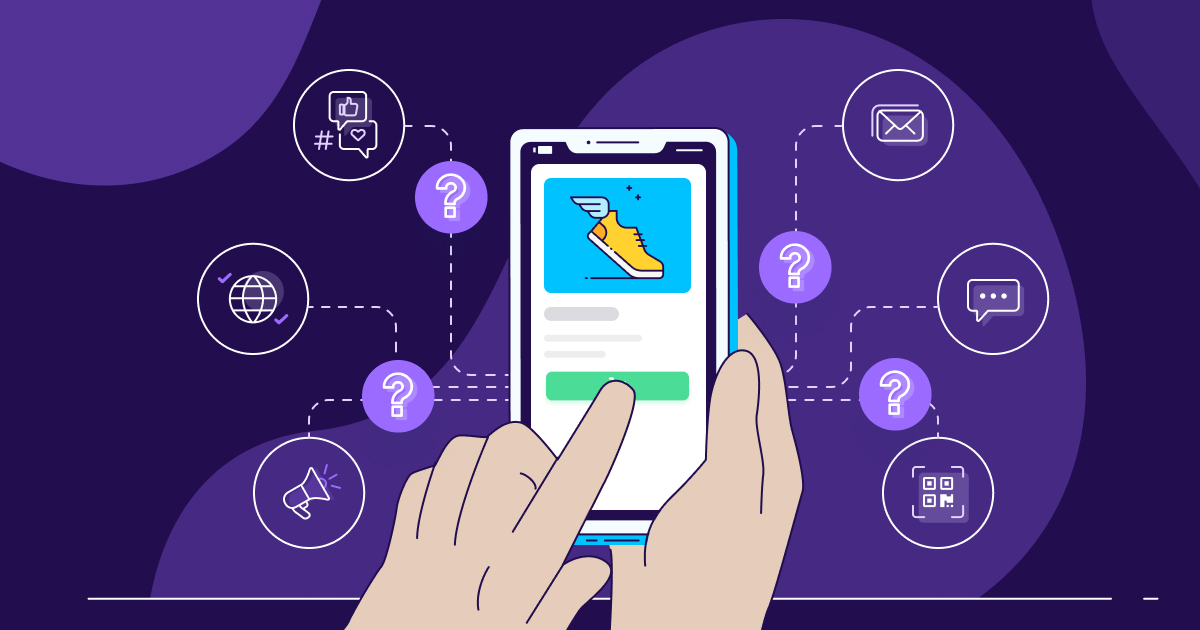
Charting raw data’s potential to navigate rough waters in the market
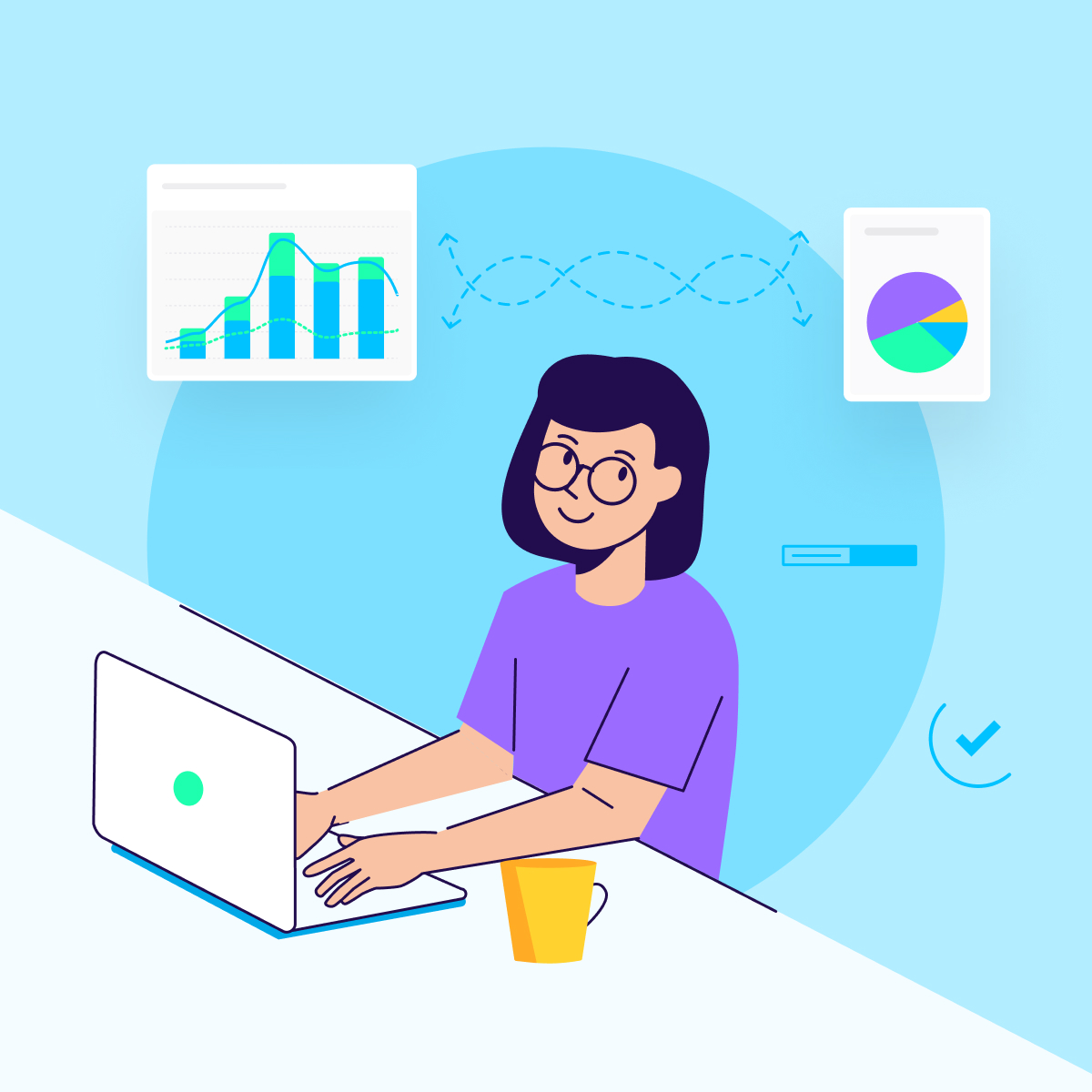
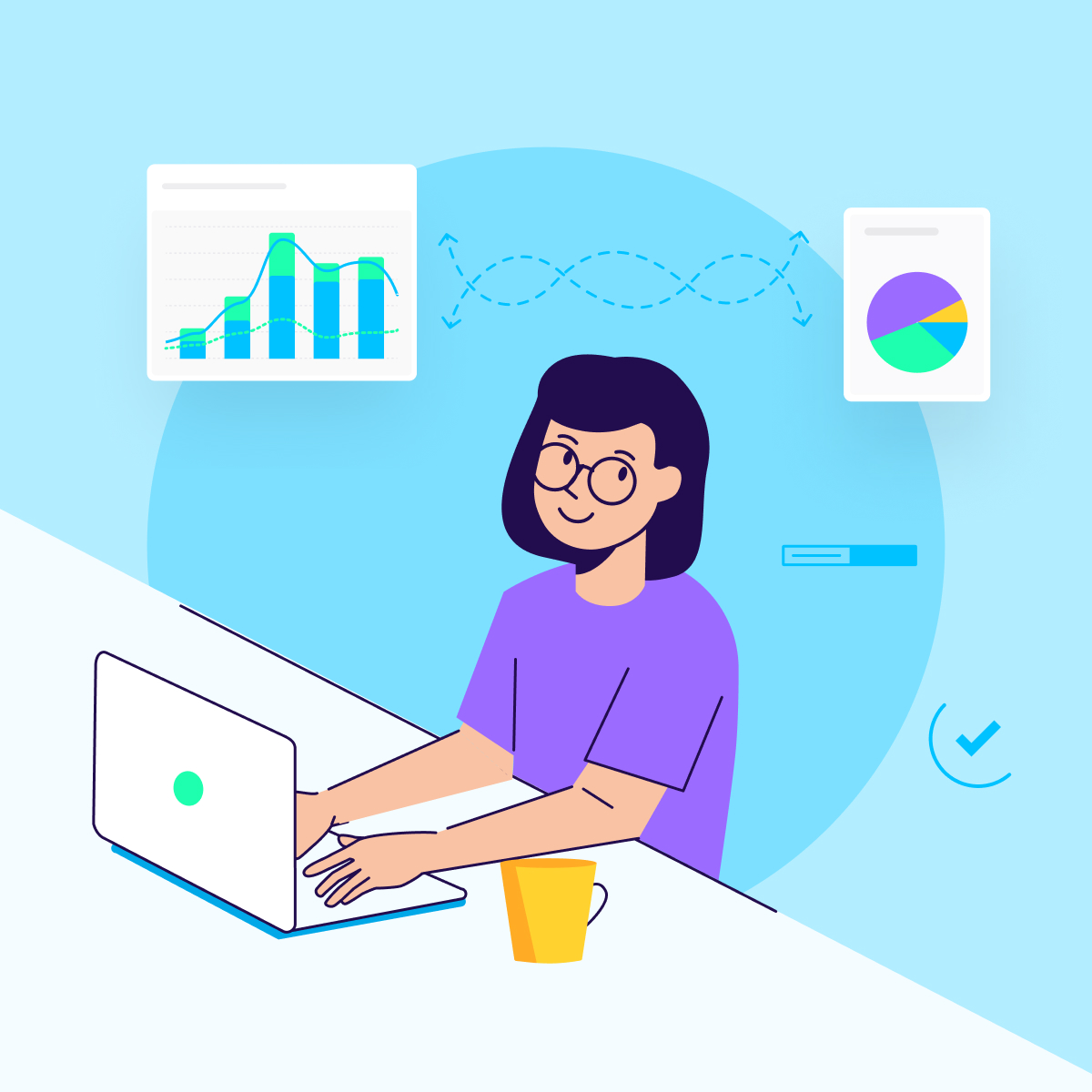
The reality of the recent COVID-19 pandemic is that we’re continuing to learn how industries are being impacted. For instance, the