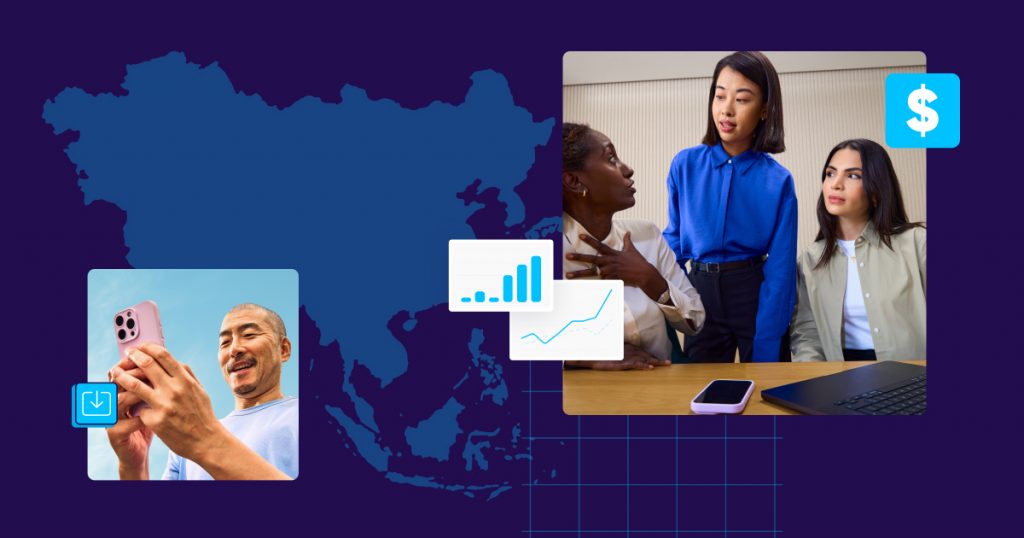
Introduction
This will shock no one, but given our justified obsession with data, this drum needs to be hit louder — marketing performance is based first and foremost on accurate measurement, and a marketer’s quality of measurement is the very key to their success.
When dealing with the opposite, inaccurate data can very easily lead to the wrong decisions, the wrong actions, and consequently cost millions of hard-earned dollars. For reasons we’ll list later on, advertisers can no longer consume data from a single source, or try to piece together the truth from several data silos.
You can’t really get a clear full picture of your campaign performance when different attribution sources report the same install and create duplicates, right?
You can’t plan and execute a comprehensive and effective campaign without knowing how to deal with restricted data and extremely limited access to user-level data, can you?
You can’t really drive growth and business success when your ROI data is contaminated with fraudulent activity and potentially mis-attribution, correct?
So why in the name of all Excels are you basing off your entire performance measurement on skewed, duplicated, missing, or messy data?
The truth is, the road to full data integrity begins and ends with simple awareness. Know what you don’t know, and your path will pave itself.
To help you fight off nasty old data inaccuracies, we’ve put the most painful and common data inaccuracies into 6 buckets, and unpacked some of the more complex concepts in mobile measurement into easily digestible nuggets — that every marketer can turn into action items.
Let the healing begin.
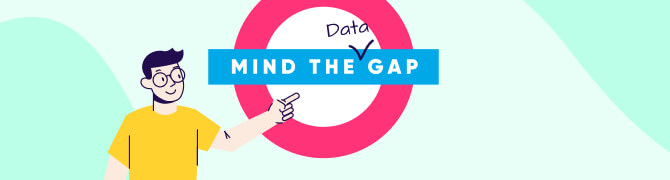
Mind the gap – What you need to know about data discrepancies
What is data discrepancy anyway?
From a definition perspective, data discrepancy is lack of compatibility or similarity between two or more facts.
More specifically in the mobile advertising ecosystem, data discrepancy translates into lack of compatibility or similarity of measured data — such as impressions, clicks, installs, engagements, conversions, in-app events, and KPIs between two or more platforms.
