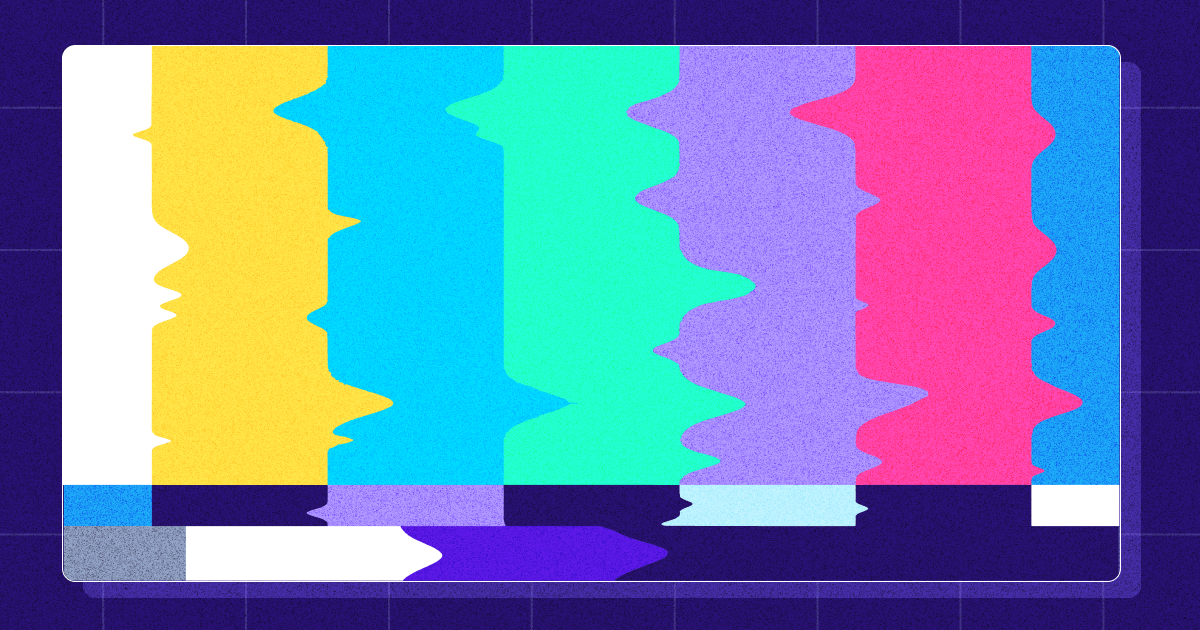
Media mix modeling (MMM)
Media mix modeling (MMM) measures the impact of marketing and advertising campaigns to determine how internal and external elements contribute to a desired outcome, be it revenue or any other KPI.
What is media mix modeling (MMM)?
Media mix modeling, also known as marketing mix modeling, is a statistical method used to measure the impact of marketing and advertising campaigns. Specifically, it reveals how the 4Ps of the marketing mix — product, price, place, and promotion — are contributing to a particular goal, which is often increasing conversions.
While the 4Ps represent the four broad categories, here are some of the most common elements measured in MMM:
- Sales data: Measures the effectiveness of various marketing strategies and campaigns in driving desired business outcomes, such as more app downloads or revenue.
- Customer data: Monitors demographic and behavioral information about customers, such as income, age, and purchasing habits.
- Media spend: Measures the total money spent on different media formats and types.
- Media exposure: Measures media reach, frequency, and gross rating points.
- External factors: Includes factors influencing sales and marketing performance, such as competitor activity, economic conditions, and seasonality.
We’ll discuss the elements in more detail later in the article.
Think of it this way: using MMM, you can identify which elements of your mobile marketing strategy are working and which aren’t, allowing you to tweak your approach and optimize your campaign.
How does MMM work?
MMM uses statistical analysis to understand how different marketing efforts affect business outcomes like sales. Using a technique called multi-linear regression analysis, it enables you to link independent variables (such as marketing spend on different channels or user engagement metrics) to a dependent variable (such as app downloads or revenue).
The idea here is to evaluate multiple models to accurately answer the ‘What will happen if you make this change?’ question.
For example, you can use MMM to measure the impact of in-app ads on total revenue. Then you can look at the effect of increased spending on these ads: would it earn you more, or less?
To use MMM effectively, you need aggregated and cleansed data from internal databases and external sources. Ideally, your data will span two to three years to factor in effects like seasonality. Then, you assign a numerical value to every media channel campaign based on the return on investment (ROI), and use this to allocate future spend and create sales forecasts.