app marketing industry is anticipating a shift from an install-based to action-based user interaction for apps.
But within this uncertainty is the constant of raw data in marketing, which can offer app marketers a significant edge, save on costs, and help them build resilience far into the future.
Raw data, in essence, is information (app installs, in-app events, uninstalls, media sources, geo, device type, etc.) that can be exported, analyzed and stored, and in the hands of an experienced marketer it can help to supercharge a brand’s campaign and marketing performance.
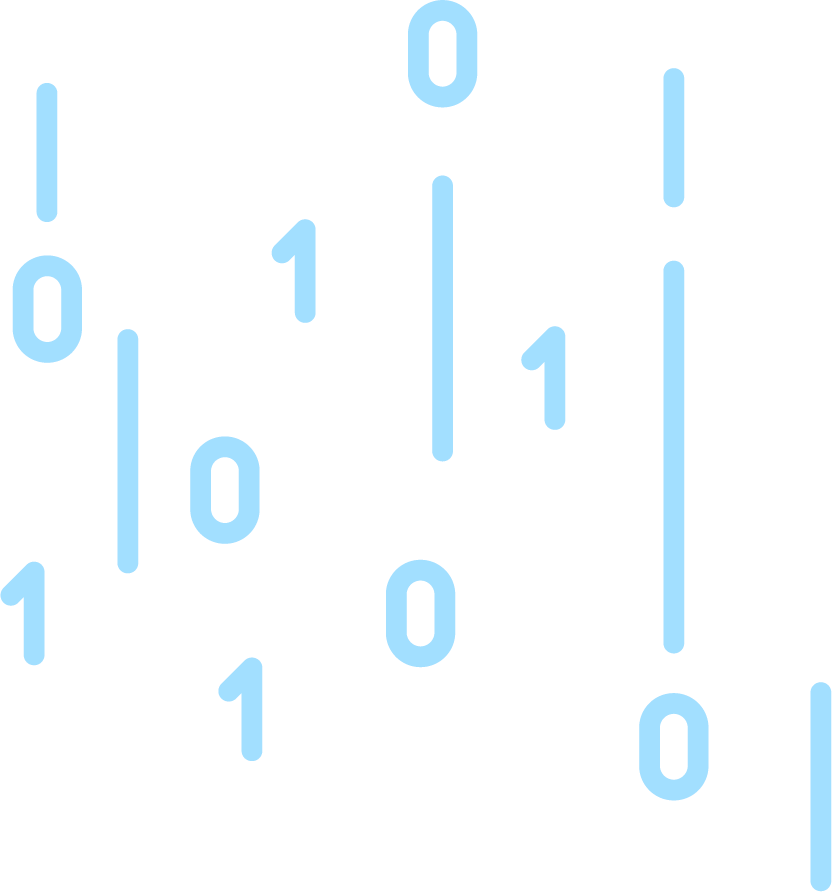
Why use raw data?
