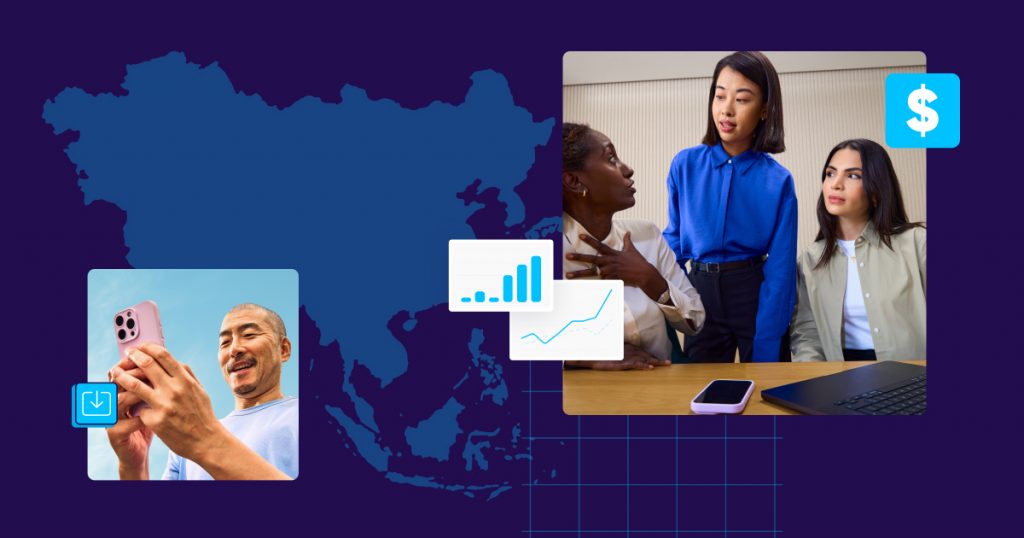
If you’re a marketer, it’s unlikely you’ve managed to avoid a conversation in the past few months where “Data Clean Room“ was not brought up at least once, and usually in an excited yet slightly confused tone.
What is this strange, hygienic chamber of data everyone’s talking about?
Some refer to data clean rooms as “the Switzerland of data”, and rightfully so, because it offers a neutral, safe space for 1st-party user data to be leveraged collaboratively. In a data clean room environment, two parties can securely share and analyze data with full control of how, where, and when that data can be used.
In this way, brands are given access to much-needed data, but in a regulatory compliant space that doesn’t violate consumers’ privacy. While user level data goes into the data clean room, aggregated insights come out in a co-mingled audience group called a cohort.
So, to get you well equipped for 2022, we’re going to take you on a journey through thick forests of unknowns and deep lakes of 1st-party data, in a guide entirely dedicated to the topic of data clean rooms.
By the end of which you’re going to know all about what they are, how they work, why marketers need them, and how they’re going to dramatically affect our ability to measure campaigns in the years to come.
But before we do, let’s begin with the story that actually led us all to this point.
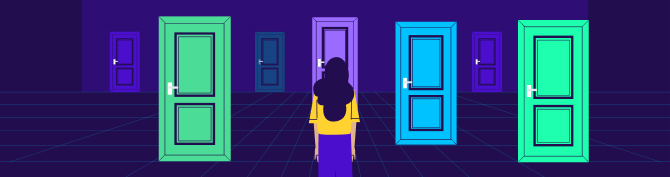
What are data clean rooms?