The four phases of an MMM process
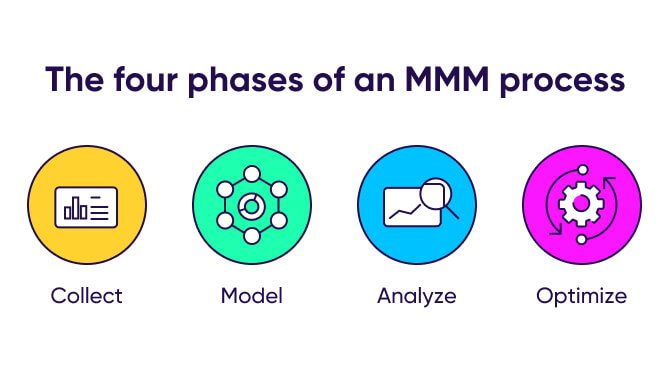
A standard effective MMM process has the following four phases:
Phase 1: Data collection
After the impending demise of third-party cookies, you need to focus on collecting first-party data for a more accurate representation of user reactions and behavior in response to your marketing strategy.
Gather comprehensive historical data on your past marketing activities, non-marketing sources, and external factors. Think: user engagement metrics, target audience demographics, and ad spend.
You also need to ensure data integrity, using methods such as second-party data partnerships (getting information from current or potential business partners for database enrichment) and data clean rooms (using aggregated and anonymized user information to protect user privacy).
Phase 2: Modeling
MMM works best with digital channels: traditional methods like print and broadcast are harder to measure. As a mobile app marketer, you can use multi-linear regression to determine the ROI with accurate, dependable insights for decision-making.
To create an MMM model, choose the dependent variable or business outcome (for example, revenue or app downloads) you want to explain. Then identify the independent variables, a.k.a. factors impacting the dependent variable (things like ad spend and target audience).
Make sure you’re including both controllable variables like price and channel, and uncontrollable variables like competition and inflation.
Finally, assign values to both the dependent and independent variables, and create a mathematical model representing the relationship between them.
Phase 3: Data analysis and insights
In this stage, you’ll use the model from phase 2 to uncover and analyze insights related to your marketing campaigns.
Evaluate the contribution of each channel to the business outcomes and dependable metrics you identified before. Continuing with our example, you can rank your marketing campaigns based on their impact on revenue or user engagement. From there, you can measure media effectiveness, efficiency, and ROI for each campaign.
Note that you can use your model for forecasting future user engagement and revenue. But models based on historical data assume past patterns will repeat in the future and, therefore, don’t account for landscape changes.
Phase 4: Optimization
Optimization is the final MMM phase, where you optimize your marketing mix for future campaigns using your results from phase 3.
Consider simulating different marketing scenarios, targeting different audiences, or changing ad spend levels to identify the optimal combination of tactics to achieve your revenue goals faster.
MMM in action: an example
Suppose you want to determine the impact of your in-app advertisements on revenue.
Working through the MMM phases, you’ll first collect data on ad spend, target audience demographics, and revenue for the past year. Then, use multi-linear regression to create a model representing the relationship between these variables.
Let’s assume the model shows that ad spend and target audience demographics have a significant positive impact on revenue. That means you can optimize your marketing mix for future campaigns by increasing ad spend on channels with the highest impact on revenue, and focusing your marketing efforts towards a more lucrative audience.
Is MMM the right model for you?
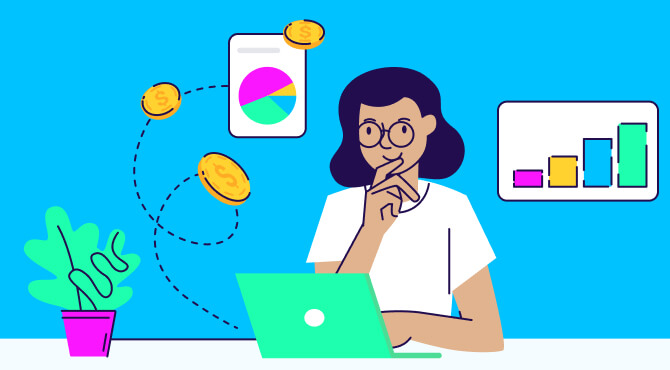
When it comes to MMM, there’s no cookie-cutter approach. You have to consider certain key factors to understand whether it’s the right model for your mobile app marketing campaign. Here’s what to think about:
Budget
Being a data-driven approach, MMM often involves a significant investment in data collection, modeling, and analysis, making the costs prohibitive for smaller app development businesses. Before committing to MMM, make sure you have a clear understanding of your approved budget.
