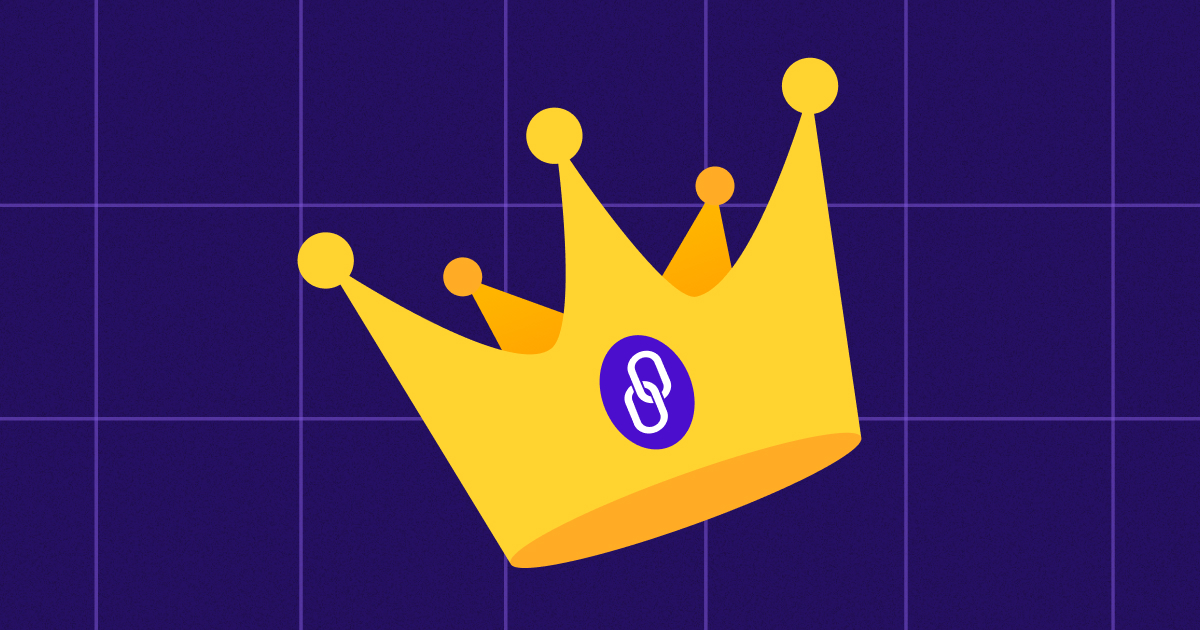
Why contextual targeting in mobile is making a comeback in the privacy age
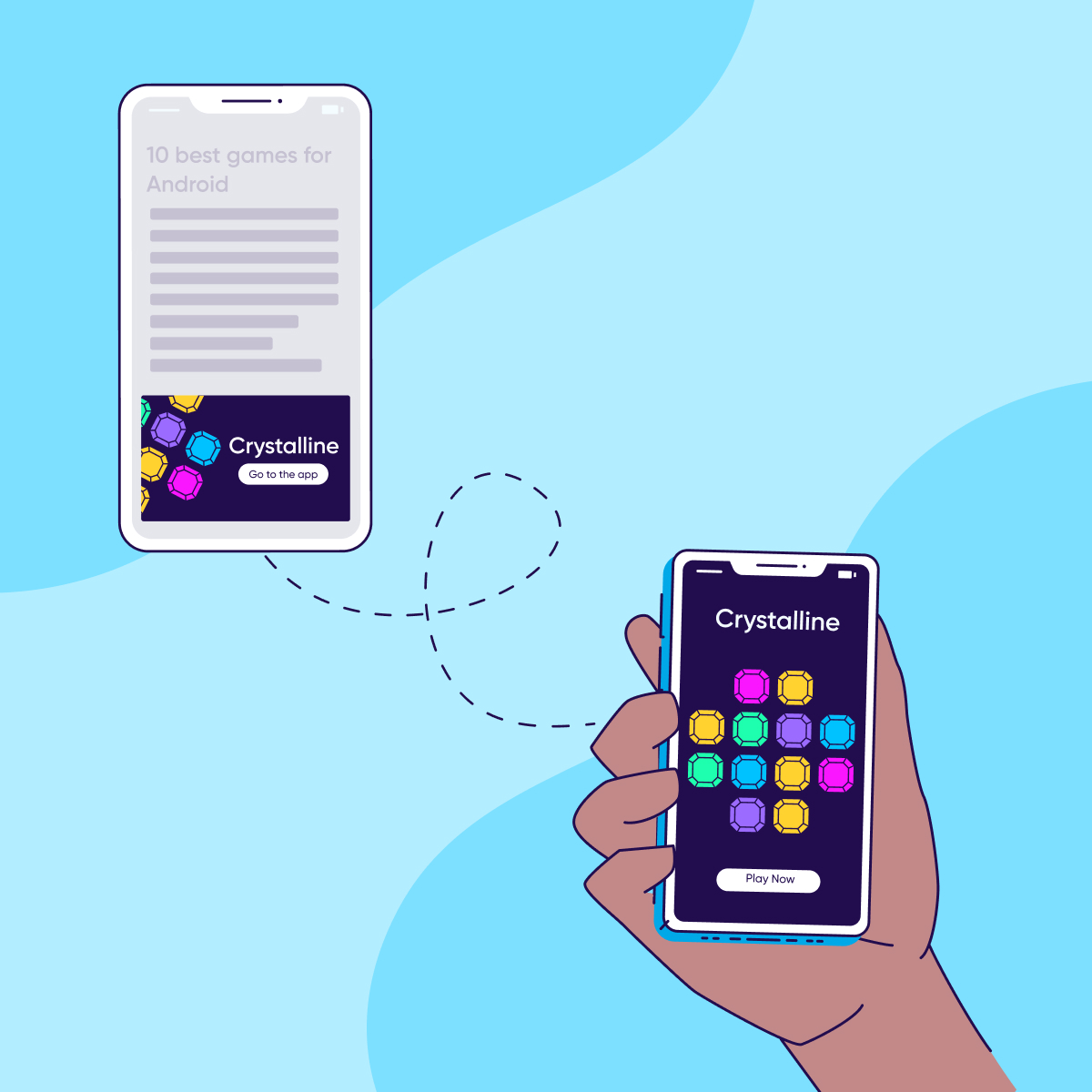
The entire industry is in the midst of grappling with the consequences of Apple’s iOS 14.5+ rollout, Apple’s new ATT privacy framework, and their accompanying attribution solution, SKAdNetwork. At this point, few of us are strangers to the challenges of such a radical and sudden shift.
We will all continue to learn about what works for the ecosystem as iOS 14.5+ and SKAdNetwork proliferate, but one of the clear early conclusions is the importance of relevant, detailed, and scalable contextual data.
While the competition for identifiable users has arguably driven up acquisition cost, the strategic value of alternative targeting approaches, beyond user IDs, presents a great opportunity for increasing the return on your investment.
What is “contextual”?
Taking a step back to define “contextual data” helps hone in on the kinds of data sources and types that can be measured to drive performance.
Contextual ad serving data can refer to any metadata for an ad request that tells us something about the viewing environment where that ad is being served. This can be as broad as the platform, e.g. iOS versus Android, or as granular as the pairing of game features or visual style in the ad content with the sub-genre of the game in which the ad is shown.
Outside of gaming, more detailed contextual data can match with brand personas in the way that interest-based audiences commonly do today. For example, user interest in cheerful, colorful, and optimistic brands could be matched with mood data to more successfully market games or brands.
With contextual data, we are attempting to infer as much about the viewing audience as the environment can tell us. The richer the data, the more we are able to predict the performance of an ad and its potential appeal to the audience.
In the past, we relied on vast stores of user data to predict audience interest and engagement. Now that both user and device-level data are far more limited, we need deeper and richer data about the viewing environment.
Understanding the viewing environment
Contextual targeting is not new, and there are thankfully many options for marketers to pursue in an effort to add tools. But it has become imperative that we do this on both sides of the ad request to capture context cues for the entire viewing environment, i.e., for the publisher and also for the ad itself.
The implication is that advertisers should pursue matching strategies to deliver creatives with similar context cues within app environments that have the same attributes, down to a high resolution of detail.
Ultimately, advertisers need datasets that go beyond basic genres and performance ranking, and into sub-genres, game features, and other attributes that are signifiers of interest and that allow advertisers to scale their creatives.
This combination gives advertisers a unique set of contextual data cues across the entire viewing environment that will help them perform the best.