It’s evolution, baby
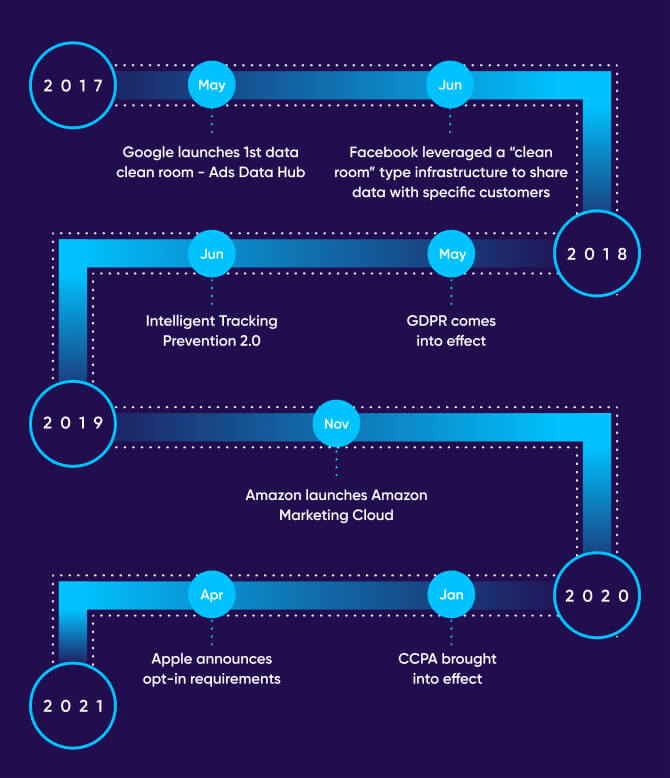
Despite its resurgence in the past year, data clean rooms as an infrastructural concept have actually been around for a few years now.
Google was not the first to coin the term, but it was the first company to commercialize a data clean room solution, launching its Ads Data Hub in 2017. The goal was to create a secure and private environment for enriching their 1st-party data (from CRMs, CDPs, event logs, etc.) with user level data contained within Google’s ecosystem, after which it could be leveraged for Google campaigns.
A mere month later, Facebook announced its own data clean room offering for the purpose of sharing data with its customers. A coincidence? Probably not.
But it was 2018 that truly set off the starter pistol of the user privacy era, with legislation such as the GDPR and Apple’s Intelligent Tracking Prevention 2.0 becoming the new privacy sheriffs in town.
Following suit in 2019, Amazon launched a data clean room platform titled Amazon Marketing Cloud, the CCPA was brought into effect in early 2020, and in April 2020 – the entire mobile app ecosystem gasped as Apple dropped its opt-in mechanism bomb in iOS 14 – aka the ATT.
Amounting user privacy laws and stricter data privacy standards have transformed the way advertisers and brands can collect and share consumer data.
Facebook announced in October of 2021 that it will no longer send user level campaign data to advertisers, but to Mobile Measurement Partners (MMPs) only, with other networks expected to join the party soon.
Between Apple’s game-changing ATT framework, Facebook’s user level data decision, and the upcoming demise of Google’s 3rd-party cookies in 2023, the scale and breadth of data sharing is becoming increasingly limited, making campaign measurement and optimization more challenging than ever before.
So, brands are now scrambling to find new ways to gain meaningful marketing insights in a privacy-compliant way.
Kicking off the data exchange alliance trend in 2019, Disney began collaborating with Target, Unilever joined forces with Facebook, Google and Twitter to create a cross-channel measurement mode, ITV entered a partnership with Infosum in 2020, and in 2021, TransUnion launched its data collaboration with BlockGraph.
The binding element that enabled all these bountiful data collaborations that are only expected to increase? Why, Data Clean Rooms, of course.
What is a data clean room anyway?
Data clean rooms allow marketers to harness the power of the combined data set while adhering to privacy regulations. Personally identifying information (PII) or attribution restricted data of individual users is not exposed to any of the involved contributors, which makes it impossible for them to single out users with unique identifiers.
PII and user level data are processed so that it can be made available for a variety of measurement purposes, producing anonymized data that can then be cross-referenced and combined with data from different sources.
In most cases, the only outputs from the data clean room are aggregate level insights, e.g. users (plural!) who have performed action X should be offered Y. That being said, user level output can take place given the full consent of all involved parties.
The key ingredient that makes data clean rooms a highly credible platform is the fact that access, availability, and usage of data are agreed upon by all data clean room parties, while data governance is enforced by the trusted data clean room provider.
This framework ensures that one party can’t access the other’s data, which upholds the ground rule stating that individual or user level data can’t be shared between different companies without consent.
Let’s say a brand wants to share insights with Target. To facilitate that, each party needs to place its user level data into a data clean room – to see what the other already knows about audiences they have in common, e.g. reach and frequency, audience overlap, cross platform planning and distribution, purchasing behavior, and demographics.
