользователей, что позволяет брендам создавать персонализированный опыт для каждого отдельного клиента на разных каналах.
Важность такой персонализации бесценна, поскольку эффективные стратегии обмена сообщениями на разных каналах могут повысить вовлеченность клиентов на 844%. Гуманизация цифрового опыта приведет бренды к повышению лояльности клиентов и, в конечном итоге, к улучшению результатов бизнеса.
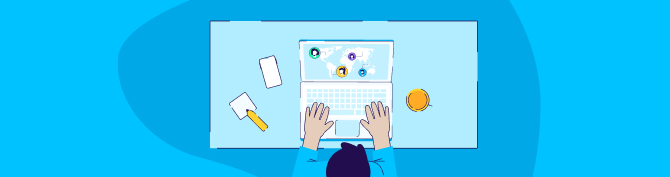
Глава 4
Глубокое погружение в продуктовую аналитику
Рост положил начало эпохе, в которой центральное место отводится науке о больших данных. Именно она имеет решающее значение для обеспечения наилучшего обслуживания клиентов. Каждая точка соприкосновения с брендом пытается создать уникальный пользовательский опыт, созданный на основе данных и оптимизированный с помощью их интерпретации.
В предыдущих двух главах мы подчеркнули важность мобильной атрибуции и автоматизации маркетинга для оптимизации пути пользователя.
Хотя обе эти технологии предоставляют аналитику, которая способствует лучшему пониманию пользователя, изначально они не были предназначены для оптимизации пользовательского опыта.
Именно по этой причине основой высокоэффективного маркетинга и роста стала аналитика продукта.
Аналитика продукта – это изучение и понимание вашего продукта и бизнеса посредством анализа взаимодействия пользователей с продуктом, а также факторов, приводящих к успеху.
Традиционным, нецифровым бизнесам часто не хватает преимуществ, которые дают большие данные, сверхсовременные модели машинного обучения и другие процессы, способствующие пониманию поведения пользователей и росту.
Даже самые оснащенные в ресурсном отношении бизнесы могут потратить годы на то, чтобы добиться эффективной практики.
Осознавая это, компании обратились к таким платформам аналитики, как Mixpanel для решения сложных задач, ориентированных на цифровые технологии, начиная от поведенческого анализа до сегментации пользователей.
Аналитика продукта не возникла на пустом месте, ее развитие было обусловлено тем, что старые технологии больше не могли удовлетворить потребности цифровых компаний.
Сила продуктовой аналитики “self-serve”
Инструменты продуктовой аналитики сводят маркетологов, менеджеров по продуктам, инженеров и специалистов по данным на единой платформе, предназначенной для тестирования, понимания и оптимизации продуктового опыта (Product experience) на разных устройствах.
Они предоставляют множество самостоятельных вариантов использования, включая оптимизацию потока воронки, когортный анализ, сегментацию пользователей и интеграцию с другими партнерами по стеку. Более продвинутые платформы продуктовой аналитики, такие как Mixpanel, также предоставляют функции таргетированного взаимодействия, которые пересекаются с автоматизацией маркетинга, включая push-уведомления, электронную почту, SMS и обмен сообщениями внутри приложений.
Основой современных аналитических продуктов инструментов является самостоятельное использование (self-serve), то есть отсутствие необходимости в специалисте по бизнес аналитике.
Аналитика продуктов способствует демократизации данных, позволяя разным командам совместно решать возникающие проблемы и не зависеть от специалиста по данным.
Это позволяет маркетологам и специалистам по продукту быстро и эффективно решать вопросы, связанные с данными и поведением пользователей. Кроме того, это позволяет специалистам по данным не отвлекаться на такие базовые задачи, как создание простых дэшбордов и сверка данных, а сконцентрировать усилия на более стратегически важных и сложных проблемах.
В итоге продуктовая аналитика решает три важных задачи в цикле роста:
- Понимание вовлеченности пользователей, клиентского опыта и полного жизненного цикла вашего продукта – от событий в самом начале воронки (то есть клики и атрибуция), до событий внизу воронки, то есть в самом приложении.
- Повышение качества обслуживания клиентов за счет глубокого анализа KPI и больших возможностей цифровой аналитики.
- Использование результатов этого анализа для улучшения платформы и партнерских интеграций.