Raw data helps marketers dive deeper and get to the heart of whether their content and marketing efforts are actually working.
This is particularly relevant in a post-COVID-19 world, where raw data can help marketers better gauge how to optimize and adapt to this new marketing landscape, as we start to see a shift in how consumers are interacting with marketing efforts—specifically advertising content.
Efficiency and productivity
Raw data can also help marketers get more bang for their budget. Solutions such as AppsFlyer’s Push API can help marketers optimize their traffic in real-time. Identifying critical metrics is key to delivering more ROI as well as boosting the lifetime value of your marketing efforts.
Marketers need to be efficient, targeting their efforts at what is generating the most ROI, especially now. Push API’s filtering and targeting capabilities enable better efficiency and productivity by giving advertisers more control over which data is being sent to them, reducing the amount of ‘noise’ (irrelevant traffic and events) and making it easier for advertiser BI teams to process that data.
Control
Advertisers can define a specific endpoint for organic data and another for non-organic data – one for in-app events and another for retargeting.
Configuring multiple endpoints provides advertisers the ability to organize their data in the way they want to while controlling inbound data more efficiently
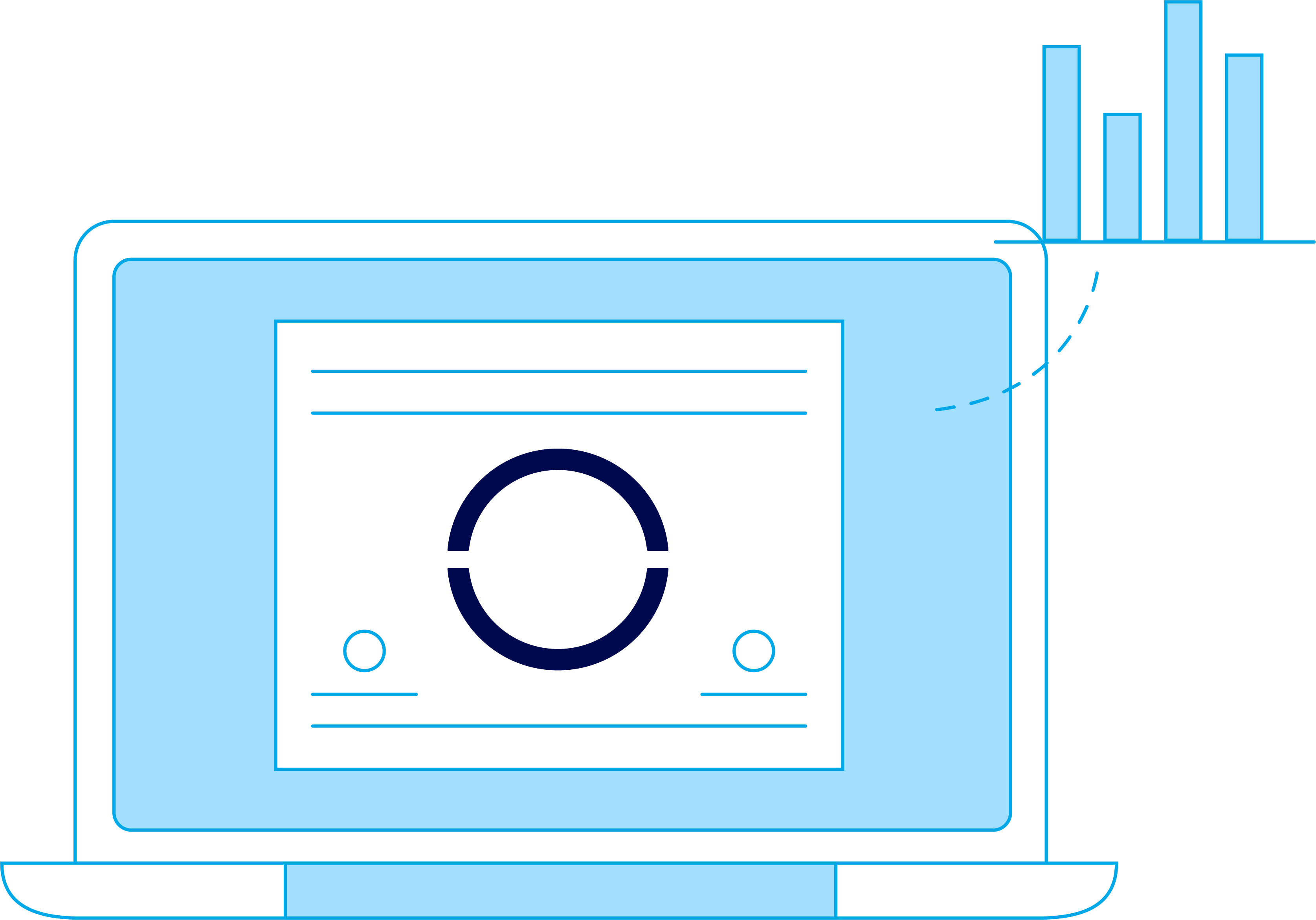
Using raw data to design effective campaigns
Raw data reports brim with valuable insights.