Now let’s pour this theory into practice using these very real real-world examples from the realm of mobile attribution:
MMPs & SRNs
Accurate data helps marketers make the right decisions and improve their ROI. That we all know. But given the vast scope of gaps, silos, and mismeasurement that can occur along the way, ensuring accurate data is often easier said than done.
So, let’s get down to business and review a few common data discrepancies that can occur between mobile measurement partners (MMPs) and some of the largest self-reporting networks (SRNs), including Google, Meta, Apple, Twitter, and Snap.
Word of caution, SRNs change their attribution and measurement practices constantly, so it wouldn’t surprise anyone if by the time this guide is published — some of the following will change once again. Always consult with your MMP to ensure you’re on top of the most recent guidelines.
#1 – Last touch attribution
Users engage with ads across multiple media sources. In the case where the SRN was one of these sources, an attribution will be claimed for an install if a user viewed or clicked on the SRN’s ad prior to the install.
When Meta claims an install attribution credit, for example, it reports it via its Ads Manager if it took place within 7 days from the click that led to the install.
That said, it’s very possible for a person to engage with an ad delivered via an SRN, and later engage with an ad on another media source before installing the app.
In this case, the SRN would claim attribution for the engagement, but will not be awarded attribution credit by the MMP, which is a frequent reason for discrepancies for advertisers that advertise across multiple channels.
Keep in mind that an SRN will usually charge for impressions regardless of attribution. But when that happens, your MMP will reflect the actual attribution, which will allow you to understand the true performance of your marketing efforts, and allocate your budget more effectively.
#2 – Different attribution lookback windows
The default attribution lookback window for app installs on most SRNs and MMPs is usually around 7 days clickthrough and one day view-through. On both platforms, advertisers are able to reconfigure the attribution lookback window to their desired setting.
If, however, the attribution lookback windows for the SRN and MMP are different, that could lead to reporting discrepancies as well.
For example, let’s say the advertiser reconfigured the clickthrough window for Meta Ads on AppsFlyer to 7 days. In this case, Meta will attribute one install to the Meta campaign, and AppsFlyer will report one organic install.
#3 – In-app events attribution
Most SRNs attribute in-app events up to 28 days following the preceding click or view (for Android and iOS consenting users). The MMP usually attributes in-app events to the SRN during the 180 days following the install.
Because of that, when analyzing campaigns over a period longer than 28 days — the MMP might report more in-app events than the SRN. In other words, the discrepancy will be in the attributed claims, and the number of LTVs and in-app events associated with the campaigns.
On a side note, some SRNs — like Meta — don’t claim attribution for view-through engagements for remarketing, which means these views will be considered by SRNs but not by MMPs.
#4 – View-through conversion reporting
As you probably know, TikTok has recently started to partially restrict device-level for view-through, and Meta no longer provides advertisers with device-level data.
While all Meta-attributed installs are accurately attributed to Meta on the MMP’s aggregate reports, view-through installs are attributed to a “restricted” media source on device-level reports.
#5 – Engagement time vs event time
Most SRNs report installs and in-app events according to last-click or last-view time, whereas most MMPs report them according to the time of the first app launch or in-app event conversion time.
#6 – Time zone differences
SRNs and MMPs may have different time zone settings on their platforms, which could affect reporting. When comparing reports over short time periods of time (hourly or daily), bear in mind that these discrepancies could be more pronounced.
#7 – Misconfigurations
Sometimes things are just not implemented correctly, which can also lead to unexpected discrepancies.
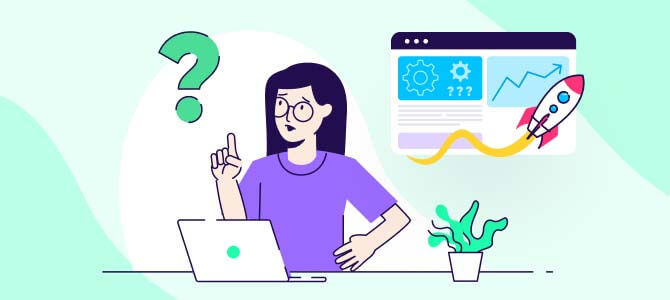
MMPs & app stores
What about the leading causes for attribution discrepancies between MMPs and app stores, you say? Glad you asked.