Теперь, когда у нас есть общее представление о продуктовой аналитике, давайте рассмотрим несколько основных вариантов использования в рамках более обширного набора маркетинговых технологий.
Пример # 1: понимание пользователей с помощью поведенческой аналитики
Возьмем типичный пример розничного приложения из списка Fortune 500, в котором UA маркетолог пытается понять путь пользователя по всей воронке конверсии.
Маркетологов по привлечению пользователей интересуют несколько вещей.
- Во-первых, они хотят понять, с какой скоростью пользователи совершают конверсию к основной метрике; для розничного приложения это покупка продуктов.
- Во-вторых, они хотят понять, что привело пользователей к атрибуции.
Атрибуцию можно разделить на объективные и субъективные измерения…
Давайте сначала поговорим об объективных измерениях.
Воронка атрибуции начинается с того, что пользователь нажимает на рекламу, показанную на одном из медиа-источников клиента, например, Facebook, Google или Apple Search Ads.
Посредством диплинка пользователь переходит от рекламы на веб-сайт или к приложению (если оно у них есть), либо к магазину приложений, чтобы установить приложение.
После того как пользователь установит и откроет приложение, поставщик атрибуции (например, AppsFlyer) приписывает этого пользователя соответствующему медиа-источнику, чтобы клиент мог объективно измерить, какие медиа-источники и варианты креативов привели к наиболее эффективным конверсиям.
После установки в игру вступают субъективные критерии атрибуции.
В конечном итоге маркетолога больше всего интересует рентабельность инвестиций в рекламу (ROAS). Это зависит от опыта пользователя как на привлекшем его медиа-источнике, так и от процесса онбординга в самом приложении или на веб-сайте. После онбординга на решение пользователя совершить покупку влияет масса дополнительных факторов.
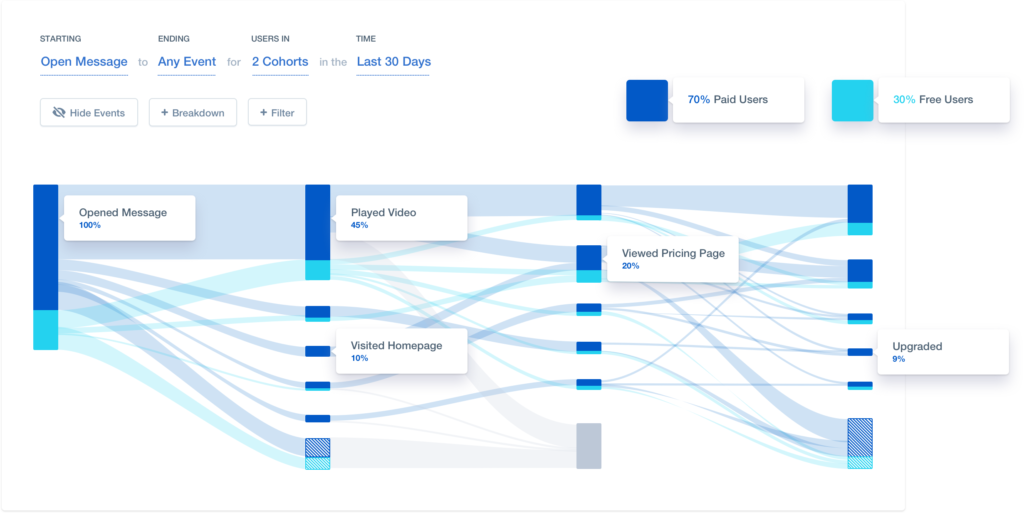
В Mixpanel одним из инструментов для понимания этого пути является Flows (см. выше).
Этот инструмент позволяет маркетологам и менеджерам по продукту сопоставить самые распространенный сценарий событий, который использует пользователь, и определить возможные препятствия в процессе онбординга или негативный UX.
Несмотря на то, что Flows дает анализ основных факторов и тенденций, основные инструменты аналитики продуктов заключаются в сегментации, анализе удержания и группировке когорт. Каждая из этих автономных функций способствует более глубокому пониманию поведения пользователя.
Например, отчет по воронке от Mixpanel (Funnels) позволяет увидеть, как ваши пользователи совершают конверсию при определенном сценарии событий. Это означает, что вы можете выявить возможности для увеличения коэффициента конверсии, определив, когда и где пользователи покидают приложение (или не совершают конверсию) в вашем продуктовом цикле или в маркетинговых кампаниях.