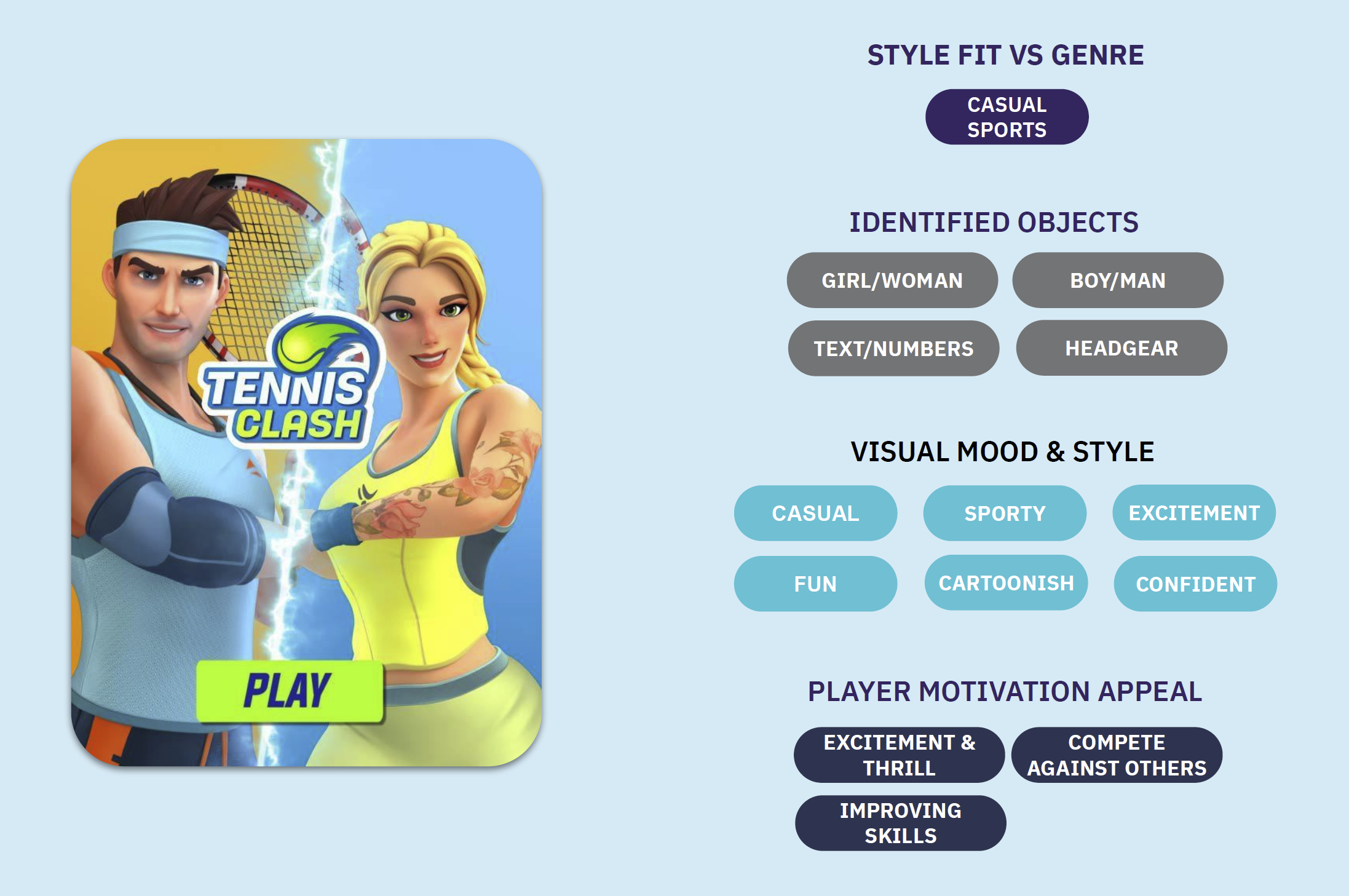
Applying contextual data in practice
One of the key challenges that scalable contextual data can help marketers solve is the difficulty in manually managing targeted app lists, metadata, and matching them in a systematic way to properly execute campaign objectives. This is compounded by the challenge of limited user data due to Apple’s recent privacy rules and the high variability in Return on Investment (ROI) when predictive performance data is scarce.
It is critical for marketers to have a solution and source of data that relieves them of cumbersome manual spreadsheets and frees up the advertising (or UA) team to focus on finding performance levers and optimizing for ROI.