The simple truth is that there are inherent differences in the attribution models used by all players in the mobile attribution ecosystem. And because of these variations, in some instances the app store might record more installs than your MMP, and in others it might be the opposite.
Let’s break it down:
Why is your MMP attributing less?
#1 – Install definitions
App stores record installs after the user downloads and installs the app, whether the user later launches the app or not. Your MMP, on the other hand, records new installs only after the first launch, which triggers its SDK.
So users who did not launch are not counted by your MMP, even if they downloaded and installed the app.
#2 – Install record date
App stores record the date of the download as the day of install, whereas your MMP records the date of the first launch as the day of install.
#3 – Time zones
App stores’ data displays according to the local time zone of the advertiser, which could differ from your MMP’s time zone.
#4 – Android user uninstalls and reinstalls within the defined re-attribution window
In some views, Google Play might show two unique installs for the same user, regardless of the time that elapsed from the first install. On the flip side, other views might show unique users, which will be more closely aligned with your MMP.
As far as your MMP is concerned, inside the re-attribution window (3 months by default) from the original install time, the user is not considered a new one when reinstalling.
#5 – iOS user uninstalls and reinstalls within the defined re-attribution window
iTunes counts a new install every time the user uninstalls and re-installs.
As with Android, your MMP does not consider a user as new when reinstalling, as long as it’s within the re-attribution window (3 months by default) from the original install time.
Why is your MMP attributing more?
#1 – Upgrading an existing app install from a version that did not contain your MMP’s SDK to a version that did
App stores regard this as the same user and not as a new install, whereas your MMP shows the upgrade as an organic install.
#2 – Device ID reset and Limit Ad Tracking fraud
Fraudulent installs made through device ID reset and Ad Tracking are very popular, and while app stores don’t regard these as new installs, your MMP sees them as a new install on a new device.
#3 – Installs coming from 3rd-party Android app stores and pre-installs
Google Play does not record these installs, while your MMP does.
The solution?
This mostly comes down to knowledge and awareness. Once you know what to look out for, you can anticipate where and why these sneaky discrepancies might pop up, you’re much better positioned to mitigate them by tweaking misconfigurations or cross-referencing your raw data.
But mainly, it’s about relying on your MMP to offer you a much-needed single source of truth, so you can pinpoint these discrepancies quickly and be able to make data-driven decisions.
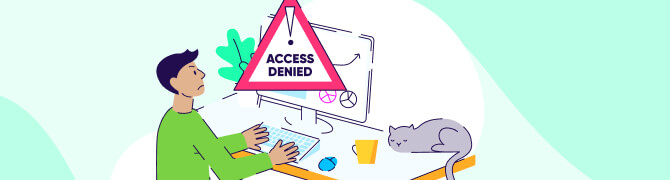
Data in the privacy era – and the dramatic effect it has on your performance measurement
Although restricted data is not the same as data inaccuracies, lacking the knowledge and tools to deal with it does affect your ability to gain a holistic view of your marketing efforts. And when you don’t have the full picture — your decisions are bound to be weaker in potency.
So, let’s assess the unique circumstances that led user-level data to become so elusive, and the practical ways you have to alleviate these recent challenges:
As the industry continues to acclimate to the new privacy era in the wake of Apple’s App Tracking Transparency (ATT) framework, it’s critical that data accuracy and user privacy continue to coexist.
Having 100% certainty in the true results of your marketing efforts is nothing short of mission-critical, which is where a single source of truth (SSoT) for marketing performance comes into the picture. It enables you to optimize your ad spend, grow your business, and offer superior UX to your users without compromising their privacy.
But how do you get there? Let’s start by addressing one of the most challenging hurdles on the path to comprehensive data visibility:
1 – SKAdNetwork – the (not so) new kid on the block
When Apple introduced the iOS 14 updates that would forever change the mobile app ecosystem, every mobile marketer on the face of the planet asked themselves the following question:
Is SKAdNetwork going to be a good method for campaign measurement? (answer: yes and no)
Over time, however, it became evident that the most pressing concern was actually:
How can I funnel all of these siloed data sources into a single source of truth?