Data clean rooms can also be used as an intermediary tool for measuring campaign performance. Instead of guesstimating audience insights, brands can actually look under Amazon or Google’s 1st-party data hood, all while being completely privacy-abiding.
In return, advertisers can get an aggregated output without individual identifiers, including segmentation and look-alike audiences, which can then be shared with a publisher, a DSP, or an ad network to inform a campaign. Alternatively, if you’re a retailer with an ad network, for example, you will be able to leverage this output when buying ads.
Making sense of it all – How does a Data Clean Room work?
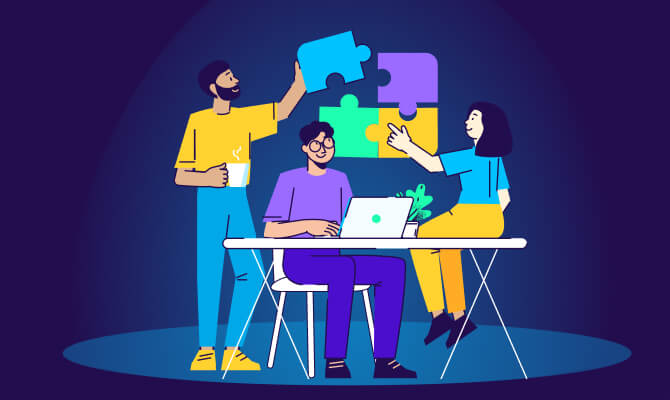
A data clean room operation involves four parts:
1 – Data ingestion
In the very beginning, 1st-party data (from CRMs, site/app, attribution, etc.) or 2nd-party data from collaborating parties (i.e. brands, partners, ad networks, publishers) is funneled into the data clean room.
2 – Connection and enrichment
Data sets are then matched at the user level, and are made to complement one another using tools such as 3rd-party data enrichment.
3 – Analytics
At this stage, the data is analyzed for:
- Intersections or overlaps
- Measurement and attribution
- Propensity scoring
4 – Marketing applications
At the very end of the data clean room journey, aggregated data outputs enable marketers to:
- Build more relevant audiences
- Optimize their customer experience and A/B testing
- Execute cross platform planning and attribution
- Perform reach and frequency measurement
- Run deeper campaign analysis
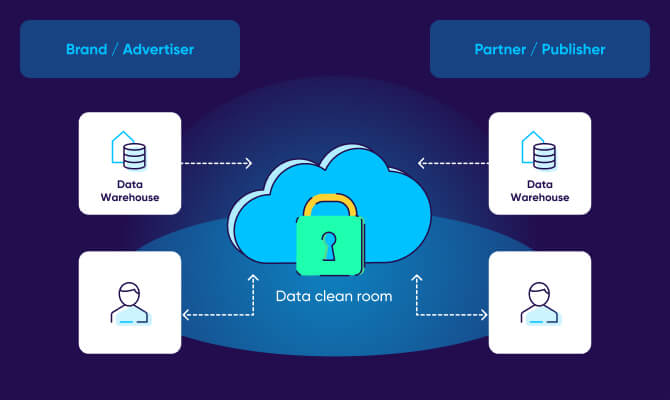
Now that we’ve covered the how, what about how the data is actually matched?
When working with a data clean room, identifiers such as email, address, name, or mobile ID are similar on both the advertiser and publisher side, which enables successful matching of both data sources.
If such identifiers do not exist, advanced tools such as machine learning and probabilistic modeling could be applied to enhance matching capabilities.
Why do marketers need a data clean room?
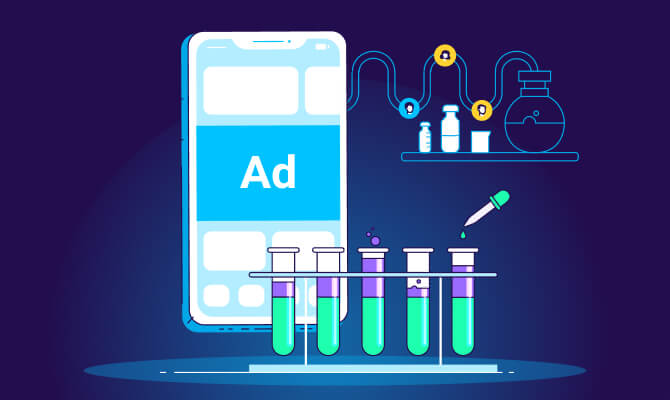
First and foremost – rising scrutiny around data privacy.