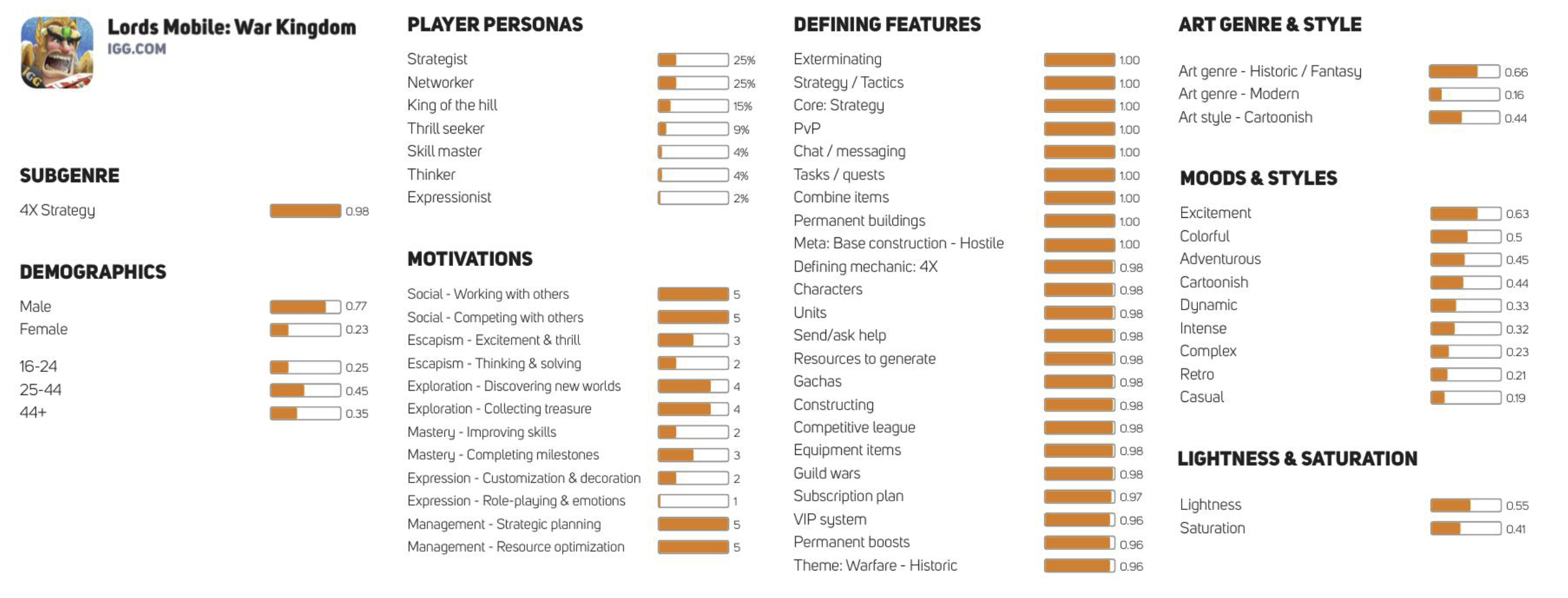
Marketers can design whitelists that scale by finding and leveraging contextual datasets that describe both sides of the ad environment in the same terms. The challenge, of course, is finding solutions that can bring solid source data and technology to apply to potentially thousands of creatives and campaigns.
Among the questions to ask about your contextual targeting approach is whether or not you believe in the data and whether it is rich and differentiated enough for you to form hypotheses that you can test and iterate on.
Thin datasets that stop at genre or app scores can only go so far. Also remember that you will need to index your own creatives and apply a consistent methodology so that you can be confident in your results, all without creating too much work for yourself.
Criteria for success
While rich contextual datasets are a potential source of insights and drivers of performance in a more privacy-centered world, we need contextual data to meet three criteria in order to drive things forward:
- The data needs to be actionable
- Both the data and informed actions must be scalable
- We must be able to measure the outcomes of our contextual data-driven strategies.
“Actionable data” means that the data must be robust enough to draw conclusions from and act on in terms of campaign execution.
For example, a one-sided dataset about app popularity or performance is not enough to support sophisticated strategies. Having a set of genres and sub-genres, information on game elements or features, or characters, moods, and styles that draw a clear picture of the particular audience on both sides of the serving environment will enable you to develop and test robust hypotheses with repeatable results.
But once a more robust and detailed dataset has been identified, the second criterion becomes essential: the data must scale.
This has always been a critical test for targeting data to pass, the best 1st-party audience data is only as good as its ability to deliver results on a large scale, and hitting a cap on reach is commonly the first hurdle.
For contextual data, seeking a unique intersection of viewing and environment, the scale will be a challenge.
Finally, and somewhat obviously, the results must be measurable. Experiments must be structured and run in such a way that lift tied to hypotheses can be measured and verified.
As always, isolating the signals of contextual targeting and matching will be a challenge within the noise of a live campaign, and your campaign management for contextual will likely be as robust as other types of targeting.
Key takeaways
As privacy initiatives ramp up over the coming year, and the industry at large searches for new performance advantages, expect rich contextual datasets to become more valuable and applied with more rigor.
Advertisers need to look for truly scalable solutions to differentiate themselves and drive outsized performance.
UA managers should be asking themselves whether they’ve investigated the best available sources of non-user level data that scales, and whether they can execute on campaign strategies that not only effectively use the data, but also drive performance.