Аналогичным образом, анализ удержания позволяет командам увидеть, возвращаются ли новые пользователи, которые пробуют ваш продукт после добавления, изменения, улучшения функций или последовательности событий.
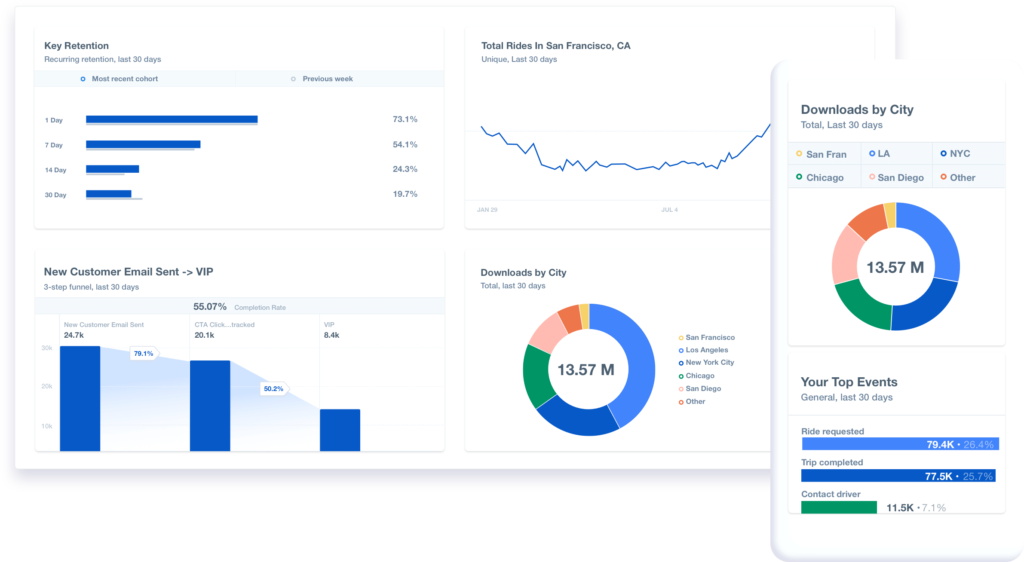
Благодаря этим аналитическим возможностям команды могут принимать самостоятельные решения о том, в какие платные медиа-платформы инвестировать, или какой сценарий событий изменить с помощью инженеров и специалистов по продукту.
Пример # 2: Оптимизация рекламных сообщений и последовательности событий с помощью когортного анализа
Возьмем еще один пример, на этот раз компанию по видео-стримингу, работающую на основе подписки, которая хочет понять, как предоставить актуальный контент для своих пользователей.
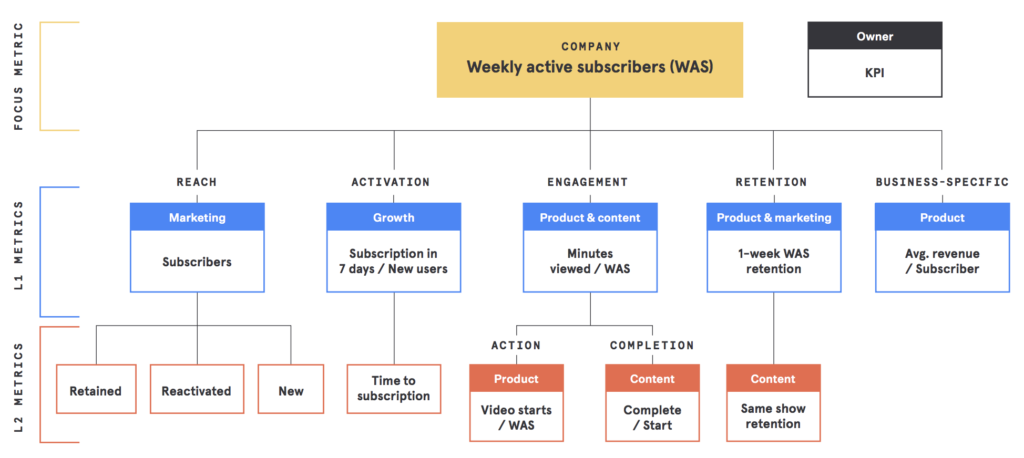
После того как пользователь привлечен и атрибутирован на правильный источник, компания видео-стриминга хочет понять, сколько времени пользователь просматривает контент, и на какой контент он тратит больше всего времени.