The reality of this new reality is that there are multiple realities. With the onset of iOS 14, marketers began receiving data streams from many different sources: aggregated deterministic data from SKAdNetwork, user level data from ATT-consenting users, aggregate data from probabilistic modeling, incrementality-based insights, dedicated APIs for Apple Search Ads, and more.
But which data stream is the actual truth here? How are marketers supposed to deal with all this incoming data and feel confident in the decisions they’re making?
Luckily, a solution for this critical problem does exist. Before we get into it, let’s take a deeper look at the issue at hand.
I got 99 problems but a SSOT ain’t one
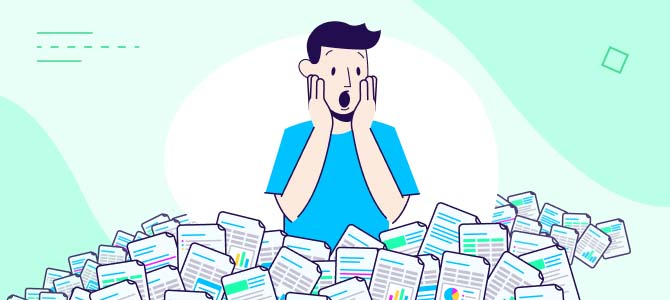
Although SKAdNetwork offers two significant advantages over other measurement methods — namely it’s deterministic and covers all users — it also poses a few major disadvantages:
LTV measurement in SKAN is a major challenge for advertisers. It only offers limited post-install data, it doesn’t cover all flows (like mobile web), postbacks are delayed, and there are potential fraud loopholes — to name a few.
To be able to mitigate this limitation, some marketers turn to ID matching, aggregated advanced privacy, and probabilistic modeling — that come with their own set of upsides and drawbacks.
Choosing the preferred model based on specific needs won’t work either, because SKAdNetwork data is anonymized, meaning no entity can determine if the same conversions were attributed by other models or not. Potentially, each install could be attributed by SKAdNetwork alone, by other attribution modes, by both or by none.
This is the very essence of SKAN: anonymization. So by design, it prevents reverse-engineering for user-level matching.
The reality is that multiple data sources, APIs and dashboards make it nearly impossible to reach accurate and actionable insights, and marketers are reduced to guesswork when it comes to analyzing their campaign performance.
Aaaaand problem solved!
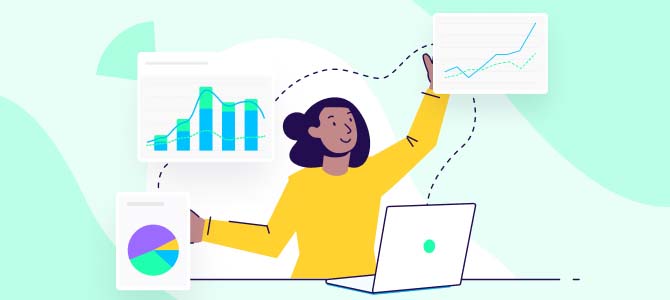
The only real solution to this extremely frustrating situation is a consolidated dashboard or API where the data is combined, deduplicated, and unified, all while preserving user privacy and aligning with Apple’s policies.
Because SKAN has its limitations, many of them can be overcome by innovating on top of the protocol’s conversion values, which are the only way for iOS advertisers to measure user LTV in SKAN campaigns.
By properly mapping out 64 possible values, advertisers can measure post-install revenue, activity, and retention (though only up to 24-72 hours in the vast majority of cases).
So, the answer to a siloed, disparate reality that stems from limited data — is leveraging conversion values to create a unified reality, or a single source of truth across the various attribution sources, where each value can be maximized and fully accounted for in the mapping.
While conversion values do limit the marketer’s ability to measure LTV (both in time and range), they do offer up the ability to unify reported data in aggregate reports.