Driven by privacy regulations and walled garden privacy initiatives (more on that in a bit), it’s becoming increasingly complex for advertisers and publishers to collect, store, analyze, and share data.
Second reason would be lack of commercial trust between parties. As we all know well, handing over valuable 1st-party data outside of a data clean room is risky from both a legal and commercial perspective.
Lastly, inefficient data synthesis processes, where data correlation across separate data sets requires heavy lifting by data scientists, which is a costly and time-consuming endeavor.
Data clean rooms to the rescue!
When it comes to data privacy, all parties within a data clean room maintain full control over their data, which is usually fully encrypted throughout the process. A data clean room includes strict governance and permissions, where each party defines what and how their data is accessed and put to use.
Another important aspect that addresses the challenges mentioned above is differential privacy, which makes it impossible to tie back a specific impression, click or activity to a specific user.
Last but certainly not least, data clean rooms offer privacy-centric computing, querying, and aggregated reporting fit for purpose integrations so data sets can be stitched together.
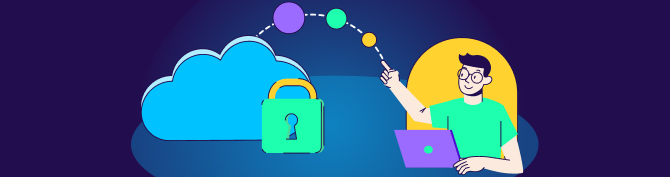
A comparative overview of a new(ish) market
User-level data used to be what mobile marketers relied on wholeheartedly. In recent years, however, the surge in privacy-centric regulation and the fact that this data was rendered more elusive than a snow leopard – means that advertisers are now struggling to make data-driven decisions.
And if you thought this is just a phase, well – think again. These ecosystem-sweeping changes are only predicted to accelerate, which would further restrict access to this data, making business optimization even more challenging than it already is.
But this is not a sad story, and these changes could very much serve as a valuable opportunity for brands to cultivate their competitive edge. Forrester articulated it well when they stated that “ethical privacy practices will be the next consumer-driven, values-based source of differentiation.”
Data clean rooms stem from this very consumer privacy-centric mindset. And driven by the need for privacy compliance and cross-media measurement and optimization, data clean rooms are becoming an essential tool in marketers’ tech stacks.
According to Gartner, 80% of advertisers with substantial media budgets will utilize data clean rooms by 2023, estimating that there are currently between 250 to 500 data clean room deployments that are either active or in various development stages.
What kind of data clean room creatures are out there, you ask? Let’s break it down.
Types of data clean rooms – Introducing the cast and crew
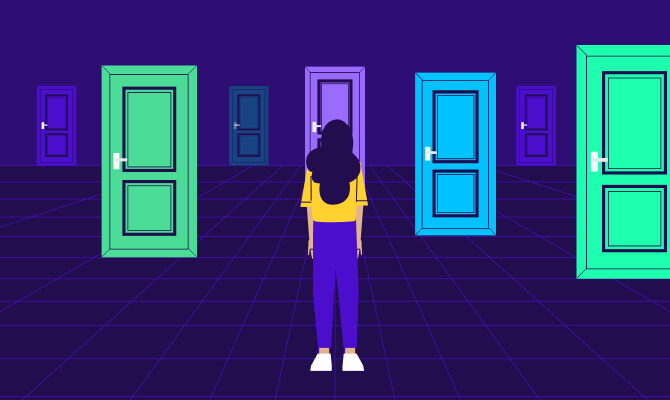
All data clean rooms help to hide consumers in a crowd by de-identifying their user-level data and clustering them based on common attributes. But in what ways do they differ from each other?
