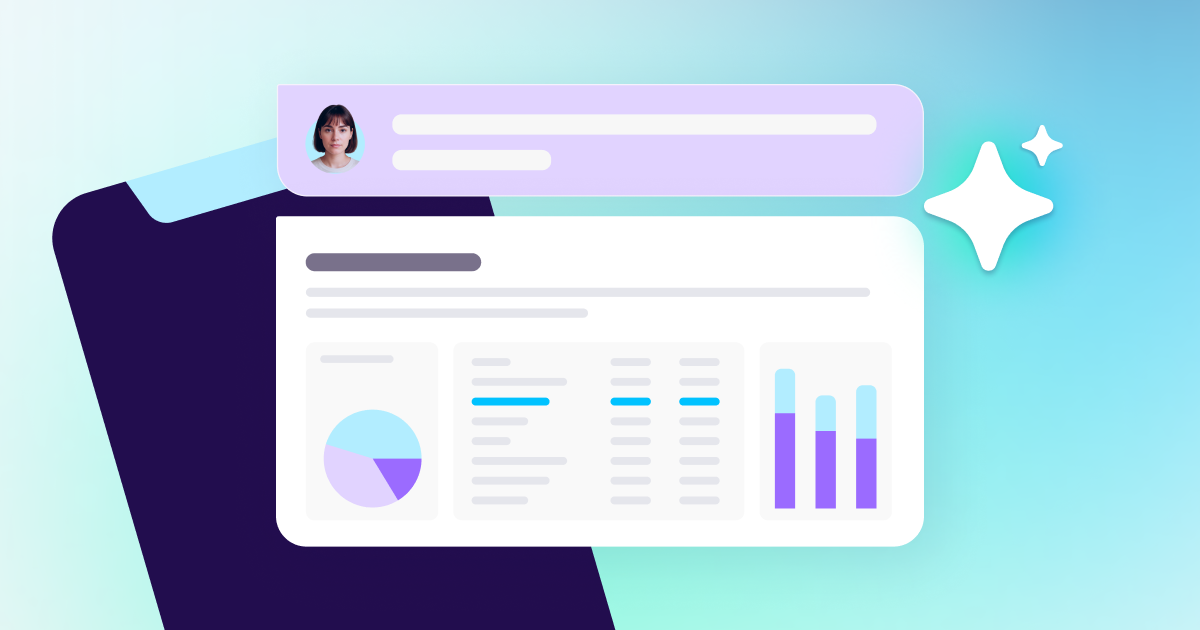
Why media mix models are the answer to unlocking measurement in a privacy-centric world
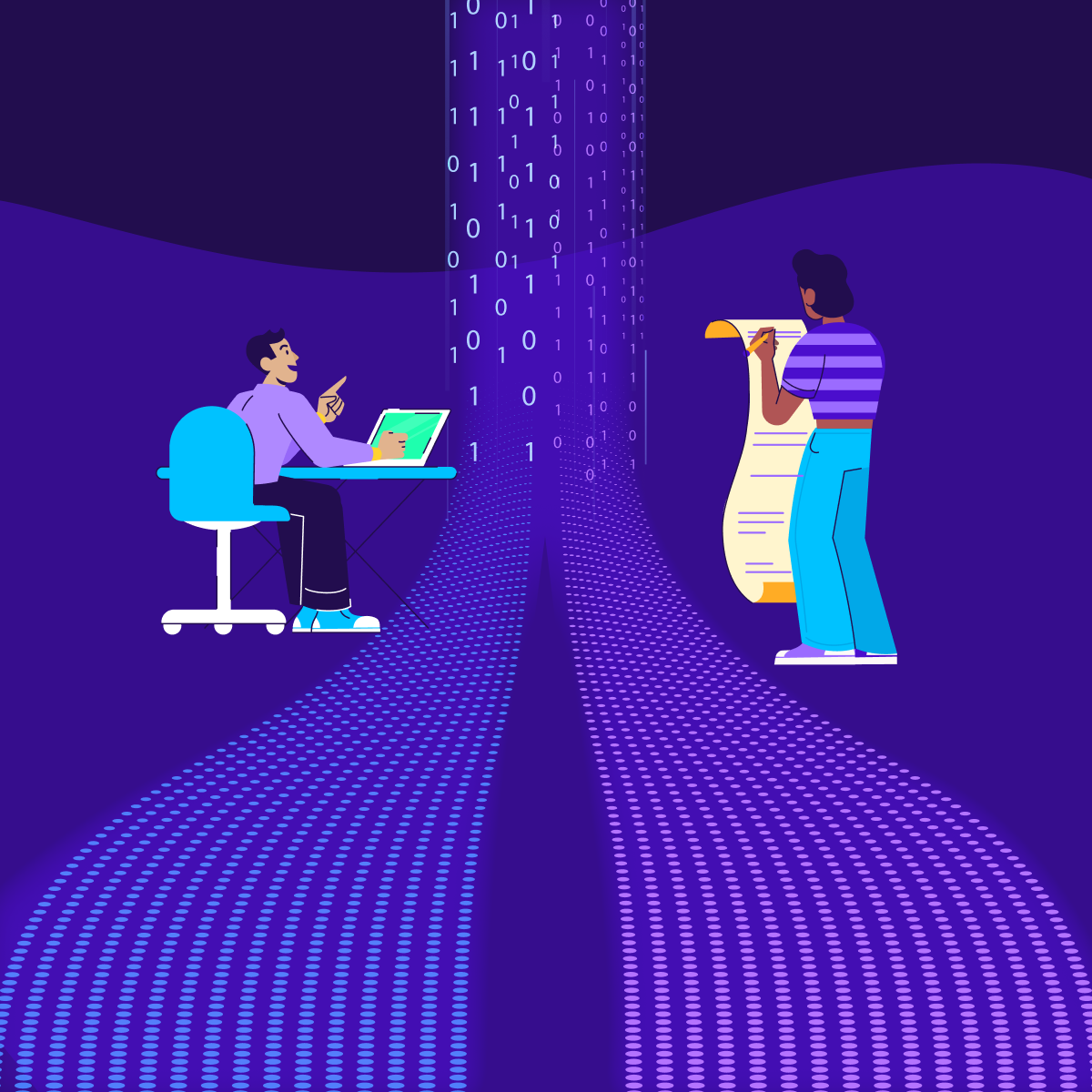
As a performance marketer, you regularly have to answer questions such as “What is the optimal media spend for each marketing channel to achieve your target KPIs?”
Could you answer this right away with absolute certainty? If not, perhaps a media mix model (MMM) could be worth investigating.
This might sound obvious, but in an increasingly complex and privacy-centric marketing landscape, media measurement and the subsequent understanding of return on investment (ROI) are becoming more vital than ever.
Media mix modeling is one of several methods available to help you measure the effectiveness of your marketing spend. Read on to find out how this statistical method can help you measure, optimize, and predict the impact of your marketing efforts on ROI.
What are media mix models?