Частота и повторные просмотры являются основными показателями успеха.
Если группа разработчиков продукта работает над новым интерфейсом, основанном на AI и предлагающем контент, им важно проанализировать, сколько часов пользователь проводит за просмотром определенного контента. Таким образом, они могут создавать когорты в зависимости от типа контента и количества часов просмотра.
Группа разработчиков продукта может создать эти когорты и передать данные в формате необработанных данных в свои модели AI и машинного обучения. Или они могут экспортировать эти когорты обратно на инструмент маркетинговой атрибуции, такой как AppsFlyer. Маркетинговая группа также может создавать свои собственные когорты для сравнения, изучая показатели удержания и процент “бросания” конкретного контента в течение определенного периода времени.
Возможности активации безграничны. Команды по продукту и маркетингу могут работать вместе, чтобы экспериментировать с последовательностью событий в онбординге, объединяя данные атрибуции (медиа-источник, кампания, тип рекламы и т. д.) с когортами продуктовой аналитики.
В Mixpanel когорты можно дополнительно активировать с помощью наших встроенных функций обмена сообщениями или интеграции с партнерами по автоматизации маркетинга. В конечном счете, единственное ограничение – это творческий подход команды и ее желание экспериментировать и совершенствоваться.
Пример № 3: Прогностическая аналитика данных
Помимо настраиваемых отчетов и сегментации, на некоторых платформах продуктовой аналитики, включая Mixpanel, есть дополнительные приложения искусственного интеллекта (AI), которые открывают доступ к анализу данных как для маркетологов, так и для специалистов по продукту.
Например, модель прогнозирующей аналитики Mixpanel использует прошлое поведение, чтобы выявить, какие пользователи, вероятно, выполнят действие, а какие нет.
Это позволяет настраивать таргетинг на потенциальных качественных пользователей с помощью своевременных рекламных оповещений и специальных предложений с помощью системы автоматизации маркетинга. Эта модель также позволяет проводить платный таргетинг и тестирование инкрементальности посредством интеграции аудиторий с поставщиками атрибуции, такими как AppsFlyer.
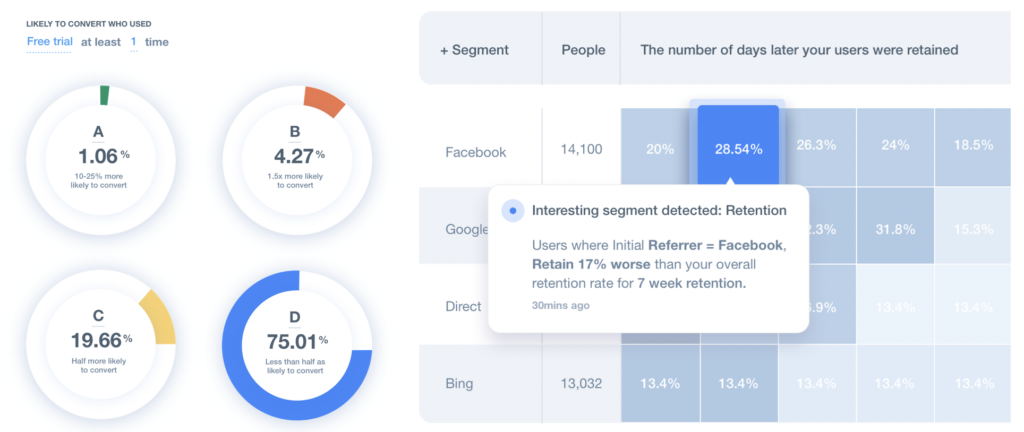
Справа: узнайте с помощью автоматической сегментации, какие пользовательские сегменты вызывают всплески показателей.
Эти данные могут быть дополнительно усилены с помощью автоматической сегментации и с помощью обнаружения аномалий.
Обнаружение аномалий уведомляет вас, когда важные показатели неожиданно резко повышаются или падают, а также показывает пользователей, вызывающих эти изменения.
Эти автоматические инсайты могут применяться к тестированию UX продукта, обмена сообщениями и даже к распределению платных медиа.
Узнать больше
In each case, product analytics enables down-the-line contributors to take control of their business outcomes and better serve В каждом случае аналитика продуктов позволяет всем участникам процесса, независимо от уровня, контролировать результаты бизнеса и предоставлять улучшенный сервис. Это дает возможность командам быть более эффективными, стимулируя рост на всех уровнях организации.