While there are a myriad possible uses, we’ve outlined a few common potential applications below:
User identification
One of the greatest hurdles in designing an effective campaign is determining the correct target audience.
Raw data can help marketers more accurately analyze changing user bases via device ID or customer user ID, so they can target their content with greater accuracy and efficiency. User identification data is an excellent tool for marketers to hone in on the target audience and ensure their campaign is being targeted toward the correct user base.
Understanding user behavior
Raw data, with the help of timestamps, can help marketers better understand user interactions, and get more granular visibility in measuring the customer journey.
User interaction data makes all the difference in the creation of a solid marketing strategy that helps generate the expected ROI.
Improving UX for higher growth
A better understanding of the user base and user interaction through raw data can also help app marketers improve their user experience (UX).
For example, knowing each single step of the journey can help Shopping and Travel apps optimize time to first purchase or retargeting users.
Performance-based billing
Raw data reports provide a clear and accurate picture of ROI, allowing for performance-based billing to clients for campaigns.
This cost-effective billing model isn’t new in the industry, as more and more agency/client relationships are moving towards performance-based pricing, where agencies/marketers are billed based on achieving certain client advertising and/or marketing goals.
User segmentation
You can use geolocation and device-type data to create specific user segmentation.
This type of segmentation is useful during COVID-19, when you’re concerned with targeting users in certain geographies or more engaged audiences.
Identify potential fraud
Although there’s still no hard data on the extent of fraud on mobile, the challenge is still significant and will only increase as mobile budgets continue to rise.
There are many ways to identify and fight potential fraud in mobile marketing.
Assuming you’re dealing with a savvy fraudster who can create a different identifier for the same device, you’ll be able to use the raw data to fish out suspicious patterns.