Data availability
Having access to a large and diverse set of data, including historical marketing data and data related to external factors impacting your app’s success, is crucial for MMM to work. Consider the availability of this data and the level of effort required to collect and process it on your end, and whether that’s feasible.
Complexity of the mix
If your app marketing campaign has a complex marketing mix involving multiple channels and tactics, MMM will make a great choice to enhance your campaign outcomes. On the other hand, if your app marketing campaign is simple and straightforward, MMM may not be the most appropriate approach.
Campaign objective
MMM is best when you want to understand the impact of different marketing activities on key business outcomes, such as app downloads or purchases. If your objective is to drive short-term results (for example, user-level engagements like clicks or impressions), data-driven attribution would be a better fit.
We’ll explore the similarities and differences between data-driven attribution and MMM in more detail later.
Skill and expertise
MMM requires expertise in data science, modeling, and marketing analytics. If your team doesn’t have the necessary skills and experience, you may find it difficult to implement the model effectively.
Timeframe
MMM is generally time-consuming, taking several weeks or even months to complete. So, if your app marketing campaign is time-sensitive, MMM may not be the best choice.
How do you measure MMM?
Analysts identify dependent and independent variables and put them into an equation. Depending on the relation between the variables, the equation can be linear or nonlinear, but monitoring and measuring certain elements is crucial when using MMM.
Here’s a breakdown of each element in more detail:
1 — Sales volume
When analyzing sales volume in MMM, you need to divide total sales into two components: base sales and incremental sales.
- Base sales are driven by underlying factors, such as pricing, long-term trends, seasonality, app awareness, and user loyalty. These generally include economic variables that fluctuate over a specific period.
- Incremental sales are driven by marketing and sales activities. You can break down total incremental sales into segments impacted by each marketing initiative, to see what portion of sales is directly influenced by marketing efforts and how effective those activities are.
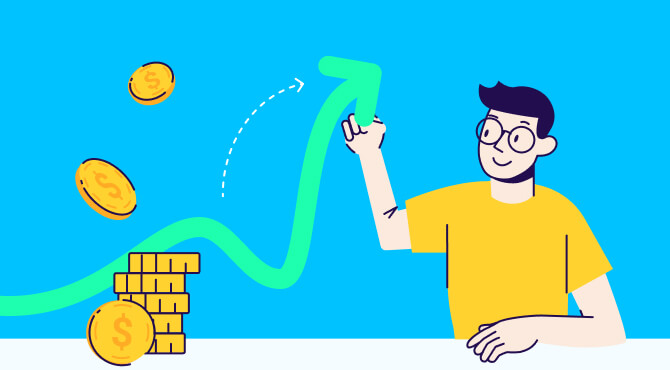
You can further analyze each type of sales volume to understand the specific impact of each marketing activity.
2 — Pricing
Pricing changes have a direct influence on sales volume, and MMM can help quantify this impact.
You can get valuable insights into the direct effects of your pricing decisions by analyzing the relationship between changes in price and changes in sales. Then, using this information, you can optimize your app pricing strategies to achieve desired outcomes.
Suppose you increase the price of your app from $3.99 to $4.99. Using the MMM technique, you find that the price increase resulted in a 5% decrease in in-app purchases, but because of the high price point, your revenue increased by 20%.
With this information, you can continue with your app’s new price point, knowing it’ll result in increased revenue with minimal impact on sales volume.
3 — Media and advertising
MMM is a valuable tool for analyzing the impact of media and advertising on sales across different mediums and channels, including online ads, print, and billboards. Although MMM results may not provide clear-cut answers, they can still give you valuable insights into how changes in advertising strategies influence app sales.
Some examples include:
- Short ads vs. long ads
- Running ads on Facebook vs. Instagram
- Airing ads during prime time versus non-prime time
You can use these insights to optimize your ad spend decisions, making sure you get the most bang for your buck.
4 — Distribution
An efficient distribution system drives growth more effectively than any other element. So, the fact that you can use MMM to determine the impact of changes in distribution efforts on dependent variables is a huge advantage. It gives you a holistic understanding of all your distribution channels and related costs, helping you make informed decisions about the channels to invest in.