Чтобы узнать больше о передовых методах анализа продуктов, ознакомьтесь с Руководством Mixpanel по метрикам продуктов.
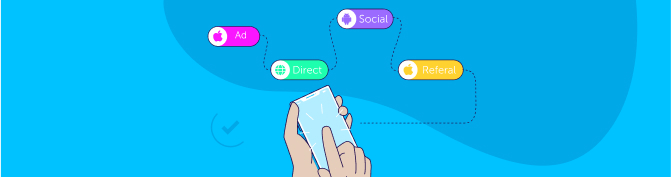
Глава 5
Глубокое погружение в данные: платформа пользовательских данных
Теперь, когда большинство активности пользователей сконцентрировано на мобайле, маркетологам становится непросто отследить все передвижения пользователя на разных устройствах. Ожидается, что бренды смогут собрать воедино разрозненные действия пользователя по разным устройствам и составить целостную картину пути клиента по всем точкам взаимодействия с брендом: на вебе, в приложении, имейле и т.д.
В эпоху подключения к интернету маркетологи должны не только идентифицировать действия одного и того же пользователя на разных каналах и устройствах, но и делать это, не нарушив его конфиденциальность.
Еще одна задача, стоящая перед маркетологами – это соединить эти данные с новыми инструментами и поставщиками для осуществления непрерывного тестирования новых возможностей для роста.
TИменно здесь в игру вступает платформа клиентских данных (CDP).
Современные платформы CDP, такие, как mParticle, помогают брендам использовать свои собственные данные о клиентах, чтобы улучшить путь пользователя как на мобильных устройствах, так и на десктопе за счет функции идентификации пользователя на разных устройствах.
В частности, CDP способствует:
- стандартизированной реализации новых SDK через партнерские плагины CDP;
- налаживанию передачи данных в реальном времени между партнерами по стеку. Такие данные можно использовать для обогащения и унификации идентификаторов клиентов на разных устройствах;
- поддержке стандартов безопасности пользовательских данных для разных партнеров.
MarTech стал более узкоспециализированным
Чтобы идти в ногу с новыми тенденциями в эпоху мобильного интернета, среднему маркетинговому стеку требуется все больше и больше инструментов.
Тенденция к узкоспециализированным инструментам имеет свои плюсы и минусы; плюсы заключаются в том, что специализированные технологии больше подходят для решения конкретных узких задач, таких как мобильная атрибуция и защита от фрода. Минусы этой тенденции заключаются в том, что она вызывает необходимость в более крупном маркетинговом стеке, который будет собирать все необходимые данные воедино.
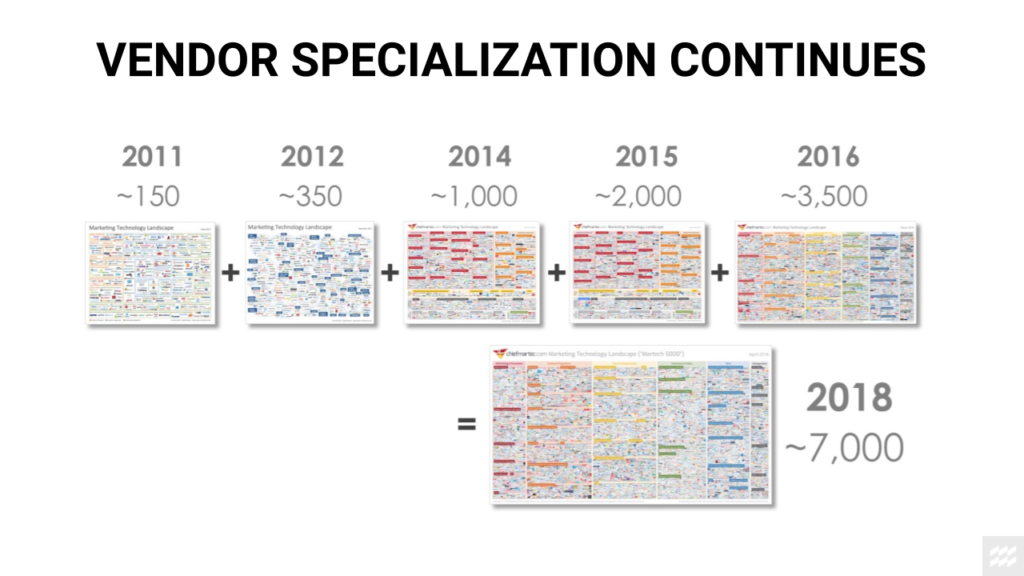
Более крупный стек не представлял бы из себя проблему, если бы существовала единая система условных обозначений на разных инструментах.