Media mix modeling (MMM, sometimes known as marketing mix modeling or econometrics) is a statistical method that measures how successful marketing campaigns have been. Used properly, these models can become an engine for all your business decision-making.
A significant advantage of MMMs is that they don’t only isolate the media’s impact, but also consider other factors such as product changes, seasonality, competitor activity, economic conditions, and so on. Because they aggregate data from a wide variety of sources, rather than relying on user-level data, MMMs can provide measurement solutions in today’s privacy-centric landscape.
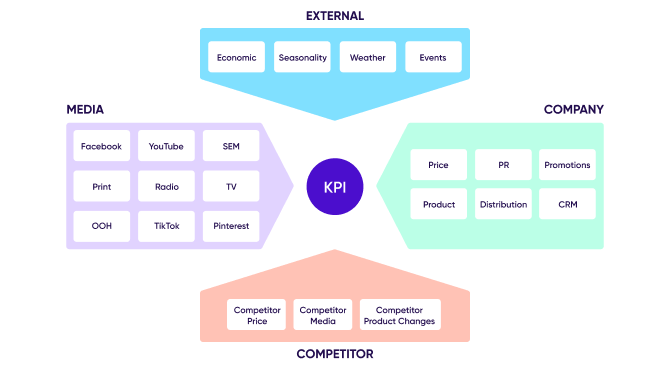
MMMs gained popularity in the late 80s and early 90s, offering marketers a way to prove the impact of channels like TV, radio, and press on a brand’s business outcomes. But, while the original models were time-consuming and costly, requiring a team of experienced analysts, the more laborious parts of the process can now be automated thanks to improved technology and open-source MMM frameworks. This is good news for performance marketers.