To help you make sense of the rapidly developing data clean room landscape, let’s break down the actual breeds out there, assess the relative performance of each across the value chain, and examine their unique pros and cons:
Walled Gardens – Big Tech platforms
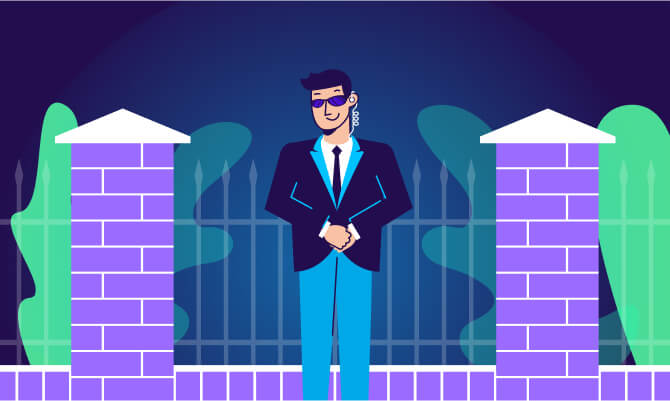
This group consists of closed ecosystems where the tech provider has significant control over the hardware, applications, or content.
Walled gardens were first introduced by Google, Amazon, and Meta (Facebook) to safely commercialize their 1st-party data, and also capture ad spend from rivals while they’re at it.
Needless to say, nearly 70% of all ad media spend sits with these three giants — each of which allows advertisers to work within their walled garden data clean rooms: Google Ads Data Hub (ADH), Facebook Advanced Analytics (FAA), and Amazon Marketing Cloud (AMC).
These security-stringent environments are where the mega SRNs make the event-level data accessible for marketers to be able to make informed campaign decisions, without jeopardizing consumer privacy or the ecosystems’ defense moats.
Pros
- Supporting 1st-party data set enrichment with event-level data
Cons
- Offering raw materials for analysis – making this data readable to the common marketer will require a team of data scientists, analysts, and engineers
- Rigid architecture
- Lack of cross platform ability to generate actionable data (i.e. multi-touch attribution)
- Lack of intercompany data collaboration
- Strict query functionality
Multi platform or neutral players
This type of data clean rooms consists of two sub-groups, each with their unique set of strengths and drawbacks:
Diversified
These are primarily legacy businesses operating in adjacent industries like marketing applications or cloud data storage, offering data collaboration mechanisms for gathering signals in a regulatory compliant way. This group includes providers such as Epsilon, Measured, BlueConic, and Merkle.
Pros
- Architectural flexibility
- Bespoke governance controls over type of data and level of analysis
Cons
- Limited access to walled garden data
- Narrow partner ecosystem
- Limited downstream integrations
- Leverages existing Customer Data Platform (CDP) / Complex Event Processing (CEP) functionality, which could lead to potential data issues
Pure players
These are your young, small- to mid-scale data clean room providers, among which are Habu, Harbr, InfoSum, and Decentriq, as well as more enterprise-focused tools such as SnowFlake.
Pros
- Architectural flexibility
- Leverages existing data piping and storage infrastructure (SnowFlake)
- Access to an ecosystem of integrated partners (SnowFlake)
Cons
- Limited 1st-party data granularity
- Often relies on 3rd-party infrastructure for data ingestion
- Narrow pool of downstream integration options
Mobile Measurement Partners (MMPs)
Ideally, an MMP is a trusted and unbiased player that enables all available user-level data to be leveraged using customers’ own business logic, and then consumed via aggregated and actionable insights.
Pros
- Cornered resource – user-level and cross channel data granularity
- Real-time conversion data
- Comprehensive analytics built for mobile apps’ business logic
- Flexible integration options
- Top-quality aggregated reporting
Cons
- Some limitations around data granularity and query-related actions could be imposed by SRNs
- Lack of existing CDP architecture
To assess the best data clean room provider for you, be sure to factor in your main channel (mobile, app, or web), business size, marketing needs, data structure, and internal resources.
