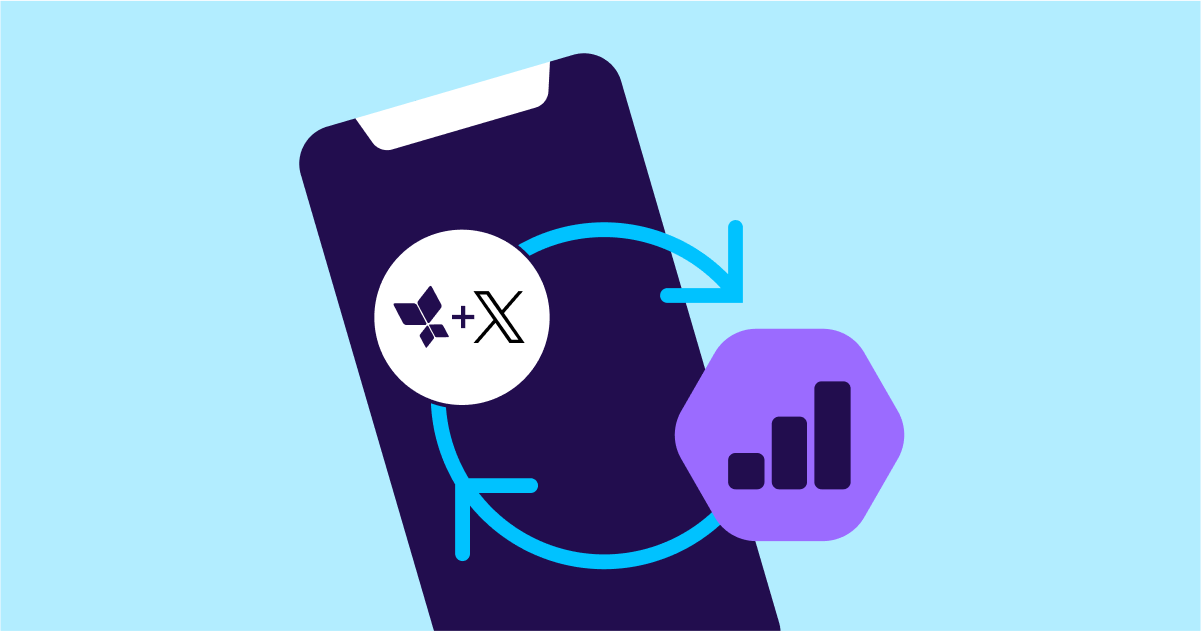
Testing a multi-billion dollar black box
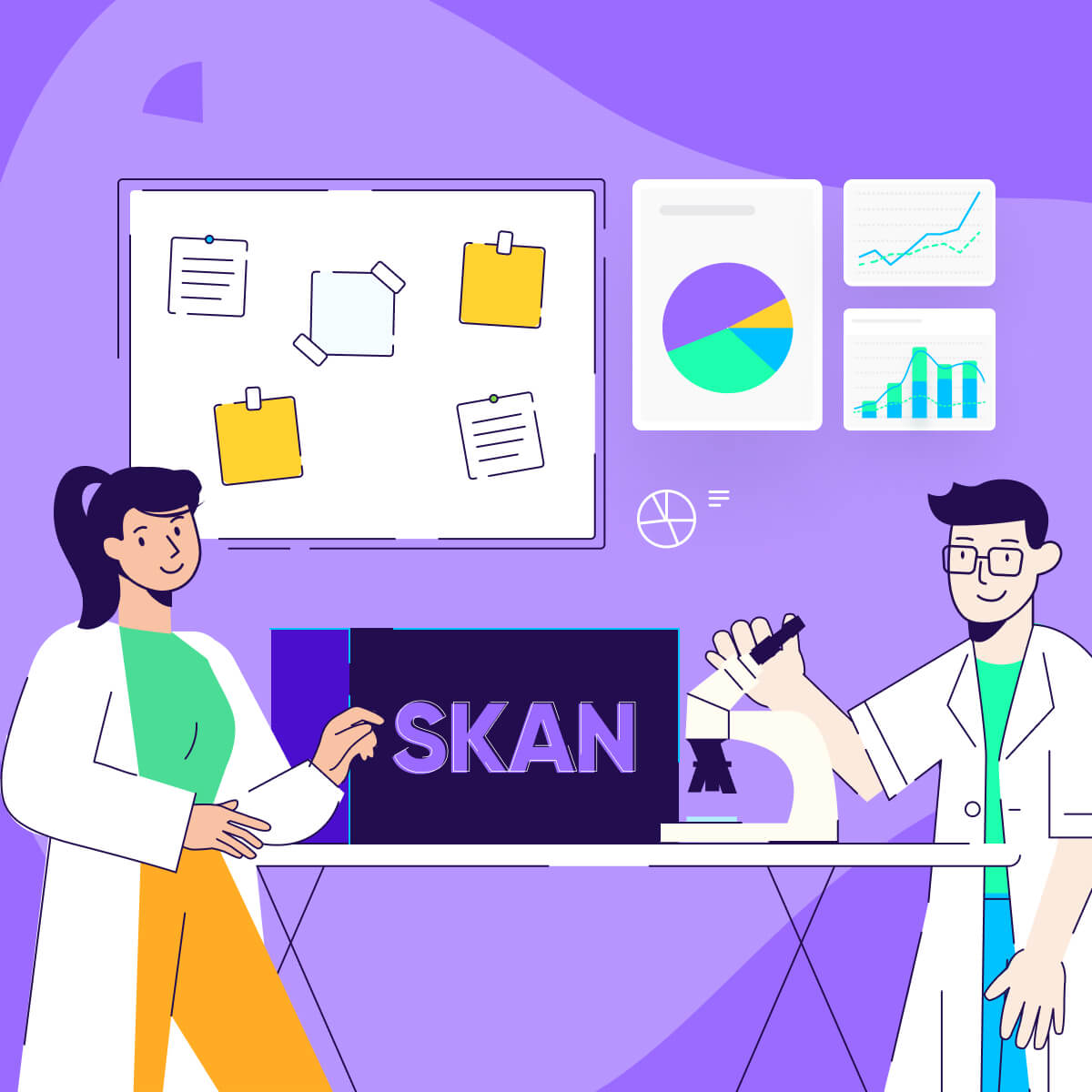
When Apple announced iOS 14 with all of its many changes 2 years ago, the reintroduction of SKAdNetwork as an attribution method for iOS shook the advertising realm.
What was perhaps most shocking (and has never really been addressed), is that no one in the ecosystem really challenged it. And due to the nature of SKAN, there is no way to verify that it is indeed working properly.
While Apple is one of the best parties an advertiser can put their trust in, when it comes to billions of dollars worth of advertising budgets, the industry wanted to be extra sure. Many parties, AppsFlyer included, pointed out where SKAN was still lacking and needed improvement, but no one asked flat out, “does it even work?”
As an attribution provider, data integrity and accuracy is our commitment to our customers. Reliance on a third party data source is not something we take lightly.
Advertisers have continued to spend millions of dollars on iOS campaigns. Some advertisers have made changes to their marketing strategy, but all in all, the world of iOS advertising has kept turning. In fact, in 2022 ID matching rates rose 10%, reaching 26% of all non-organic installs in 2022 (SKAN included). In addition, SKAN install rates surged 3.5X from June 2021 to January 2022, and have grown an additional 10% since then.
Happily, SKAdNetwork has evolved somewhat and continues to grow closer to the attribution solution app developers deserve. As we embark on the journey into SKAN 4.0, maybe it’s time to finally ask: Even when considering the many limitations SKAN has, how accurate is SKAN attribution?