К счастью, CDP способны трансформировать и объединять данные из разных систем и создавать единое представление пути клиента, независимо от несоответствия между системами.
Это позволяет маркетологам беспрепятственно отправлять пользовательские данные на встроенные интеграции с более 250 поставщиками.
CDP помогают маркетологам быстро запускать и тестировать новые инструменты, так как эти встроенные интеграции почти не требуют поддержки со стороны разработчиков для установки и обслуживания.
Данные в реальном времени делают возможным доступ к пути клиента на разных устройствах и платформах
Ключевое слово в эпоху мобильного интернета
это подключение к интернету, благодаря которому мобильные устройства постоянно подключены к почте, социальным сетям, приложениям и так далее. Пользователи ожидают, что маркетологи будут соответствовать веянию времени и смогут понять, что за действиями на разных платформах стоит один и тот же пользователь.
Для этого маркетологи должны иметь доступ к этим данным на разных устройствах в режиме реального времени.
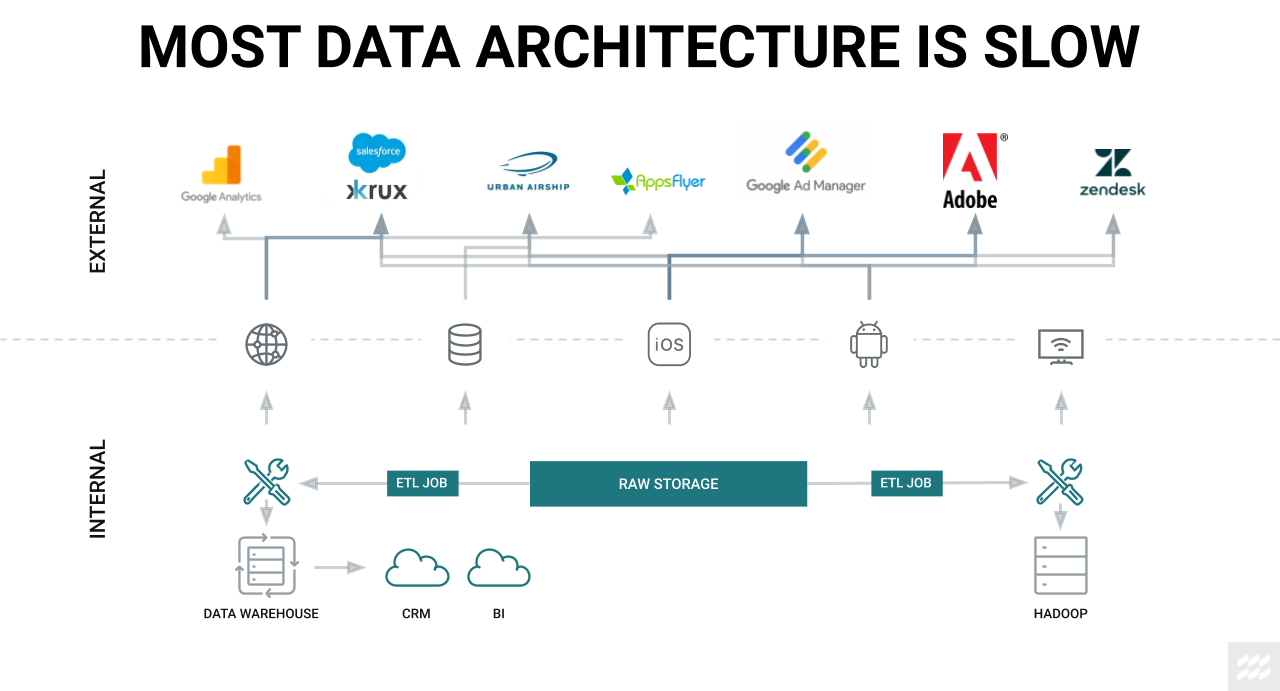
В отличие от предшествующих DMP, CDP используют API-интерфейсы для сбора данных в реальном времени для создания полных профилей клиентов, независимо от источника. Эти данные могут передаваться на сервисы и платформы в вашем стеке, что позволяет предпринять немедленные действия.