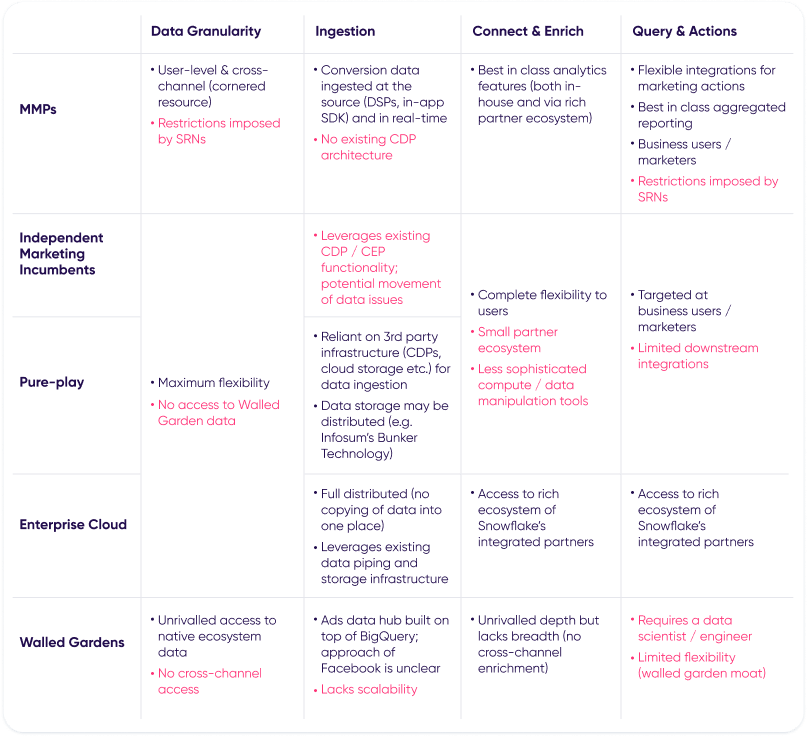
Where is the market heading?
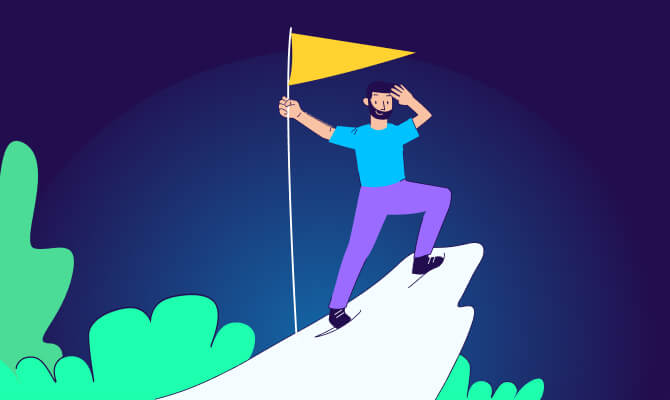
1st-party data collection has already become a highly strategic mission, and this trajectory will continue to pick up speed in the years to come. Driven by this trend, the growing interest in privacy-preserving data collaboration beyond walled gardens has resulted in a proliferation of neutral data clean room providers.
In fact, Gartner predicts that 80% of marketers with media budgets in excess of $1B will adopt data clean rooms by 2023.
This is good news for our entire data-starving ecosystem, because the more diverse the options are, the easier it would be for businesses to adopt the most suitable data clean room platform for their unique needs.
And the more businesses collaborate over regulated intermediary data grounds such as data clean rooms, the easier it would be for marketers to measure, attribute, and optimize their campaigns.
Let us show you to your room – How to choose the right data clean room for your business?
Advertisers who spend meaningful dollars on data ecosystems – need to make a data clean room investment now. But whether you’re implementing a brand new data clean room or looking to ramp up an existing one – how do you make an informed decision on the best-fit solution for your business?
