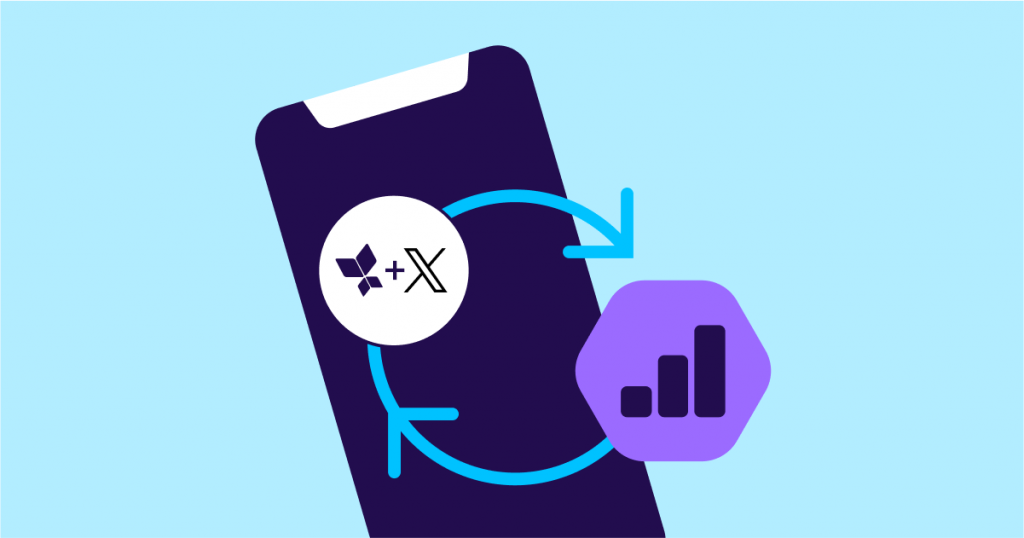
4 min read
Stepping into clarity: introducing complete iOS measurement on X Ads
Measure all iOS conversions with X Ads’ Advanced SRN. Get privacy-safe insights and drive campaign results.