2 – Data Clean Rooms – and how they enable marketers to make solid decisions without direct access to user-level data
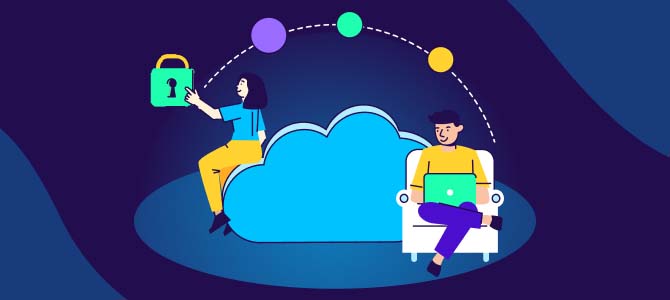
Data clean rooms allow marketers to harness the power of combined data sets while adhering to privacy regulations. In essence, they’re a tool that allows marketers to leverage user-level data insights without actually being exposed to it.
Within the data clean room environment, personally identifiable information (PII) or user-level attribution data is not visible to any of the involved contributors, which makes it impossible for them to single out users with unique identifiers.
PII and user-level data are processed so that it can be made available for a variety of measurement purposes, producing anonymized data that can then be cross-referenced and combined with data from different sources.
In most cases, the only outputs from the data clean room are aggregate level insights, e.g. users (plural!) who have performed action X should be offered Y. That being said, it’s important to bear in mind that user-level output can take place given the full consent of all involved parties.
Why should you consider a data clean room?
The key ingredient that makes data clean rooms a highly credible platform is the fact that access, availability, and usage of data are agreed upon by all data clean room parties, while data governance is enforced by the trusted data clean room provider.
This framework ensures that one party can’t access the other’s data, which upholds the ground rule stating that individual or user level data can’t be shared between different companies without consent.
Let’s say a brand wants to share insights with Macy’s. To facilitate that, each party needs to place its user-level data into a data clean room — to see what the other already knows about audiences they have in common. For example, reach and frequency, audience overlap, cross platform planning and distribution, purchasing behavior, and demographics.
Data clean rooms can also be used as an intermediary tool for measuring campaign performance. Instead of guesstimating audience insights, brands can actually look under Amazon or Google’s 1st-party data hood, all while being completely privacy-abiding.
In return, advertisers can get an aggregated output without individual identifiers, including segmentation and look-alike audiences, which can then be shared with a publisher, an ad network, or a DSP to inform a campaign.
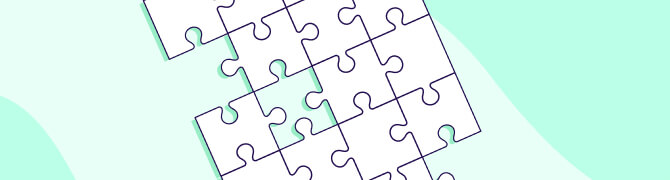
Incompleteness of data – and how a holistic view of touchpoints and related costs can impact your bottom line
Unsurprisingly, the market today is facing a serious challenge around the standardization of data.
Without being able to report properly and holistically on their KPIs, marketers are making serious budget and optimization decisions based on incorrect or partial information, which is easily any marketers’ worst nightmare.
Cost reporting is a zero-sum game, and working with partial data is equal to not having the data to begin with. How can you assess the true ROI of your campaigns, optimize efficiently and decide how to allocate your budget without it?
Incomplete data is not and should not be an acceptable status quo, especially when it involves your most essential data — cost and revenue.
For the average marketer, extracting, gathering, aggregating, and analyzing the data from every single source is a daunting task, to say the least. Yet every marketer should be able to have accurate and complete cost data side-by-side with their trusted attribution data.
Hey, big spender – the challenges of spend-related data inaccuracies
- A distinction must be made between ROI and true ROI. While working with accurate ROI data is the holy grail for performance marketers, working based off of inaccurate ROI data can completely derail your marketing efforts and even risk your app’s financial stability.