To help you decide, let’s shed more light on the competitive landscape of data clean rooms, where two main factors are considered:
- The volume and quality of the data – referred to as depth
- And the variety of received data – referred to as breadth
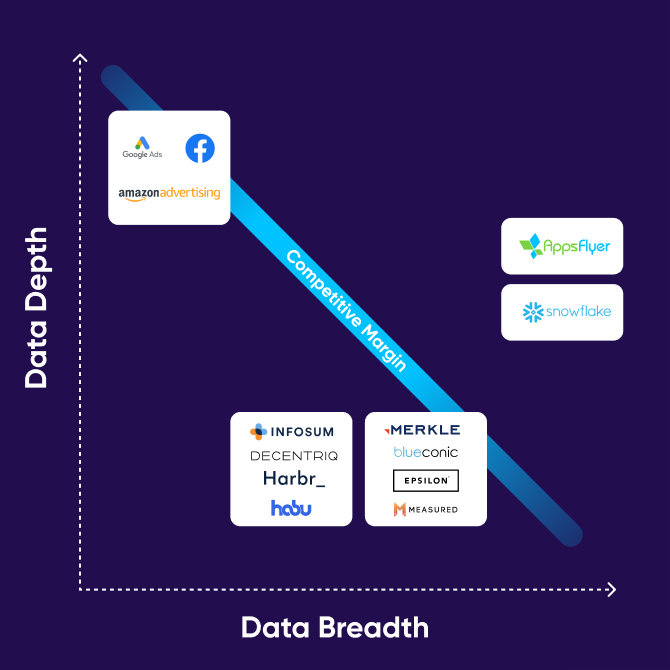
The walled garden group has the advantage of data depth – but lacks variety. The pure-play group usually offers the data clean room technology alone with very little data depth or breadth. And then there are your MMPs – providing both the data clean room technology, depth and breadth of data, and a variety of partner integrations.
When considering a data clean room, keep in mind there are several best practices you can follow to ensure you get the most value possible:
- First, be sure to factor in your main channel (be it mobile, app, or web), business size, marketing needs, data structure, and internal resources.
- Then, begin designing your data clean room with your consumers in mind. Not just for the present, but for the future. The best data clean rooms are set up to anticipate shifts in consumer behavior.
- Finally, start testing with a live audience. Analyzing consumer behavior in real time and getting actionable insights is nothing short of invaluable.
Here’s a head scratcher for you – Why haven’t data clean rooms been more widely adopted (yet)?
- Let’s get this one out of the way, folks – data clean rooms aren’t cheap. The mega-sized walled garden providers offer alternatives, but the logistical and operational hurdles of working with these platforms can put a strain on all parties.
- The success of data clean rooms is rooted in data being shared, and not all advertisers are quick to divulge detailed transactional data, mainly due to the misconception of potential privacy risks. And when half-baked data goes in – half-baked data comes out, resulting in rough measurement at best.
- Universal standards for implementation are yet to be determined. That means that pooling data that exists in multiple formats and the prep work that goes into aggregating it – could be time intensive.
- Lastly, we need to remember that user-level data is still available in some instances (e.g. Android devices and consenting iOS users), which could alleviate at least some of the urgency to implement a data clean room solution.
Can these hurdles be overcome given the right technology partner, resources, and data preparation? Of course. But more on that in our next chapter.
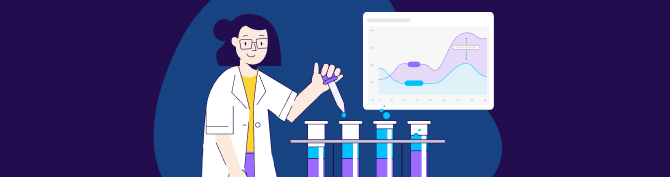
The practicality of Data Clean Rooms – Harnessing everyday use cases to fire up campaign measurement
By now we know that Data Clean Rooms offer advertisers and publishers secure, closed-loop measurement that is fully privacy-compliant.
But in which instances should you put it to use? Which scenarios could benefit from analysis in a Data Clean Room environment?
Buckle up, people. Because in this section we’re going to learn how Data Clean Rooms empower marketers to:
- Build more relevant audiences
- Continuously improve their customer experience
- Fuel cross platform planning and attribution
- Optimize reach and frequency measurement
- Perform deeper campaign analysis
So, let’s get practical.
1 – Performance measurement
Keeping track of retention, ARPU, LTV, and ROAS are flagged as key use cases for Data Clean Rooms, and rightfully so. A Data Clean Room offers a neutral environment to analyze both the advertiser’s CRM data and the ad exposure data provided by the relevant marketing partners.
In this use case, advertisers can upload their 1st-party data into a Data Clean Room following a campaign, match up identical key identifiers, and conduct analysis across their customer data and the ad exposure data made available by the Data Clean Room provider.
Let’s say you’d like to compare your recent purchase data against Google’s ad exposure data. Google’s walled garden data clean rooms — Ads Data Hub — will allow you to attribute the percentage of new customers to the marketing activity that took place across Google’s advertising channels.