Let’s assume you want to expand your distribution efforts for your mobile app.
You can use MMM to analyze the sales data from different distribution channels, such as partnerships, social media platforms, and app stores. If you find that partnering with other popular apps drives app downloads and purchases, you can focus your distribution efforts on building more partnerships, rather than relying on app stores and social media alone.
Media mix modeling vs. data-driven attribution modeling
Data-driven attribution modeling refers to the various attribution models, such as single-touch attribution and multi-touch attribution, that monitor user-level engagements throughout the customer journey.
As an app marketer, you can use these insights to help you understand which tactics have the biggest impact as consumers move through the app journey. These attribution models evaluate performance after a campaign ends.
Generally speaking, media mix modeling and data-driven modeling attribution models help you understand how your marketing tactics affect a specific business objective, like revenue. Both methods also use statistical and mathematical models to analyze data.
But that’s where the similarities end.
MMM doesn’t factor in user-level engagements — instead, it measures the impact marketing efforts have on meeting predetermined business objectives, without considering the customer journey. On the other hand, data-driven attribution focuses on person-level data, such as the total number of impressions and clicks.
Here’s a table to help you compare MMM with data-driven attribution modeling, and the role each can play in mobile app marketing.
Features | Media mix modeling (MMM) | Data-driven attribution modeling |
Purpose | To understand the impact of marketing mix on sales and revenue | To understand the impact of individual marketing touchpoints on conversion |
Data used | Historical aggregated data from marketing activities and external factors | Detailed individual-level data such as clicks, impressions, and conversions |
Modeling approach | Multi-linear regression analysis | Machine learning algorithms |
Key outputs | Marketing mix optimization, media effectiveness and efficiency, ROI, and forecasting | Attribution of conversion to individual touchpoints, allocation of budget and resources |
Timeframe | Uses historical data for several months to a year | Uses real-time or near real-time data |
Complexity | High, due to multiple variables and complex regression models | Low to moderate, depending on the complexity of the attribution model |
Limitations | Assumes past patterns will repeat in the future and doesn’t account for market changes | May not accurately capture the overall impact of the marketing mix and multiple touchpoint interactions |
Advantages and disadvantages of MMM
Most app marketers today don’t have clear goals and KPIs, and rely solely on ‘vanity’ metrics (metrics that don’t have any solid impact on business outcomes). Others fail to segment their audience and end up launching generic marketing messages and campaigns that underperform in today’s era of hyper-personalization.
If you’re also finding it difficult to measure the impact of your marketing spend, adding MMM as part of your marketing strategy might just be the answer to your measurement woes. But, like any other modeling system, MMM also has its share of caveats.
Here’s an overview of the pros and cons:
MMM advantages
- Accurate, with complete coverage of digital and traditional marketing channels
- Captures the relationship between variables
- Measures both online and offline conversion outcomes
- Estimates and measures media saturation and yield levels, so marketers can pinpoint optimal investment levels
- Advanced MMM approaches provide scenario planning and budget optimization capabilities, enabling marketers to run simulations to forecast business outcomes
- Accounts for drivers that directly impact ROI
- Forfeits the use of personally identifiable information to ensure user privacy is never compromised
MMM disadvantages
- Requires a lot of historical data inputs
- Relies on a number of assumptions for non-marketing factors
- Provides infrequent reports
- Doesn’t consider the relationship between channels
- Doesn’t give any insight into brand or messaging
- Doesn’t factor in customer experience
Key takeaways
- Media mix modeling (MMM) is a statistical approach used to evaluate the impact of various marketing channels and tactics on a specific business outcome, typically sales revenue.
- MMM uses historical data to analyze the relationships between marketing inputs and outputs, allowing marketers to understand the contribution of each channel to overall performance and optimize their media mix for maximum impact.
- When deciding if MMM is right for your mobile app marketing campaign, consider your budget, data availability, complexity of the mix, campaign objective, skill and expertise, and timeframe.
- A standard MMM process has four phases: data collection, modeling, data analysis and insights, and optimization.
- MMM differs from data-driven attribution modeling, which draws on user-level data to measure how individual marketing touchpoints affect conversion.
- MMM can help marketers identify the most effective channels, measure marketing ROI, and make informed decisions about how to allocate budget to boost sales and revenue.