- Brands usually work with anything between 5 to 30 ad networks, and normalizing cost data from so many different sources is a monumental task, largely because of having to juggle so many APIs.
- After data normalization, advertisers need to consolidate their cost and attribution data, which is not only a challenge in its own right, but also leads to limited and inaccurate insights.
- Data changes constantly, especially in the first few days of a campaign’s life, which requires frequent data refresh multiple times a day in order to maintain accuracy.
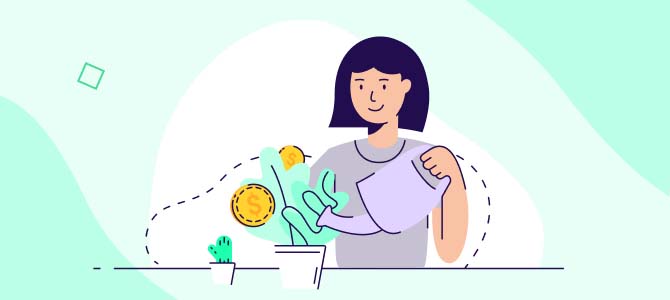
Show me the money! The challenges of revenue-related data inaccuracies
Companies usually employ 3 main revenue streams that need to be maintained and analyzed separately:
- In-app purchases (IAP) – advertisers are quick to fire an event whenever an IAP takes place, but oftentimes forget to take into account refunds or deferred payments — which calls for constant updates using app store data.
- Subscription revenue tracking requires either building a complex product or purchasing an off-the-shelf piece of software, which still leaves advertisers with the painful task of consolidating it with their attribution data.
- In-app advertising (IAA) – requires near real-time freshness and user-level capabilities, which is not easy to maintain. Additionally, measuring ad revenue in-house is very likely to hurt accuracy and consequent decision making.
Luckily, we like challenges!
Solution #1 – Extracting your cost data from any source
As a marketer, you need to extract data from hundreds of sources and choose your preferred method of cost extraction. Since no two sources report data using the same level of granularity and frequency — extracting what you need when you need it — is no walk in the park.
For example, there’s no unified standard for click URL structures, types of dimensions, campaign naming conventions, or cost metrics (Twitter has tweets, Facebook has likes, Snapchat has swipe ups, etc.), which could turn the task of comparing hundreds of networks’ performance and cost side-by-side into a miserably painstaking endeavor.
On top of that, some networks report cost data on the campaign level, while others do so on any structural level, which is why a good cost reporting solution has to be flexible.
To be able to tackle this, be sure to partner with a measurement provider that can extract the data you need using various methods — such as click, API or ad spend ingestion capabilities, which can help you reprocess incomplete or incorrect data — while having the flexibility to ingest additional sources of data (e.g. influencer channels, email marketing, and push notifications).
Solution #2 – Owning the data management flow
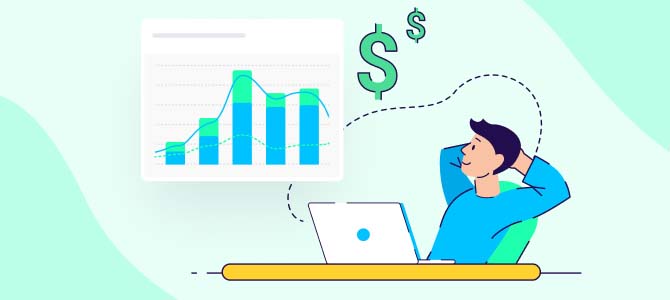
Relying on other networks, partners or channels to pass data regularly and in a timely manner so advertisers can make critical decisions — is nearly impossible today.
And if you collaborate with other teams on identifying gaps, lags, or inconsistencies, and correct the data on the go — just think how much time is lost on communicating these gaps before being able to actually fix them.
Take for example a scenario where a network has issued a rebate, and as a result — an advertiser wants to reflect the true cost for that channel in their ROI calculation for a given period of time. Having the ability to update and reflect the true numbers in the UI quickly and easily, could very much be a game changer.
That’s why allowing advertisers to own every element of their data pass, no matter the source or method of extraction — could be such a major pain relief.