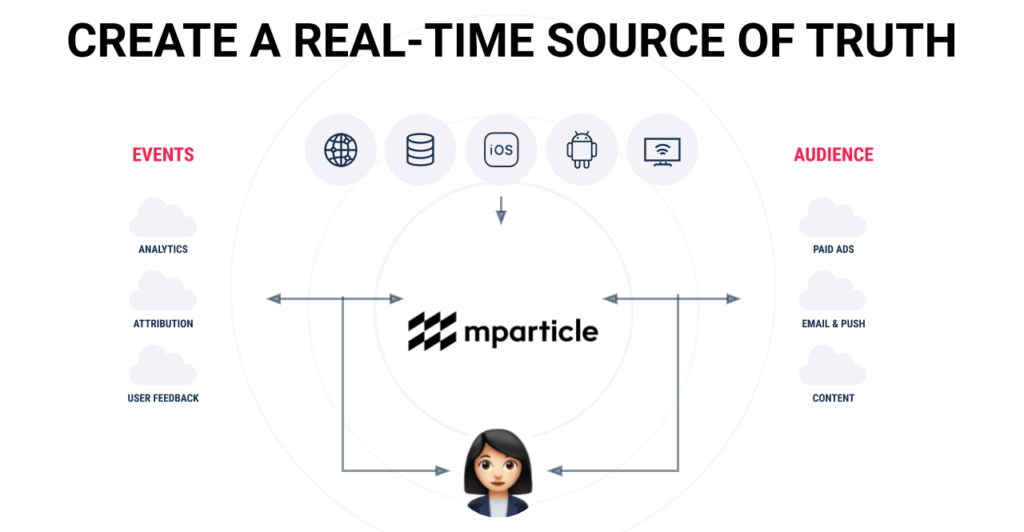
Использование данных в режиме реального времени дает брендам возможность сделать взаимодействие с клиентами более релевантным и контекстуальным.
Например, компания по продаже кроссовок GOAT использует технологию AppsFlyer для мобильной атрибуции, а технологию mParticle CDP для синхронизации данных между всеми инструментами в своем стеке.
mParticle связывает историю событий GOAT на мобильных устройствах и десктопе, например, просмотры экранов и добавления в корзину, что позволяет GOAT анализировать действия пользователей на разных каналах.
GOAT также использует технологию mParticle для создания аудиторий и обновления кампаний по автоматизации маркетинга в режиме реального времени. Это обеспечивает более релевантное и контекстуальное взаимодействие с пользователями.
Предусмотренная защита конфиденциальности
Бренды должны согласиться с тем, что данные клиентов являются самым ценным активом, доступным в 2019 году. Клиенты также начали требовать усиления контроля над тем, как собираются и обрабатываются их личные данные.
Бесконтрольное управление данными клиентов уже в прошлом, на фоне действующих положений GDPR и грядущего принятия CCPA в США.
Компании, полагающиеся исключительно на устаревшие системы управления данными, которые не соответствуют стандартам GDPR, будут вынуждены платить штраф в размере до 4% от общего дохода или вообще прекратить сбор данных, чтобы избежать наказания. CDP платформы созданы как раз с учетом конфиденциальности и безопасности данных, так что соблюдение новых норм и положений будет осуществляться без ущерба для анализа пользовательских данных.
И в заключение…
Приобретение платформы CDP в самом начале формирования вашего маркетингового стека дает огромные преимущества в плане соответствия правовым нормам, межканальных отчетов и обмена данных с партнерами.
Для компаний с менее модернизированным стеком, добавление CDP станет тактическим шагом, который послужит оптимизации старых технологий и открытия для себя новых вариантов использования (например, оптимизация в реальном времени, синхронизация аудитории для повторного вовлечения на платных и собственных медиа-каналах, многоканальное A/B-тестирование и т. д.).
Все это сводится к качеству пользовательского опыта.
В 2019 году единственной возможностью для маркетологов создать правильную коммуникацию с пользователем в нужное время и в нужном месте – это получить нужные данные в соответствующей системе в режиме реального времени.
Чтобы узнать больше о CDP, ознакомьтесь с этим подробным гайдом.