Today, MMM has jumped up the priority list for many advertisers seeking to understand the true impact of their marketing campaigns — and, therefore, their marketing ROI. With Apple’s ATT framework, its SKAdNetwork, and Google’s much-anticipated Privacy Sandbox severely limiting access to user-level data, performance marketers have learned to stay ahead by combining MMM with attribution and incrementality testing.
Why invest in an MMM?
Building an MMM is not a simple task. It requires experienced analysts or data scientists, cross-team collaboration, typically over 12 months of historical data from all sources, and dedicated budgets.
However, the investment will pay off as your model becomes the engine of your budget-setting and optimization process.
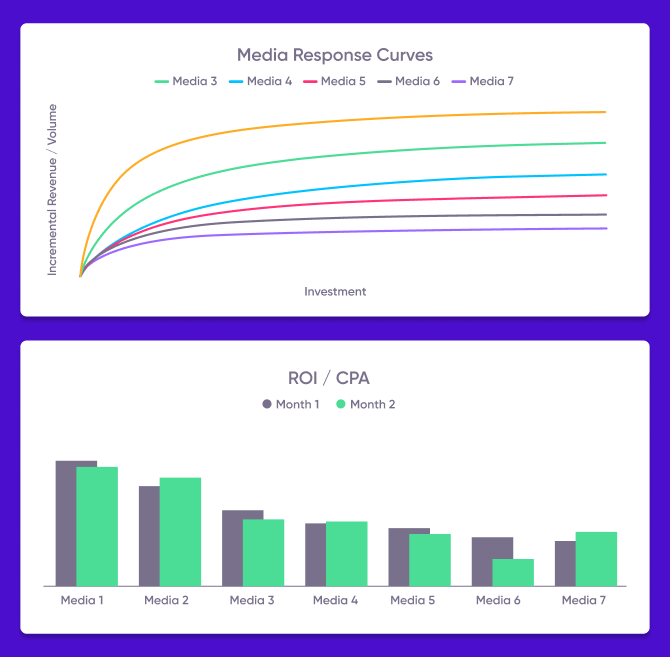
Here are some of the questions an MMM can help you answer:
- Where should we allocate ad spend?
- What’s the ROI of each marketing channel?
- Which media campaigns drove the greatest return?
- What’s our optimal media mix?
- When is the optimal time of year to invest?
- What should our budget be to achieve our annual targets?
- Can we identify any areas of diminishing returns in our marketing investments to optimize spending efficiently?
- What’s the impact of external factors, such as seasonality, economic trends, and competitor activities, on our business?
This is not an exhaustive list of questions by any means. The key thing to note is that MMM is a powerful tool for both tactical media activations and longer-term strategic planning. The more time you spend using an MMM, the greater the impact you’ll see on your business. Typically, brands can see a 10-20% improvement in ROI after one MMM iteration.
What’s the difference between MMM and attribution?
While both MMM and attribution aim to quantify the impact of different marketing activities, they do so through very different lenses, ultimately using different data and methodologies.
MMMs avoid privacy issues by using aggregated data and advanced statistical techniques to analyze historical data, quantifying the impact marketing, media, and other external factors have on your KPIs. You can use the insights you gather to optimize your future marketing investments.
Attribution, on the other hand, operates on more granular, user-level data. It traces the journey of individual customers across different touchpoints to determine which marketing activities were responsible for a conversion. Attribution focuses on the user’s path to purchase within a shorter timeframe and doesn’t account for external factors. However, its superpower is its granular insight – for example, it can answer questions like, “What is my most efficient placement?”
Combining approaches for bigger impact
Bear in mind that, in our privacy-first world, these two measurement approaches complement each other. So, rather than asking, “Which method is better/more accurate?”, you should be asking, “How can I use both together to maximize my marketing returns?”