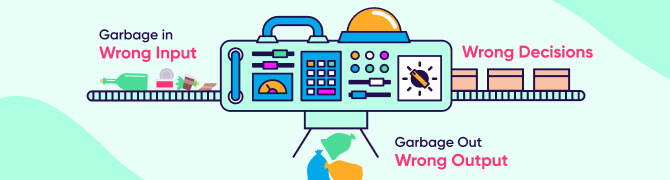
Garbage in, garbage out – and the importance of data integrity across your BI systems
In modern marketing, attribution data is the source of every decision. But regardless of how sophisticated your marketing BI and teams are, if their decisions’ input parameters are wrong — that inevitably means their decisions’ outcome will be wrong too.
Fraud and mis-attribution are the two main culprits for skewed data nowadays. And in both cases, great looking measurement leads you to believe you made smart, profitable decisions. What you probably don’t know is that you’re buying your own fraudulent traffic, and doing this over and over again on a growing scale will lead your business to the dreaded bleeding cash cycle.
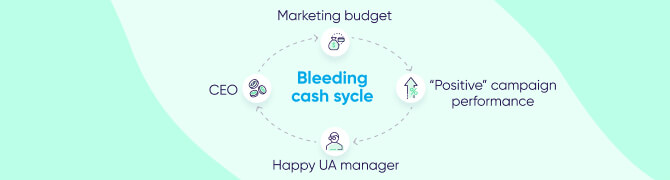
Fraud and the bleeding cash cycle
ROI seems to make some marketers enjoy a false sense of security.
But the thing is, these marketers don’t know that they don’t know their true ROI, and what might seem like positive ROI could be heavily distorted by sophisticated forms of fraud, or false positive attribution caused by loose attribution platforms, leading to millions of dollars in losses.
These marketers spend their precious budget on marketing activities without paying attention to the finer details — like where their installs are coming from, and whether the numbers actually make sense.
Numbers DO lie! Your marketing data is not to be trusted blindly
In most cases, marketers’ work is measured by simple volumes, allowing them to enjoy looking at the upwards facing graphs without paying to think whether their growth was due to their smart investments or something completely different.
Not knowing any better, they end up paying for organic users they shouldn’t pay for, or even worse — paying fraudulent sources that steal credit from other legitimate sources. They’ll then reinvest in the fraudulent sources that generated so-called “positive performance” through bots and fake users, all while bringing in zero value.
In most cases this fake growth trend will look good enough when presented in board meetings to keep everyone nodding with approval, while their money is wasted and fueling the fraud business.
Mobile ad fraud is a $2.1 billion industry with long-term effects on all marketing aspects (the most obvious being direct financial loss), and it’s funded mainly by advertisers who simply allow it to exist by not treating it.
Back in 2021, install-level fraud took up the bulk of all fraudulent activity, and although CPA, remarketing, and in-app purchases have yet to be quantified financially — they still add additional financial exposure.
And once fraud hits your data — it renders it irrelevant and unusable. You’re not looking at actual data, and can’t separate legitimate from fraudulent activity, or rely on it for future optimization. Most likely, It will encourage you to re-invest in bad media sources that either stole credit from users or generated fake ones.
But above all, fraud is simply a huge waste of valuable time and very valuable human resources. To mitigate it, your teams will need to spend endless hours working on reconciliation and trying to make sense of complex fraud and performance reports.
To learn more about mobile fraud prevention, have a look at our State of Mobile Ad Fraud report.
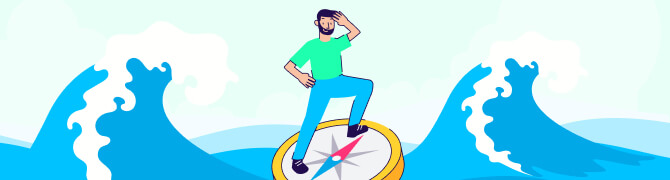
Navigating rough waters – raw data in the fight against data inaccuracies
In the ever-changing world of mobile marketing, raw data can offer marketers a significant edge, save costs, and help them build business resilience far into the future.