For example, you’ll be able to pinpoint how many installs or unique identifiers are from the same IP, illogical time stamps (every second, every 5 seconds etc.), or the presence of many identifiers for advertisers (IDFAs) for a single identifier for a vendor (IDFV).
Ultimately, brands will need to measure the effectiveness of their optimization efforts.
Attribution and analytics partners such as AppsFlyer offer extensive measurement solutions that affords advertisers a bird’s eye view across a number of channels, while also offering more detailed deep dives wherever necessary.
Having this continuous feedback mechanism for their use of raw data should allow marketers to make more informed decisions over the long run.
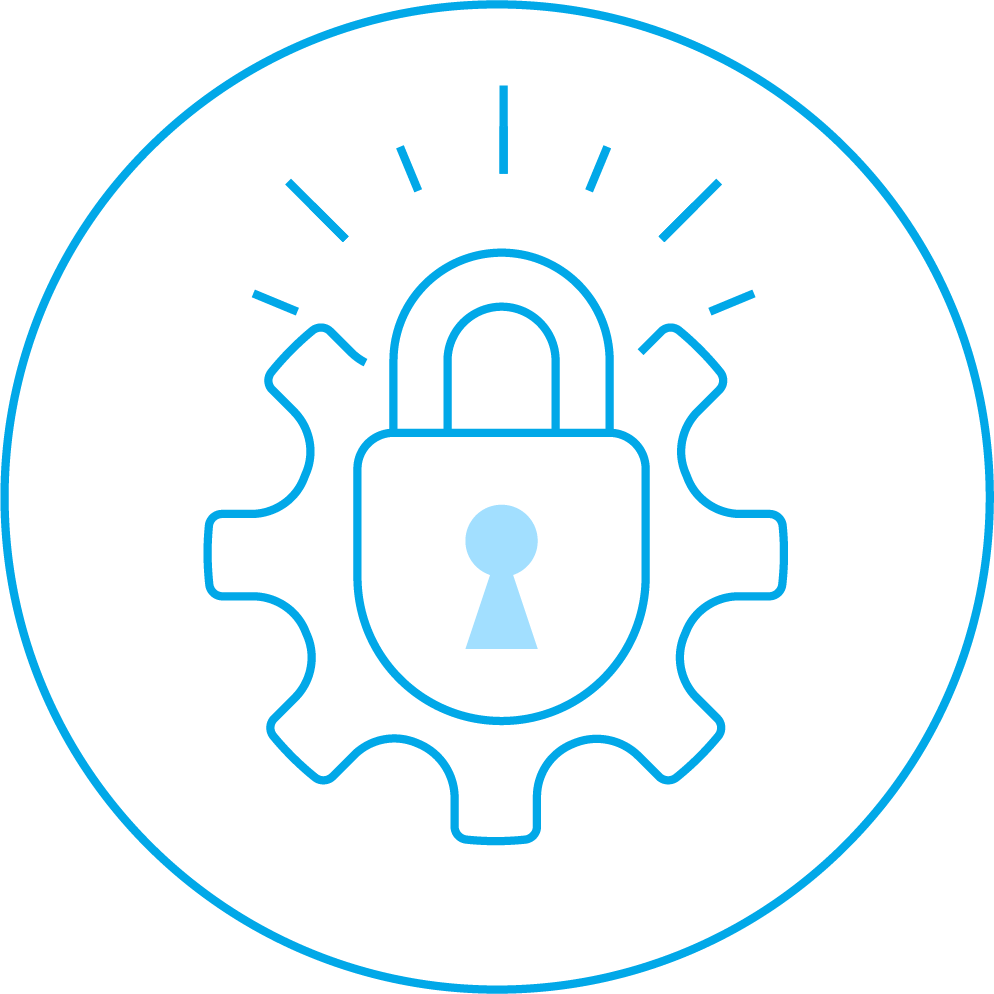
How to access raw data
Raw data should be easily accessible to all marketers. On the AppsFlyer platform, accessing a raw data report is simply downloading a CSV file (spreadsheet) of your data automatically synced to external BI systems.
A secure database of information with continuous updates on performance feedback is not only a cost-effective method to chart the evolving topography of advertising, but it’s also a great way to customize reports.
Safety and analysis: Why store raw data now and how to do it?
While it can be convenient to have continuous access to raw data, losing your connection to your attribution provider may cause your data to be lost.
Marketers therefore prefer the option of storing their data to prevent loss and allow for retrieval for later analysis.
- Better management: Making the most of storage and BI infrastructure
- Accessible repository: Having real-time access to raw data
Storing raw data can be complicated, particularly when you need to make sure user privacy protections are not infringed upon while maintaining your data security. Businesses have a fiduciary duty to safeguard their user’s data and ensure that it does not go to a third party.
AppsFlyer’s toolset allows users to store every data point sent to AppsFlyer, including organic data, full attribution and engagement data, session data, click data.
All of this mobile attribution and marketing data is stored on a secure storage locker hosted on Amazon S3, allowing users to back up their AppsFlyer data to the Amazon Cloud. Advertisers can also maintain their own data retention policy when the data is stored by them
