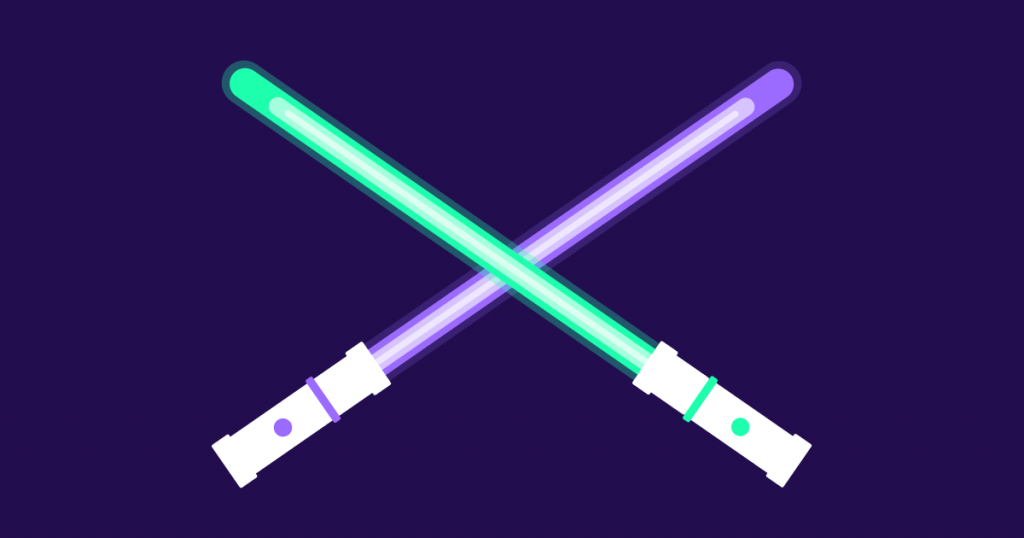
6 min read
The force of AI in ad fraud: fighting innovation with innovation
AI is both a catalyst and a solution for ad fraud. Learn how AI-driven fraud detection can help you fight sophisticated scams and protect your ad budget.