Spoiler: The bottom line is – when it comes to install attribution, SKAN works as expected. SKAN is a reliable, accurate attribution method for simple user acquisition flows. Let’s be clear: SKAdNetwork, even the improvements that come with v4.0, is still lacking. It doesn’t even begin to be a full-coverage iOS mobile attribution solution. However, advertisers can rest assured that in the very least, it does what it says it does: provides accurate click-to-install attribution for user acquisition campaigns in iOS.
Dissipating a piece of the black box
The ins and outs of SKAN aren’t limited to install attribution, and many pieces of the SKAN puzzle are still untested and not transparent to the industry. These include (but are not limited to): how reinstalls are measured vs. re-attributions, attribution logic in cases of uninstalls, indirect click-to-download flows, lookback window logic, cross-device attribution.
However, tackling the main use case that SKAN claims to cover, direct click-to-install flows, is something we can test. No one said this would be simple.
There are several complexities in place that make data integrity validation harder to do in the new iOS reality:
1. App-to-app flow
SKAN v3.0 or below only applies to app-to-app flows, meaning a user would have to see an ad in an app, click on the ad, and then download the advertised app. In order to test how well this flow is attributed by Apple, we’d need two apps (the one where the ad was viewed and the one downloaded), a network , and complete control over the ads shown.
2. ID matching
The most accurate way to test data integrity is to compare the number of installs reported by Apple with the number of app launches successfully detected by AppsFlyer. In order to do this deterministically, ID matching is necessary. In iOS 14.5+, IDFAs are not shared by default; however, when a user journey goes from one app to the other and both are owned by the same vendor, the match can be done using IDFV.