If you’re in eCommerce, simply feed the Data Clean Room with your CRM data, unique identifiers (emails, postal addresses, mobile IDs etc.), and purchase date. Then, each media owner will include their ad exposure data and unique identifiers used to create the campaign audience.
At this point, you’ll be able to accurately measure the intersection between new customers and those exposed to the campaign across each media avenue, and then determine what percentage of new customers can be attributed to each channel.
2 – Building more granular audiences
After Apple dropped its ATT bomb, which dramatically hampered access to user-level data — granularity became marketers’ most saught-after holy grail over the past year.
A Data Clean Room enables granularity to a degree that up until recently was simply not possible. It collects data from authorized 3rd-party sources that are ingested and segmented into a range of behavioral, demographic, and location buckets, and then leveraged to enhance your internal database for more granular data enrichment and analysis.
The beauty of it all — is that rather than requiring users’ personal data to be shared in order to conduct analysis, a Data Clean Room enables multiple data sources to be virtually connected through anonymized cohorts.
This enables marketers to measure the intersection that exists between their target audience and the various media audiences. Finally, they’re able to understand the optimal route to reach their audience, plan more effective campaigns, and unlock omni-channel measurement.
How can granular audience insights supercharge your marketing efforts? Glad you asked:
Honing audience targeting
Segmenting your audiences based on fine-tuned data such as consumer behavior and shopping habits — can have a dramatic effect on your campaign strategy.
Let’s say your brand has recently solidified a new partnership with another brand that shares an audience overlap with yours. Using Clean Room-enabled audience insights, you can identify overlay points and shared characteristics that can then be leveraged into further strategic analysis.
Crafting tailored content and curating engagements
When you understand the interests of each market segment, you can create more relevant content, promotional recommendations, and new ad formats specifically tailored to those interests.
Refining your messaging, formats, ad types and channels to be able to address each segment individually, speak their unique language and address their specific pain points — is so much easier when utilizing a Data Clean Room environment.
Granular segmentation use case
Say you own an eCommerce brand and your 1st-party data includes customer attributes and associated product stock keeping units (SKUs). You’d like to run a campaign targeting a prospective audience that exhibits similar attributes, and then follow up with a relevant remarketing campaign based on shopping history and frequency.
First, create your target segments. Then, upload the relevant data sets into a Data Clean Room, where your team can work with ad partners to cross analyze your 1st-party data with their 3rd-party data. This results in aggregated, actionable outputs that can help you craft targeted campaigns — without jeopardizing your users’ privacy.
3 – Optimizing reach and frequency measurement
Once you have PII-level impression data from partnered ad networks, you can understand exactly what ads are being served to which customers and how often, which — in turn — can be used to deduplicate campaign reach and frequency, minimize ad fatigue, and improve your media planning.
Data clean rooms can also validate the assumption you’re reaching out to the right audience, which will help you tweak and hone your segmentation criteria. And, Data Clean Rooms allow you to optimize your customer journey, engaging users based on where they are in the funnel and how they interact with your ad.
4 – Incrementality measurement
Impression data from publishers, audiences, 1st-party response and conversion data can all be tied together at the user level to help you understand the incremental impact of your marketing efforts.
Think about the ability to compare between your test and mediating groups through A/B testing, or more importantly — between your exposed and unexposed groups. Pretty powerful stuff, huh?
5 – Showcasing user quality to prospective advertisers
Publishers can inject user level data into a Clean Room’s secure environment and allow advertisers to gauge customer overlap — and even users’ quality — based on various characteristics.
On the flip side, advertisers can build an audience and then test it against publisher X to assess results. It’s an ideal sandbox for both publishers and advertisers to weigh in and demonstrate the value of their acquired users.
6 – Forging 1st-party data partnerships
On the strategic side of things, two entities can agree to join and match datasets in a safeguarded and permission-only environment, cultivating new partnerships within the media ecosystem.
This secured cross-analysis can also help propel product development, and enable marketers to improve their strategic planning.
7 – Training, inference, and propensity scoring
Lastly, a Data Clean Room environment enables you to regain access to restricted granular user level data — required to successfully run training and inference models, and even propensity models, by which you can get an estimate of the likelihood that a customer will perform a specific action.