By combining MMM and attribution, you can optimize media for both the short and long term. What’s more, you’re well-positioned for the cookieless future. You could also add in geo or matched marketing testing: an incremental testing approach that can be used to verify the results and recommendations you see through MMM and attribution.
How to get started building an MMM
When you’re ready to build an MMM for your business, it’s crucial to approach it systematically. Here are some steps to guide you in your development:
- Define objectives: Be clear about the goals or KPIs you want to measure. These could include sales, app downloads, traffic, conversion metrics, and more.
- Define a learning agenda: List the key questions you initially expect your MMM to answer. While it’s tempting to create an exhaustive list of questions, it’s better to focus on a core group of about six. Then, refine and delve deeper into subsequent MMM updates.
- Gather data points: You need a range of data from multiple sources, typically covering 12-18 months. This could include historical sales data, media budgets, reach, frequency and engagement metrics, competitor data, and so on.
- Prepare your data: Preparing your data for analysis involves data cleaning, normalization, and dealing with missing values.
- Develop your model: Develop a statistical model using techniques such as regression analysis and/or machine learning (using open-source frameworks, as detailed below) to isolate the key drivers of your business KPIs.
- Implement the model: Use the model to guide marketing decisions, iteratively refining it as more data becomes available.
Validate your model: Regularly run geo/matched market tests to validate the magnitude of the impact that the MMM is suggesting for your media channels.
What platforms/software/people do I need to build an MMM?
The first step on any MMM journey is answering the question, “Who is going to build your model?”. Typically, it comes down to three choices:
- Your in-house analytics or data science team
- An MMP or analytics consultancy
- Your media agency
Each option has its pros and cons, which we won’t go into here. However, a word of caution about in-housing your MMMs: while this can be viable for a small-scale proof of concept, it’s easy to underestimate the complexity of the task. This can lead to models that, while statistically robust and satisfying the intellectual curiosity of your analyst or data scientist, don’t provide any commercial value.
This is one of the most common reasons why MMM projects get abandoned in the first place. So, it’s best to look for experienced partners when you’re starting out. Over time, as your program proves its success, you can begin to consider in-housing.
If you choose the second or third option, all you’ll need is your historical data. Most MMM practitioners will request at least 12 to 18 months of historical data across your business metrics, media spend, impressions, clicks, installs, and so on. If you don’t have this much data, then start collecting it now. Models can be built on 12 months of data, but typically these will come with some significant caveats and potential issues.
Stakeholder buy-in is key to successfully implementing an MMM. If your brand has relied heavily on attribution, then the results you see through an MMM may cause discussion.
However, as outlined earlier, MMM and attribution measure two different things: MMM measures incremental impact, while attribution assigns value to marketing channels that appeared in a user journey. Once your key stakeholders understand this distinction, actioning the recommendations provided by your MMM becomes much easier.
Open-source options
If you do want to run a small-scale proof of concept in house, it’s worth checking out either Google’s or Meta’s open-source MMM initiatives:
- Google’s LightweightMMM uses the Python programming language and a Bayesian approach to MMM modeling.
- Meta’s Project Robyn uses the R programming language and various machine-learning techniques to provide a semi-automated approach to MMM modeling.
Remember that these are simply measurement frameworks: the data and results you derive have nothing to do with Google or Meta. It’s also worth noting that Google’s LightweightMMM is not an official Google product, unlike Meta’s Robyn.
While each tool has its pros and cons, you don’t need to get bogged down in the details. The key question is, which programming language are your data scientists most comfortable with? If it’s R, use Project Robyn. If it’s Python, use LightweightMMM.
Key takeaways
As the landscape of personal data tracking evolves, advertisers must stay ahead of the curve to ensure a comprehensive understanding of campaign performance and ROI.
While building a media mix model is not a simple task, the insights and recommendations it provides make the investment worthwhile. MMMs provide answers to some of the toughest questions businesses and marketers face, notably offering guidance on where to invest your marketing budget to maximize its impact and return.
Don’t delay — thanks to open-source initiatives like Meta’s Project Robyn and Google’s LightweightMMM, the barrier to creating your first MMM is lower than ever. The sooner you embark on building your models, the sooner you’ll start to see their impact.