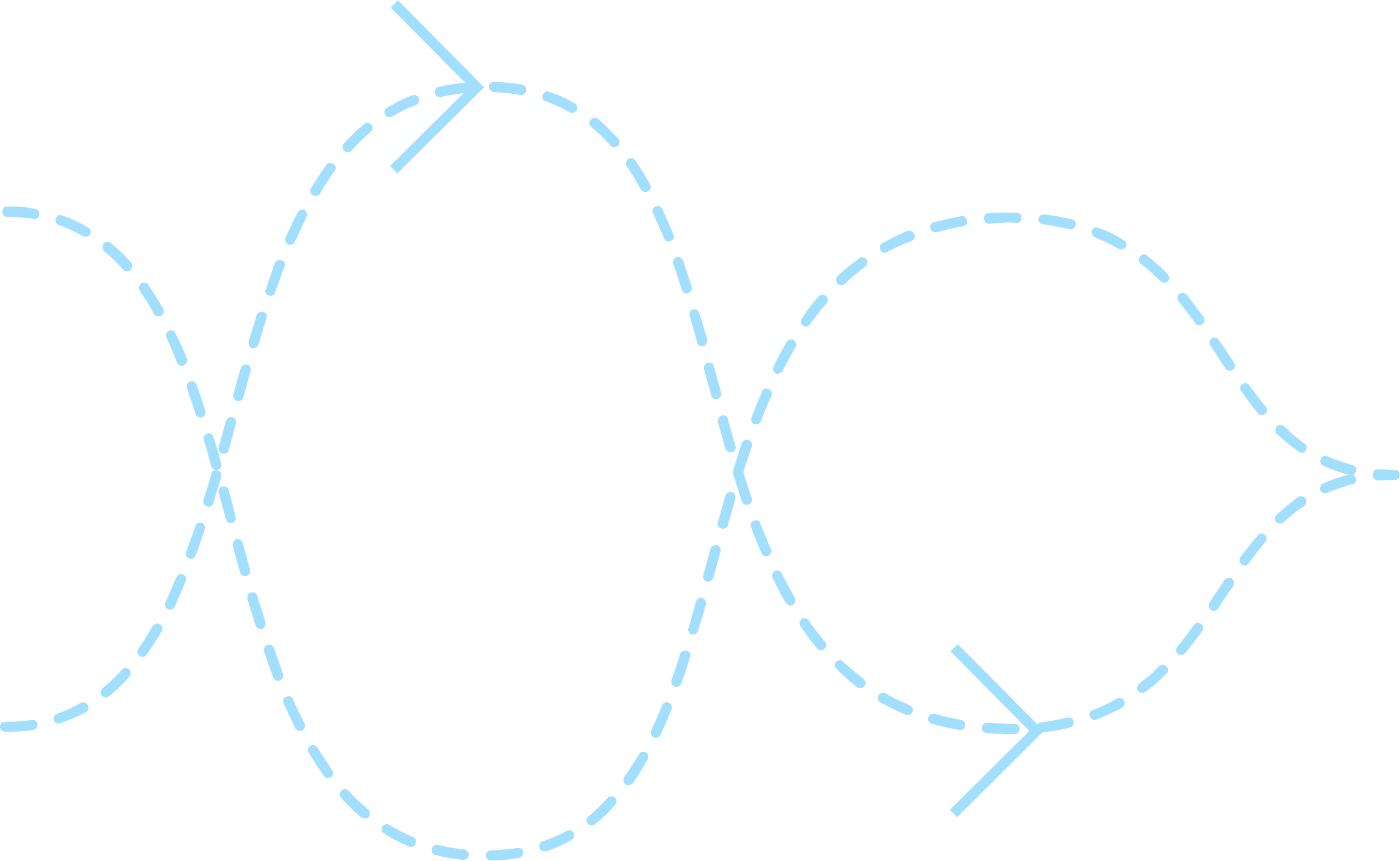
Building resilience
Our external environment is teeming with data that can be used to serve any number of objectives.
Yet the potential of raw data to allow marketers to draw any number of actionable insights has been made even more compelling during the COVID-19 pandemic, a time when the focus has turned towards squeezing more value out of every dollar. Now may be an ideal time to learn how to correctly wield this tool.
But what’s perhaps more important is the significance of using raw data in the long term.
As demand recovers and budgets begin to stabilize, raw data should give marketers and brands an extra toolset to optimize campaigns far into the future.
Raw data solutions
Of course, there is no one-size-fits-all approach to raw data, and different measures are called for depending on business needs and budget. And because everyone should have access to their own data, AppsFlyer offers solutions that allow businesses to export raw data for feeding into their BI platforms or for them to use it any way they see fit.
- Push API V2.0 Push API sends attribution event messages in real-time to your server-side endpoints. Doing so enables you to follow user journeys via multiple environments and touch points.
- Pull API is a raw data service by AppsFlyer that allows for downloading CSV reports using uniform resource identifiers (URIs). To set up the Pull API feature, all a user has to do is send the URI to AppsFlyer by pasting it in the browser address bar, or by using scripts. Data Export Tab functionality can also allow you to easily download CSV reports from your dashboard.
- Data Locker stores every single data point sent to AppsFlyer; including organic data, full attribution and engagement data, session data, click data and more. In a way, it’s the ultimate mobile marketing data sync. While credible mobile attribution and engagement data is incredibly powerful, mobile data is too often stuck in the dashboards of each individual provider. Data Locker from AppsFlyer provides 24/7 access to all of the user’s mobile attribution and marketing data via a secure storage locker hosted on Amazon S3. The full scope of your AppsFlyer data is synced to the Data Locker, so the user can focus on insights and marketing optimization.