3. SKAN postbacks
SKAN postbacks are sent to the ad network, and a copy can be sent to the advertiser if they predefine an endpoint to receive them (available in iOS 15 or later). In order to make sure that no postback was lost along the way we verified both postbacks types. Postbacks are also sent at a delay post-install, meaning that the data can take time to trickle in and take longer to validate.
Methodology
Goal:
Compare the number of Apple SKAN postbacks to the number of installs we measured through ID matching attribution.
Setup:
We created an iOS campaign flow using our own testing ad network, publisher app, and target app (app to be downloaded).
Method:
We launched a crowd testing campaign in September 2022, asking people with iOS devices to download our publisher app, click on an in-app ad and then download the target app. All in all, 380 people showed interest in participating and 203 completed the flow within 10 days. This gave us a large enough cohort size for testing.
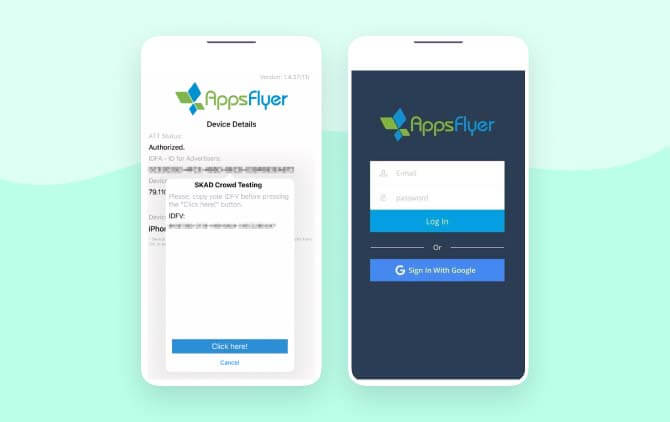
Right: Advertised app
Validation:
Since SKAN attribution is a 3rd party data source, validation of the results was critical. As the attribution provider, app owner and the ad network, we were able to compare the number of SKAN postbacks received from Apple reporting SKAdNetwork attribution, to the number of installs measured via AppsFlyer ID matching attribution.
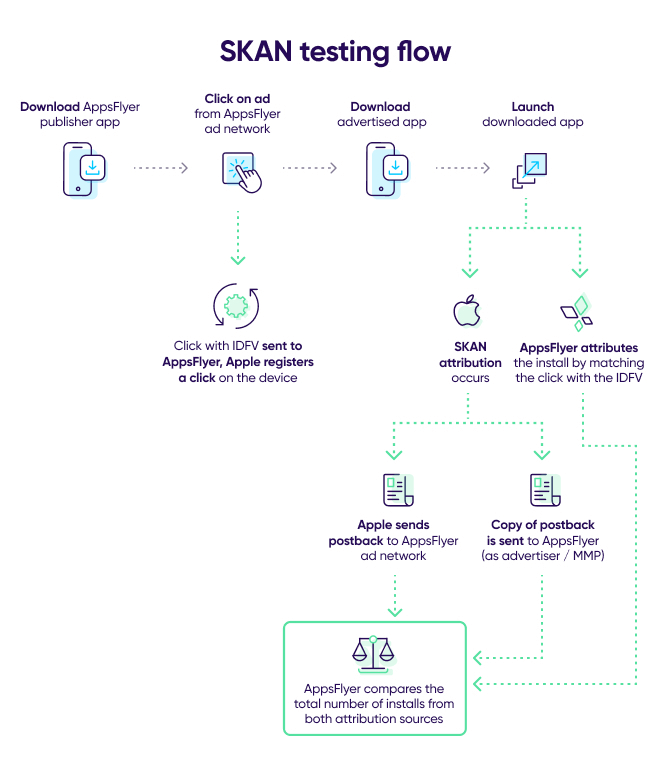
Results:
There was a 100% match between the SKAN postbacks received from Apple and the attribution successfully carried out by AppsFlyer via ID matching. In other words, SKAdNetwork attribution was accurate and successful in 100% of the cases.
Pay for attribution data confidence
As a customer-obsessed service provider, it was critical to us to ensure that our customers can be confident about the integrity of their data.
With data visibility continuously decreasing in the new privacy era, advertisers need to be able to make decisions confidently, and this starts with confidence in their MMP.
As we move toward an even more complex future, where data sources will be obfuscated or partial, AppsFlyer will continue to test and ensure that advertisers can remain confident in their data sources, while safeguarding their end users’ privacy.