What is exactly raw data, you ask? In essence, it’s information like single app installs, in-app events, uninstalls, and device type that can be processed and analyzed, and in the hands of experienced marketers — can even help supercharge brands’ campaign performance.
What are the upsides of using raw data?
Remember the notorious cash bleeding cycle no marketer wants to be caught in? Well, raw data helps you dive deeper and get into the heart of whether your campaign efforts are actually working or not.
Efficiency, baby
Now, more than ever before, marketers need to be efficient and target their efforts at what’s generating the highest ROI. Raw data can help marketers do just that, by optimizing their traffic in real-time, identifying critical metrics, boosting the lifetime value of their marketing efforts, and getting more bang for their buck.
Taking back control
Advertisers can define a specific endpoint for organic data and another for non-organic data, one for in-app events and another for remarketing.
Configuring multiple endpoints allows you to organize your data the way you want it to be organized, reduce the amount of “noise” (irrelevant traffic and events), control inbound data more efficiently, and make it easier for your BI team to process that data.
Leveraging raw data in designing effective campaigns
Raw data reports are filled to the brim with valuable insights. Here are a few common applications:
- Understanding user behavior – With the help of timestamps, raw data can help you better understand user interactions and get more granular visibility into your user journeys.
- Improving UX that drives growth – Once you’ve gauged user behavior using raw data, you can apply your insights into improving user experience.
- Performance-based pricing – Raw data reports provide a clear and accurate picture of ROI, allowing for a performance-based pricing model — where agencies or marketers can be billed upon achieving certain goals.
- User segmentation – You can use cohort analysis or consenting user-level data to create highly efficient user segmentation.
- Identify potential fraud – There are many ways to identify and fight potential fraud in mobile marketing. One of them is looking at your raw data, which will allow you to fish out suspicious patterns — even when dealing with savvy fraudsters that create different identifiers for the same devices.
For example, you could be able to pinpoint how many installs or unique identifiers are coming in from the same IP, pick up on illogical time stamps (e.g. every 1-5 seconds), or the presence of multiple IDFAs for a single IDFV.
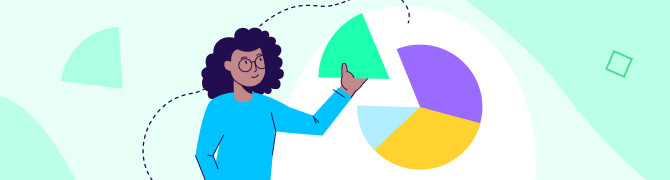
Key takeaways
- Dealing with inaccurate, missing or messy data, can very easily lead to the wrong decisions, the wrong actions, and consequently cost millions of hard-earned dollars.
- There are inherent differences in the attribution models used by all players in the mobile attribution ecosystem. And because of these variations, your MMP and SRNs might record and measure data differently — leading to noticeable data discrepancies.
- In the realm of SKAdNetwork, advertisers can no longer consume data from a single source, or try to piece together the truth from several data silos. A single source of truth (SSOT) for marketing performance can enable you to optimize your ad spend, grow your business, and offer a superior UX to your users without compromising their privacy.
- Some marketers have no idea what their true ROI is, as it could be heavily distorted by sophisticated forms of fraud, or false positive attribution caused by loose attribution platforms, leading to millions and even billions of dollars in losses.
- Mitigating data discrepancies mostly comes down to awareness. Once you know what to look out for, you can anticipate where and why these discrepancies might pop up, you’re much better positioned to mitigate them by tweaking misconfigurations or cross-referencing your raw data.
- Raw data as a way to ensure business resilience – The potential of raw data to draw any number of actionable insights in the battle against data discrepancies is more compelling now than ever before. It gives brands an extra toolset to optimize campaigns far into the future.
- Partnering with a strong MMP can help you mitigate most if not all of these data inaccuracy issues, work out of a much-needed single and holistic source of truth, quickly pinpoint any potholes, and make data-driven decisions.